LLNet: Lightweight network with a channel and spatial attention mechanism for local climate zone classification from Sentinel-2 image
INTERNATIONAL JOURNAL OF CLIMATOLOGY(2023)
摘要
The local climate zone (LCZ) system is a landscape framework defining a universal understanding of urban microclimate and urban environment and is important for the research of urban thermal environment, regional planning and carbon cycle. Currently, most existing approaches are difficult to deal with the features of built-up area classes which are mainly related to three-dimensional structures, resulting in poor accuracy of these classes. The convolutional block attention module (CBAM) can get meaningful context to better represent features by re-weighting the features spatially and channel-wise. This study proposed a Lightweight-LCZ-Network (LLNet) based on CBAM and depthwise separable convolution for LCZ classification from Sentinel-2 image. The current largest LCZ classification data set So2Sat LCZ42 was employed to train and test the proposed model. Results indicated that the accuracy of the LLNet model achieved overall accuracy (OA) of 71.6% and Kappa coefficient of 0.688, realized an accuracy improvement by about 2%. In LLNet model, 65% of ordinary convolutions were displaced with depthwise separable convolutions, which decreased the amount of model parameters by 2/3 and maintained alike classification accuracy. In addition, the proposed model was applied for LCZ classification of Wuhan and Hefei cities to assess its generalization ability. The OA (Kappa coefficient) of the proposed model is 74.6% (0.72) for Wuhan and 77.5% (0.75) for Hefei, respectively. We concluded that the proposed LLNet model with strong robustness for LCZ classification from Sentinel-2 data has an effective trade-off between size and accuracy.
更多查看译文
关键词
local climate zone,depthwise separable convolution,deep learning,convolutional block attention module,Sentinnel,land surface classification
AI 理解论文
溯源树
样例
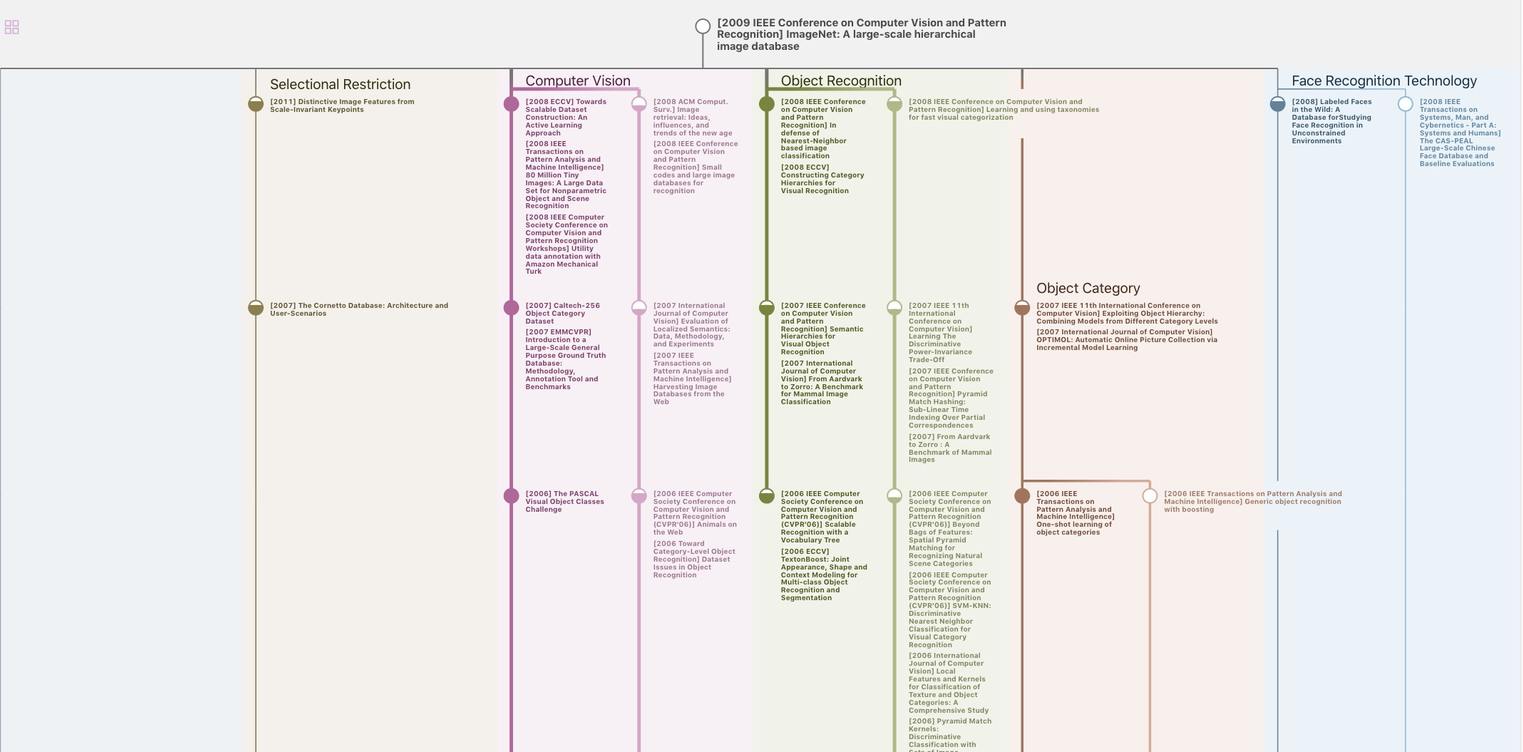
生成溯源树,研究论文发展脉络
Chat Paper
正在生成论文摘要