Enhanced machine learning model for meteo-oceanographic time-series prediction
ROMANIAN JOURNAL OF PHYSICS(2022)
摘要
Environmental state evaluation through its dynamic parameters plays a crucial role in assessment procedures to apply prediction models for early anomaly detection. This work presents the implementation of a Machine Learning Encoder-Decoder pattern used to in-situ environmental data (air and seawater temperature and wind speed) measured at Gloria offshore drilling platform - Romanian Black Sea Shelf. The station was chosen because there are no boundary interactions with the coastal region, enabling the multivariate, unidirectional time-series prediction algorithm. The model provided less than 5% mean absolute error (MAE) for 7 data points (months) forecast requiring the last 10 data points as input. The model accuracy enables the anomaly identification for meteo-oceanographic monthly average data. Future evolution of the seawater temperature extends the model for coastal areas with a less than 5% additional accuracy reduction. This model was developed mainly using available open-source frameworks permitting the integration with most of the visualization platforms available today.
更多查看译文
关键词
Environmental health assessment, machine-learning prediction, environmental modelling, machine learning, time-series prediction
AI 理解论文
溯源树
样例
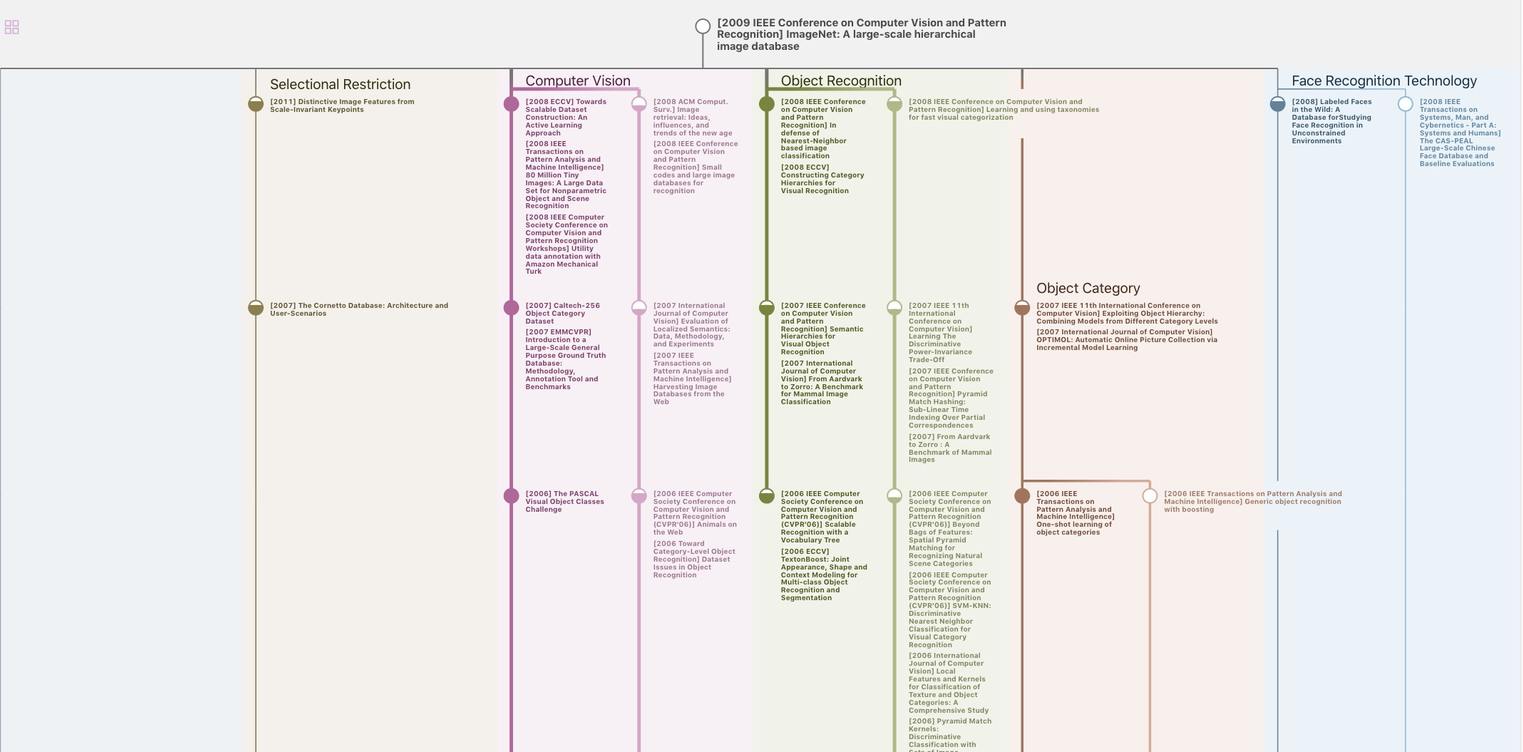
生成溯源树,研究论文发展脉络
Chat Paper
正在生成论文摘要