Few-Shot Object Detection Based on the Transformer and High-Resolution Network
CMC-COMPUTERS MATERIALS & CONTINUA(2023)
摘要
Now object detection based on deep learning tries different strategies. It uses fewer data training networks to achieve the effect of large dataset training. However, the existing methods usually do not achieve the balance between network parameters and training data. It makes the information provided by a small amount of picture data insufficient to optimize model parameters, resulting in unsatisfactory detection results. To improve the accuracy of few shot object detection, this paper proposes a network based on the transformer and high-resolution feature extraction (THR). High-resolution feature extraction maintains the resolution representation of the image. Channels and spatial attention are used to make the network focus on features that are more useful to the object. In addition, the recently popular transformer is used to fuse the features of the existing object. This compensates for the previous network failure by making full use of existing object features. Experiments on the Pascal VOC and MS-COCO datasets prove that the THR network has achieved better results than previous mainstream few shot object detection.
更多查看译文
关键词
Object detection, few shot object detection, transformer, high-resolution
AI 理解论文
溯源树
样例
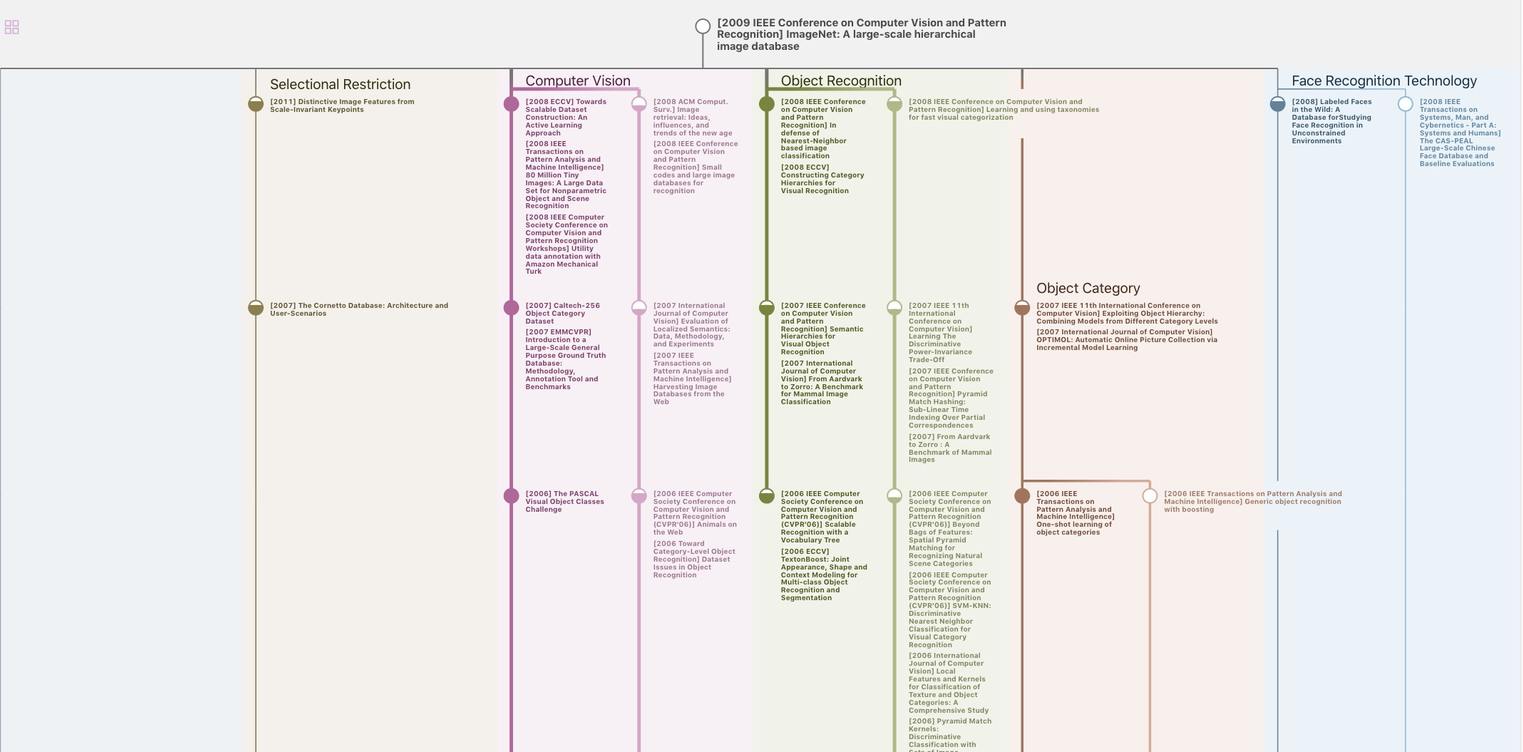
生成溯源树,研究论文发展脉络
Chat Paper
正在生成论文摘要