On improvability of hash clustering data from different sources by bipartite graph
PATTERN ANALYSIS AND APPLICATIONS(2022)
摘要
Clustering is a long-standing challenging task in pattern recognition and computer vision. In recent years, with development of multimedia technologies and explosive growth of data, the access to data is various. Clustering data from different feature sources has attracted considerable attentions for the remarkable clustering performance due to exploiting complementary information from data of different features. However, in fact, existing work often suffers from heavy computational load that restricts their capacity for large-scale datasets and most existing methods about fusing multi-view data are not perfect enough, namely, the quality of the joint matrix learned from multiple sources is not high enough. In this paper, we propose an efficient and effective clustering approach which combines sparse subspace learning with a bipartite graph for binary/hash codes produced from data of different sources by a collaborative discrete representation learning model that is an efficient and effective data fusion and binary code learning method. The bipartite graph that owns low time complexity is constructed to extract local geometric structure information for improving clustering performance. Extensive experiments performed on four benchmark datasets validate the efficiency and effectiveness of the proposed approach in comparison with ten state-of-the-art methods.
更多查看译文
关键词
Multiple sources,Binary/hash code learning,Sparse subspace learning,Bipartite graph
AI 理解论文
溯源树
样例
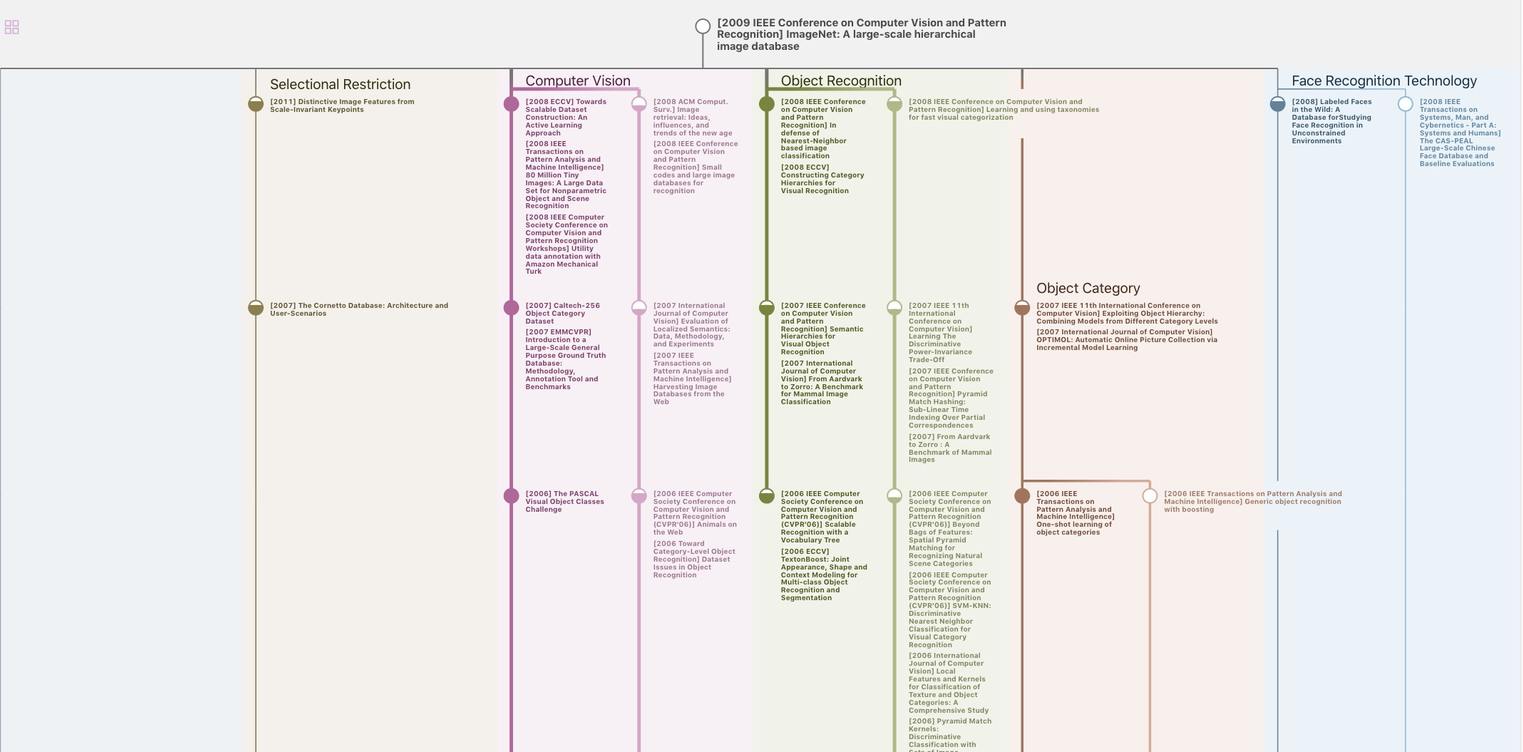
生成溯源树,研究论文发展脉络
Chat Paper
正在生成论文摘要