AI-aided design of multiscale lattice metastructures for controllable anisotropy
MATERIALS & DESIGN(2022)
摘要
Controllable anisotropy of the microscale lattice metastructures is the key to achieving designable mechanical properties of the macrostructures. However, a current computational challenge is precisely controlling the anisotropy of the design domain containing an ultra-large number of lattice structural units. In this work, we decompose the large-scale design domain into several mesoscale subregions and propose an artificial intelligence-aided design method that can automatically control the anisotropic properties of the mesoscale design domain. First, a 3D convolutional neural network is proposed to replace the time-consuming homogenization computation process, thus enabling fast prediction of equivalent mechanical properties of lattice structures with arbitrary geometrical and material parame-ters. Then, inspired by the encoded metaplot, the mesoscale design domain is divided into several sub-units with certain encoding. Finally, the combination type of the lattice structures of the subunits is adjusted automatically by a genetic algorithm according to the given target anisotropic properties. Furthermore, the effects of the lattice types, subunit numbers, and species number of subunits contained in the subregion on the accuracy of the inverse design are discussed. The results show that the proposed intelligent design method can effectively control the anisotropic properties of the mesoscale design domain.(c) 2022 The Author(s). Published by Elsevier Ltd. This is an open access article under the CC BY-NC-ND license (http://creativecommons.org/licenses/by-nc-nd/4.0/).
更多查看译文
关键词
AI-aided design, Controllable anisotropy, Lattice metastructure, 3D-CNN, Genetic algorithm
AI 理解论文
溯源树
样例
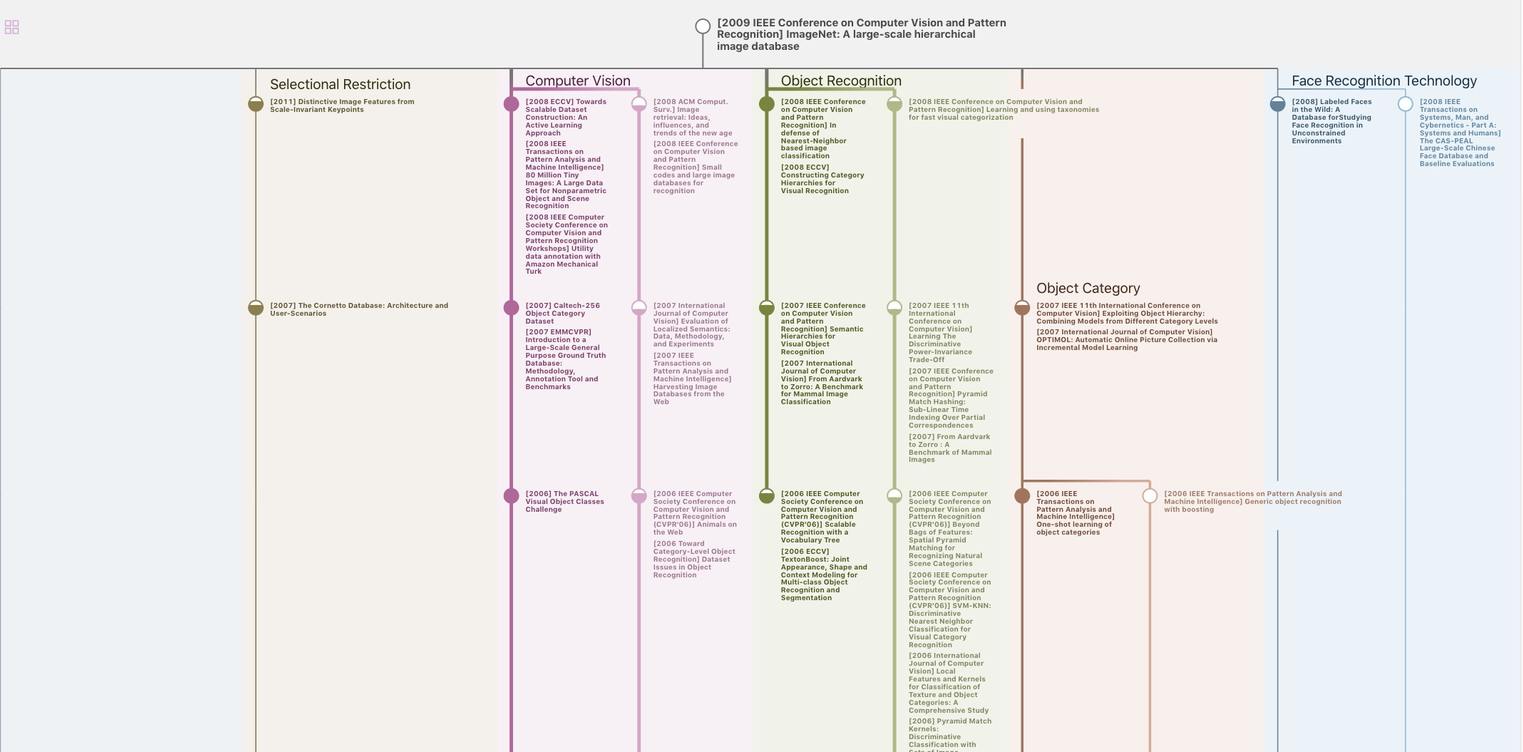
生成溯源树,研究论文发展脉络
Chat Paper
正在生成论文摘要