Spatial-Temporal Attention Network for Temporal Knowledge Graph Completion
DATABASE SYSTEMS FOR ADVANCED APPLICATIONS (DASFAA 2021), PT I(2021)
摘要
Temporal knowledge graph completion, which aims to predict missing links in temporal knowledge graph (TKG), is an important research task due to the incompleteness of TKG. Recently, TKG embedding methods have proved to be effective for this task. However, most of existing methods regard TKG as a set of independent facts and consequently ignore the implicit relevance among facts. Actually, as a kind of dynamic heterogeneous graph, the evolving graph structure of TKG is able to reflect a wealth of information. To this end, in this paper we regard temporal knowledge graph as heterogeneous and discrete spatial-temporal resource, and propose a novel spatial-temporal attention network to learn TKG embeddings by modeling spatial-temporal property of TKG while considering its special characteristics. Specifically, our model employs a Multi-Faceted Graph Attention Network (MFGAT) to extract rich structural information from the egocentric network of each entity. Additionally, an Adaptive Temporal Attention Mechanism (ADTAT) is utilized to flexibly model the correlation of entity representations in the time dimension. Finally, by combing our obtained representations with existing static KG completion methods, they can be extended to spatial-temporal versions to predict missing links in TKG while considering its inherent graph structure and time-evolving property. Experimental results on three real-world datasets demonstrate the superiority of our model over the state-of-the-art methods.
更多查看译文
关键词
Temporal knowledge graph completion, Temporal knowledge graph embedding learning, Spatial-temporal data mining
AI 理解论文
溯源树
样例
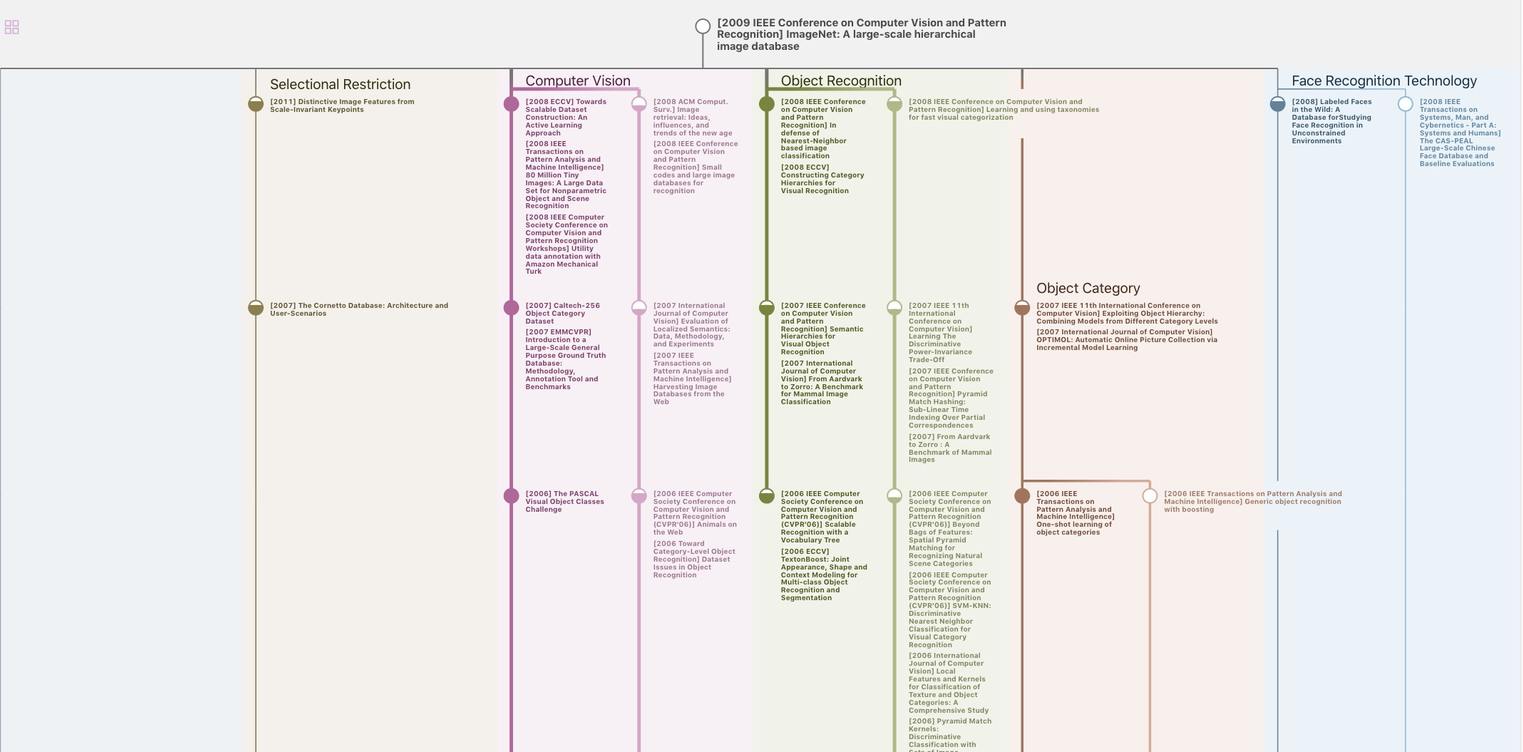
生成溯源树,研究论文发展脉络
Chat Paper
正在生成论文摘要