Contrastive learning-based structure preserving projection for hyperspectral images
INTERNATIONAL JOURNAL OF REMOTE SENSING(2022)
摘要
Unsupervised feature extraction methods have been widely applied to remove the huge amount of redundancy in hyperspectral images due to their effectiveness when the label information of samples is unreachable. However, because of the lack of label information, unsupervised feature extraction methods are deficient in the discriminant ability compared to supervised methods. When the number of samples is small, the effect of dimension reduction is usually not good enough. To address the problems, an unsupervised structure preserving projection method named contrastive learning based sparsity preserving projection (CL-SPP) is proposed in this paper. Firstly, CL-SPP increases the discriminant ability of samples by introducing the concept of positive and negative pairs, and adjusts the number of positive and negative pairs in the training set through a parameter. Then, by minimizing the contrastive loss function, CL-SPP makes the positive pairs more similar and the negative pairs less similar after projection. Moreover, the proposed contrastive learning-based method is also extended to the supervised case, as well as a general graph embedding model framework based on comparative learning. Experiments on three hyperspectral images demonstrate that the proposed methods have a better performance than related approaches. More impressively, the effect of CL-SPP is comparable to its supervised version.
更多查看译文
关键词
Feature extraction,sparse representation,collaborative representation,contrastive learning,hyperspectral images
AI 理解论文
溯源树
样例
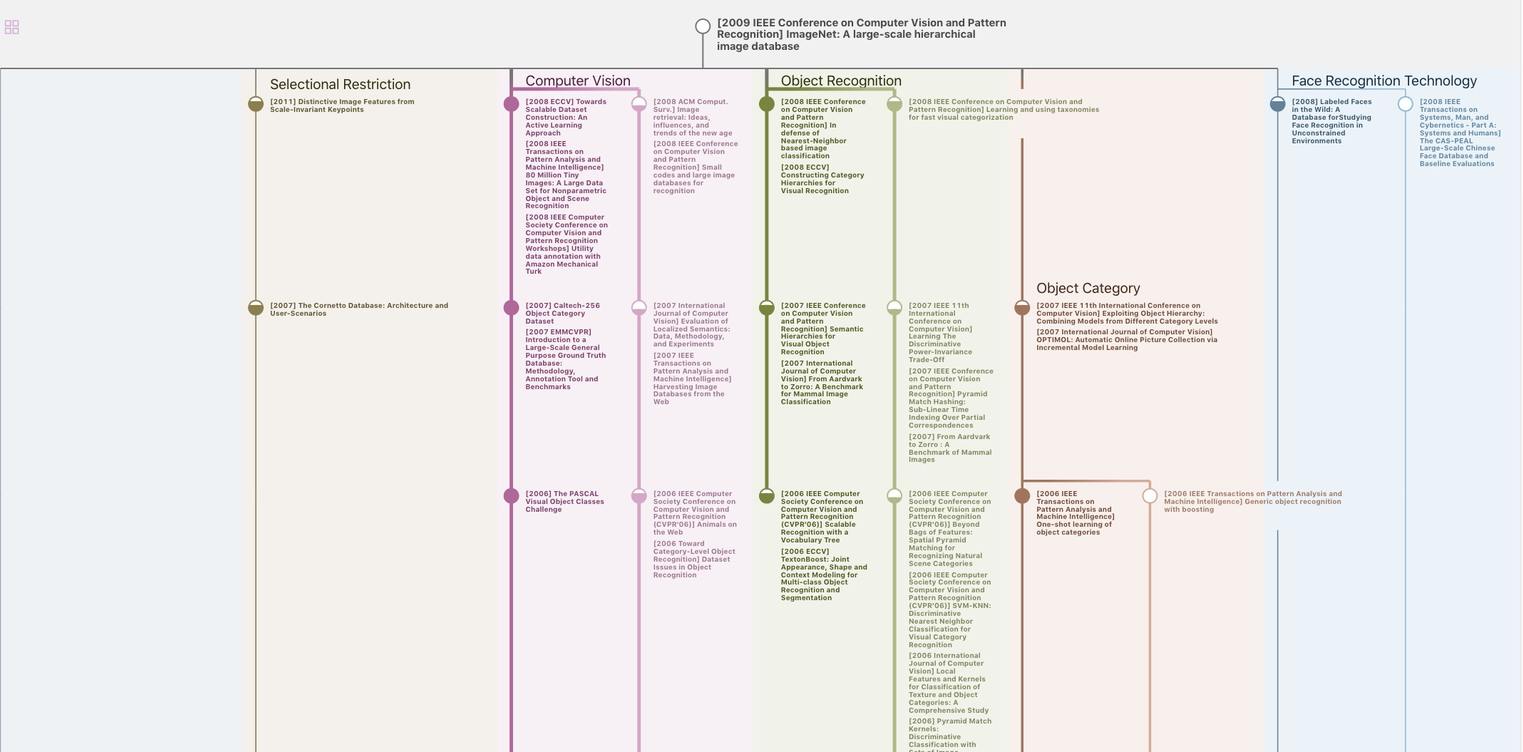
生成溯源树,研究论文发展脉络
Chat Paper
正在生成论文摘要