Comparison of the Prediction of BOF End-Point Phosphorus Content Among Machine Learning Models and Metallurgical Mechanism Model
STEEL RESEARCH INTERNATIONAL(2023)
摘要
The five machine learning models (MLM) of ridge regression, gradient boosting regression (GBR), support vector regression, random forest regression (RFR), convolutional neural network, and a metallurgical mechanism model (MMM) are compared in predicting the end-point P content in the basic oxygen furnace steelmaking process. The prediction accuracy of MMM is much lower than those of five MLM. The GBR and RFR models have the best performance, with the correlation coefficient values of 0.599 and 0.608, respectively. The smallest mean absolute relative error value of 0.155 and the root mean square error value of 0.00319 are obtained with GBR and RFR, respectively. The values of correlation coefficient after data distribution optimization for all MLM are increased two times higher than before. The second blowing time, lime weight, and oxygen consumption amount are evaluated to have the greatest impacts on the end-point P content. The end-point P content decreases with decreasing the second blowing time and with increasing the lime weight and the oxygen consumption amount. The GBR and RFR models are optimized by removing the variables with little impacts on the end-point P content. The highest prediction accuracy is obtained when 14 variables are remained.
更多查看译文
关键词
BOF, machine learning model, metallurgical mechanism model, prediction of end-point phosphorus contents, variable importance
AI 理解论文
溯源树
样例
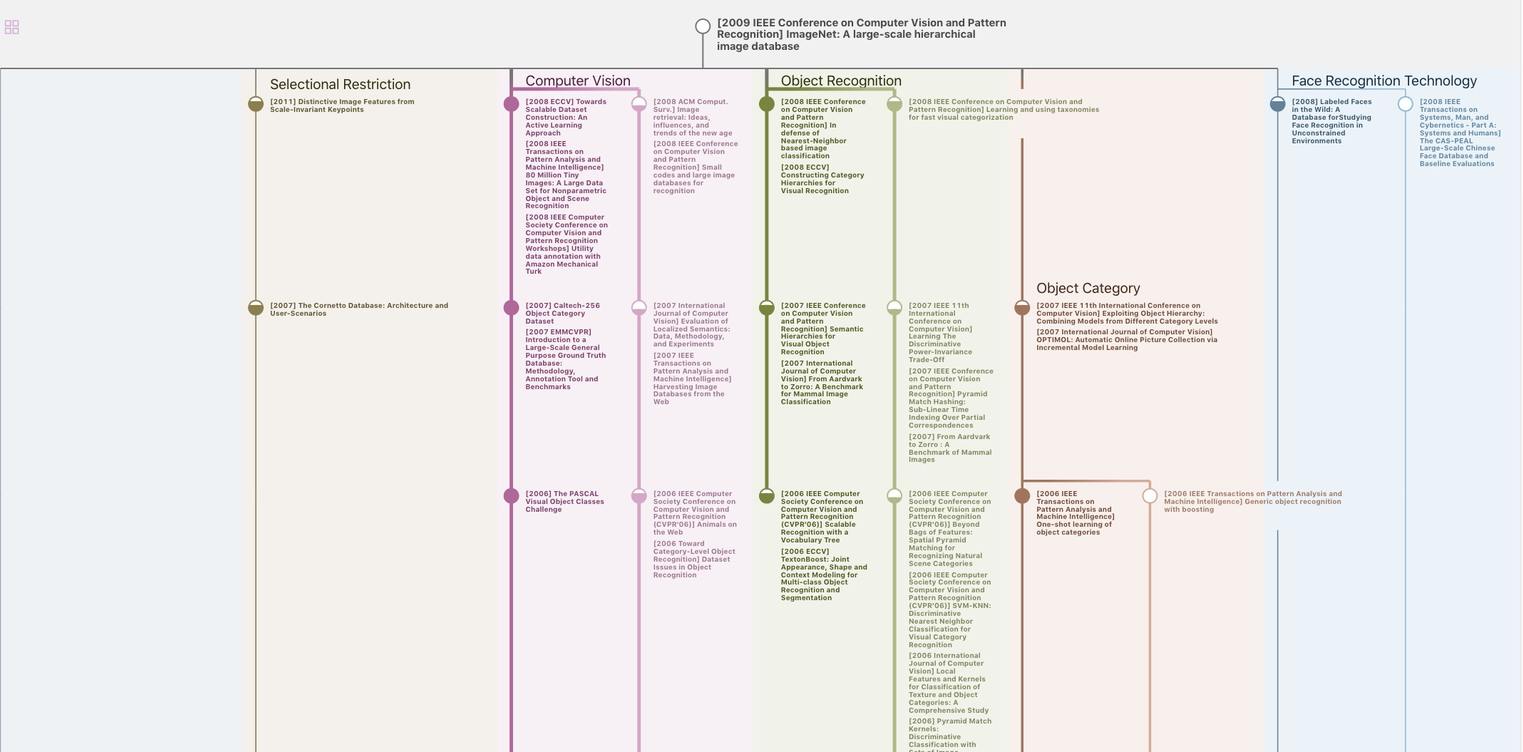
生成溯源树,研究论文发展脉络
Chat Paper
正在生成论文摘要