Multilayer Perceptron Generative Model via Adversarial Learning for Robust Visual Tracking
IEEE ACCESS(2022)
摘要
Visual tracking is an open and exciting field of research. The researchers introduced great efforts to be close to the ideal state of stable tracking of objects regardless of different appearances or circumstances. Owing to the attractive advantages of generative adversarial networks (GANs), they have been a promising area of research in many fields. However, GAN network architecture has not been thoroughly investigated in the visual tracking research community. Inspired by visual tracking via adversarial learning (VITAL), we present a novel network to generate randomly initialized masks for building augmented feature maps using multilayer perceptron (MLP) generative models. To obtain more robust tracking these augmented masks can extract robust features that do not change over a long temporal span. Some models such as deep convolutional generative adversarial networks (DCGANs) have been proposed to obtain powerful generator architectures by eliminating or minimizing the use of fully connected layers. This study demonstrates that the use of MLP architecture for the generator is more robust and efficient than the convolution-only architecture. Also, to realize better performance, we used one-sided label smoothing to regularize the discriminator in the training stage and the label smoothing regularization (LSR) method to reduce the overfitting of the classifier in the online tracking stage. The experiments show that the proposed model is more robust than the DCGAN model and offers satisfactory performance compared with the state-of-the-art deep visual trackers on OTB-100, VOT2019 and LaSOT datasets.
更多查看译文
关键词
Deep learning, generative adversarial network, multilayer perceptron, visual tracking
AI 理解论文
溯源树
样例
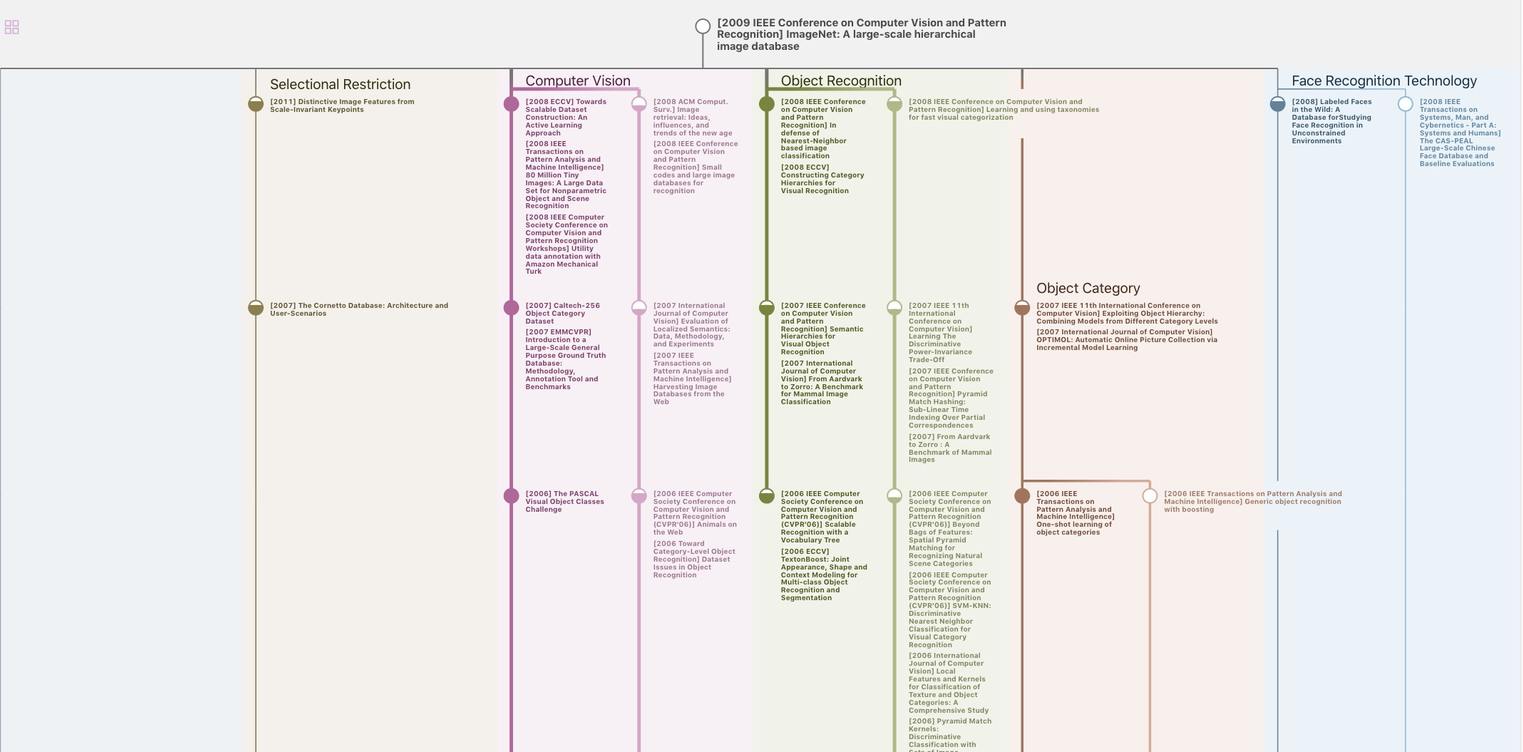
生成溯源树,研究论文发展脉络
Chat Paper
正在生成论文摘要