Co-evolutionary competitive swarm optimizer with three-phase for large-scale complex optimization problem
Information Sciences(2023)
摘要
Practical optimization problems often involve a large number of variables, and solving them in a reasonable amount of time becomes a challenge. Competitive swarm optimizer (CSO) is an efficient variant of particle swarm optimization (PSO) algorithm and has been applied extensively to deal with a variety of practical large-scale optimization problems. In this article, a novel co-evolutionary method with three-phase, namely TPCSO, is developed by incorporating a novel multi-phase cooperative evolutionary technique to enhance the convergence and the search ability of CSO. In the modified CSO, the population is evenly decomposed into two sub-populations, then the update strategy of each sub-population is adjusted by the requirements of the diversity and convergence during the evolution pro-cess. In the first phase, the diversity is paid more attention in order to explore more regions. And in the second phase, the promising area in two sub-populations are exploited by introducing excellent particles of two sub-populations. The third phase focuses on the convergence by learning from the global best solution. Finally, the performance of TPCSO is evaluated and proved by large-scale benchmark functions selected from CEC'2010 and CEC'2013. The experimental and statistical results show that TPCSO can effectively solve these large-scale problems and fast obtain the optimal results with higher accuracy by comparing with several algorithms.(c) 2022 Elsevier Inc. All rights reserved.
更多查看译文
关键词
CSO,Three-phase co-evolutionary strategy,Update strategy,Optimization performance,Large-scale
AI 理解论文
溯源树
样例
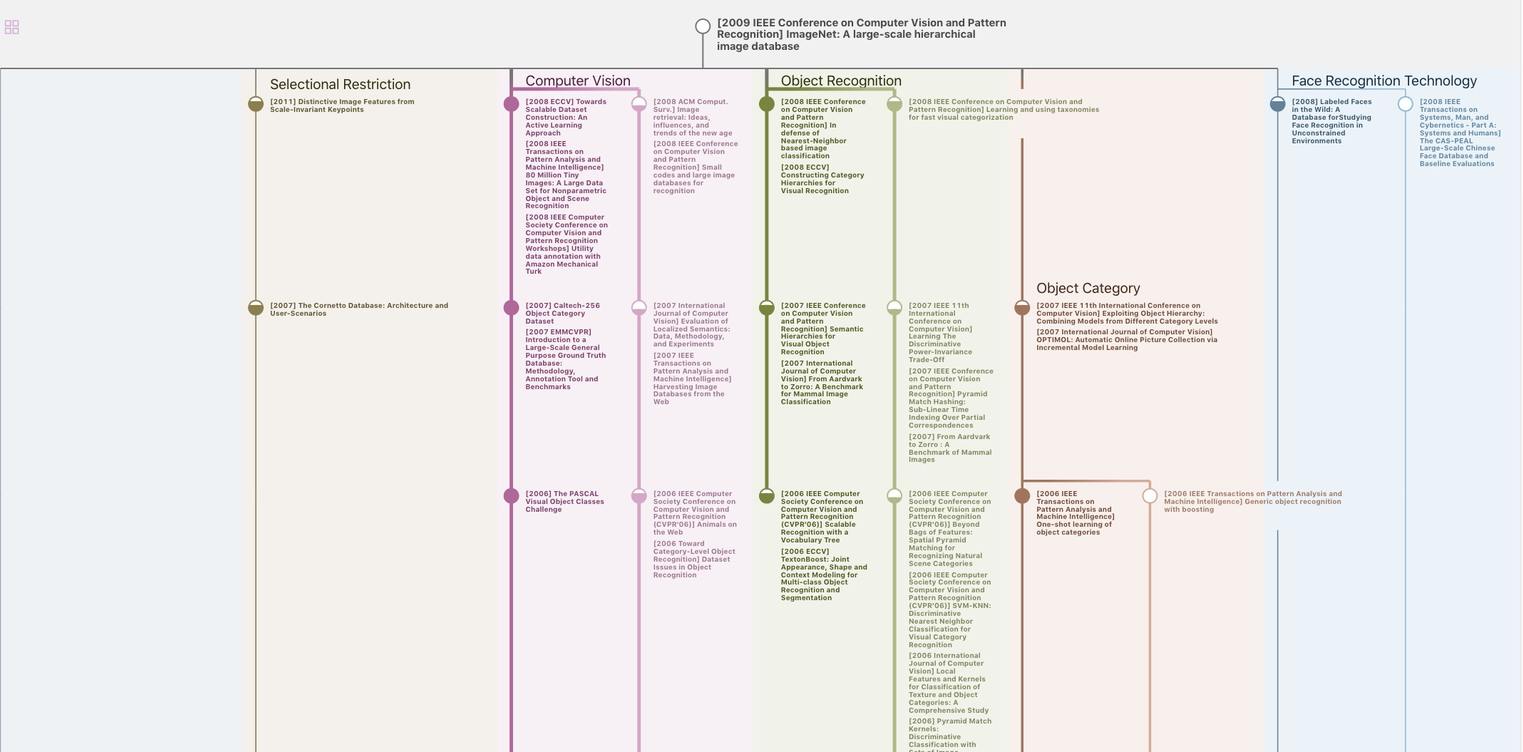
生成溯源树,研究论文发展脉络
Chat Paper
正在生成论文摘要