Evaluation of the Sample Size Requirements of Machine Learning Models Used in Engine Design and Research
INTERNATIONAL JOURNAL OF ENGINE RESEARCH(2023)
摘要
Machine Learning (ML) techniques have been effectively used to learn the intricate relationships between variables that play a significant role in the field of engine design. However, there are two challenges to this approach – (1) Identifying ML regression models that could capture the trends of a response variable, given a non-parametric training set of relatively small size, with an acceptable accuracy and response time, and (2) identifying the size of the dataset, with respect to the input parameters, to be used for training and validation of the ML models. There is not enough information in the literature to reach a consensus on the sampling size to be used for an engine design problem that would yield an acceptable measure of goodness-of-fit. This is evident from the varied size of the training/validation data size used within the engine research community, as will be elaborated on in the following sections. The objective of this paper is to provide an insight into the sampling size required by the different ML models to achieve an acceptable fit between the model and the data, to be used in three types of engine design/optimization problems – (1) conventional diesel combustion (CDC) engine performance over a wide range of speed and load, (2) cold-start operation of a direct-injected spark-ignition (DISI) engine, and (3) high-load performance of a dual-fuel reactivity-controlled compression ignition (RCCI) engine.
更多查看译文
关键词
Engine research,machine learning,Gaussian process regression,sample size requirements,CDC,RCCI,DISI
AI 理解论文
溯源树
样例
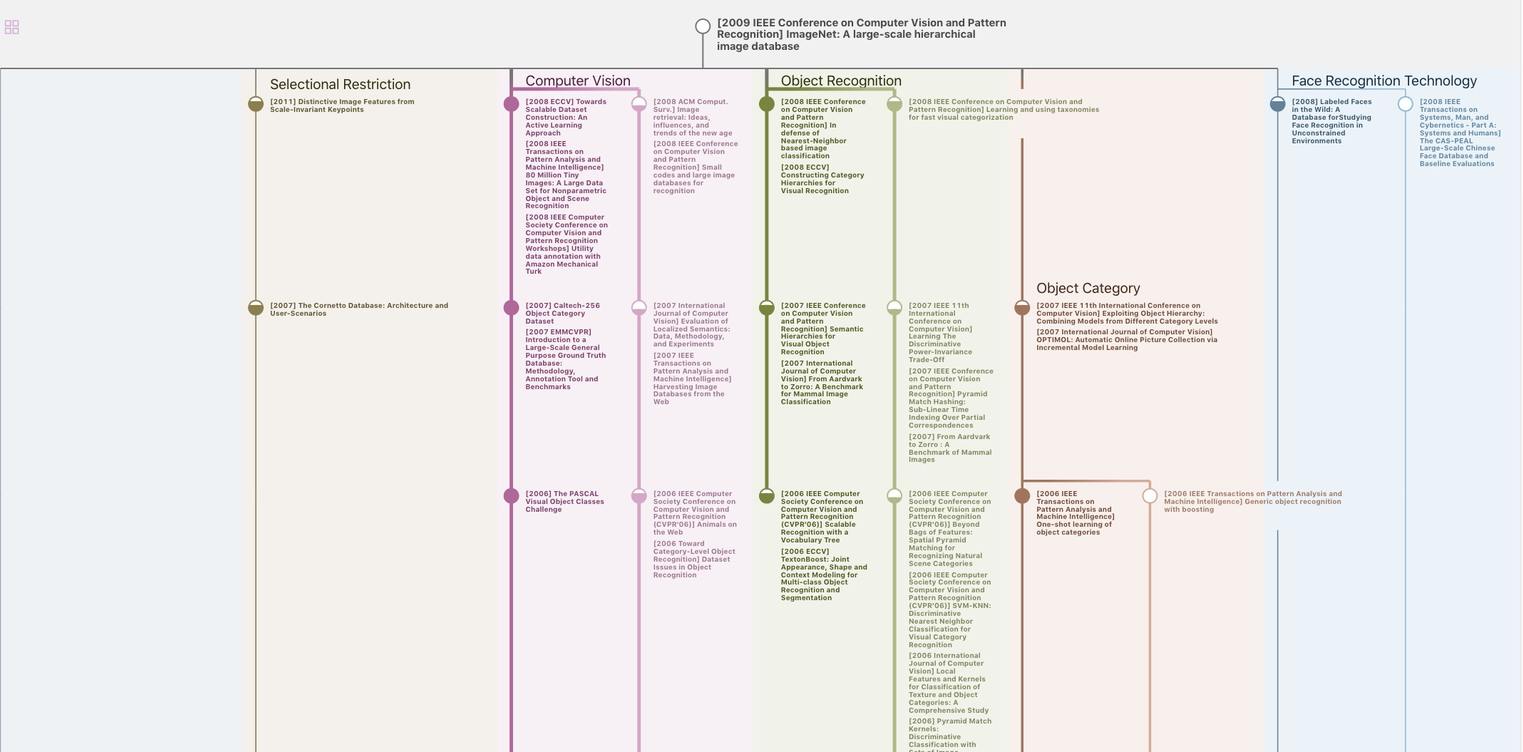
生成溯源树,研究论文发展脉络
Chat Paper
正在生成论文摘要