Evaluating Sex-Disparities in Machine Learning Decision Support Tools for Acute Coronary Syndrome Classification in the Emergency Department
CIRCULATION(2022)
摘要
Introduction: Women are less likely than men to be promptly diagnosed with acute coronary syndrome (ACS) and have worse post-ACS outcomes. These diagnostic failures are partially due to ACS findings on surface ECG manifesting differently in women, which may result in unnecessary delays in treatment. To narrow health disparities, we aim to investigate the sex-specific signatures of ACS as they appear on ECGs. Methods: This was a prospective observational cohort study of chest-pain patients evaluated for suspected ACS at 3 UPMC-affiliated tertiary care hospitals. After featurization, all ECG data were separately fed into 7 machine learning classifiers to predict ACS. We examined the results by sex. We also investigated two other methods: (1) building two independent models based respectively on the female and male subgroups and (2) building a model based on the initial total sample supplemented by the patients’ sex. We used Shapley values to explain the decision-making criteria of the models. We report the results for random forest, the best performing classifier. Results: Our sample consisted of 4132 patients (Age 59 ± 16; 47% female; 15% ACS). Machine learning models continue to disproportionately underperform in females across all classifiers evaluated. The sensitivity, specificity and false negative rate in the global model blinded to sex were 82.89%, 76.22% and 17.11% for men, and 67.39%, 74.16% and 32.61% for women (p<0.0001). This statistically significant gap could not be alleviated by building sex-specific models or feeding sex to the input of the model. Indeed, the rate of false negatives in sex-specific models and global models with sex as input were 14.67% and 18.42% for men, and 34.04% and 32.61% for women (p<0.0001). The explainability analysis of the sex-specific models revealed that STT configuration in lateral leads is most informative in women, whereas STT configuration in all leads and particularly in anterolateral leads most informative in men. Conclusions: Machine learning models display crucial sex differences in the ACS signatures on the ECG that consistently put women in a detrimental situation. The alternative methods investigated here are not adequate solutions for this disparity. Thus, further investigations should be conducted.
更多查看译文
关键词
acute coronary syndrome classification,acute coronary syndrome,coronary syndrome,sex-disparities
AI 理解论文
溯源树
样例
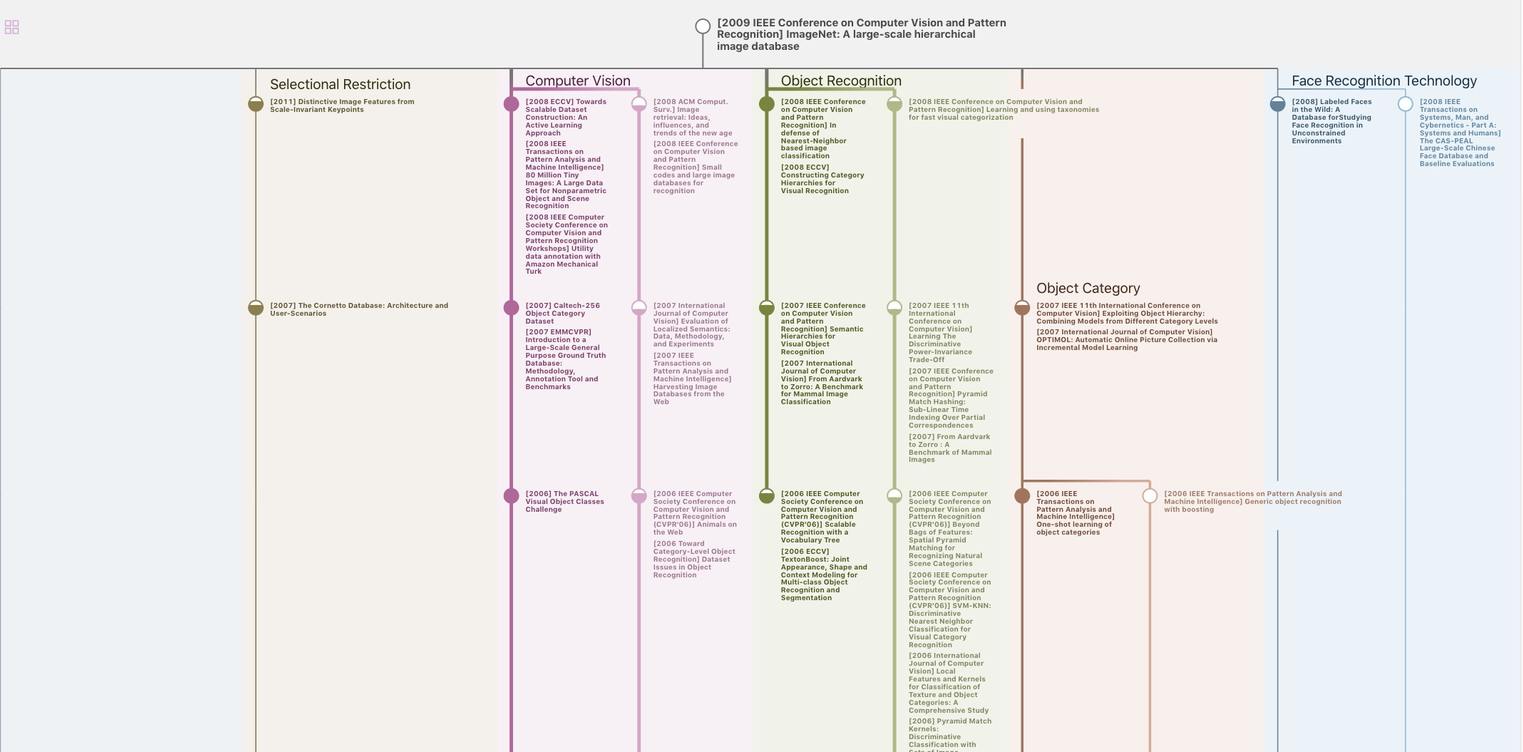
生成溯源树,研究论文发展脉络
Chat Paper
正在生成论文摘要