Cluster-based Federated Learning Framework for Intrusion Detection
2022 IEEE 13th International Symposium on Parallel Architectures, Algorithms and Programming (PAAP)(2022)
摘要
With the rapid development of Industrial Internet, the network intrusion detection has become particularly important. In the Industrial Internet, large-scale data is distributed in the edge nodes caused the joint analysis of network intrusion detection at each edge node has become necessary. Federated learning structure can avoid data out of local nodes to protect user privacy data. However, the data distribution is different for each edge nodes, which limits the effectiveness of federated learning models. We focus on the non-IID data features and propose a new cluster-based federated learning framework for network intrusion detection. In this method, we cluster clients into different communities by data labels, which the clients contain the similar proportion of data labels in the same community. Based on the clustering results, we decompose federated learning model aggregation into cluster aggregation and global aggregation by leveraging similarities both within and between clusters. We conduct extensive experiments based on UNSW_NB15 dataset. The results show that our method has better performance than FedAvg and FedProx. It can work well in scenarios with different distributions of data samples while ensuring data security and privacy protection.
更多查看译文
关键词
Intrusion detection,federated learning,cluster,similarity,non-IID
AI 理解论文
溯源树
样例
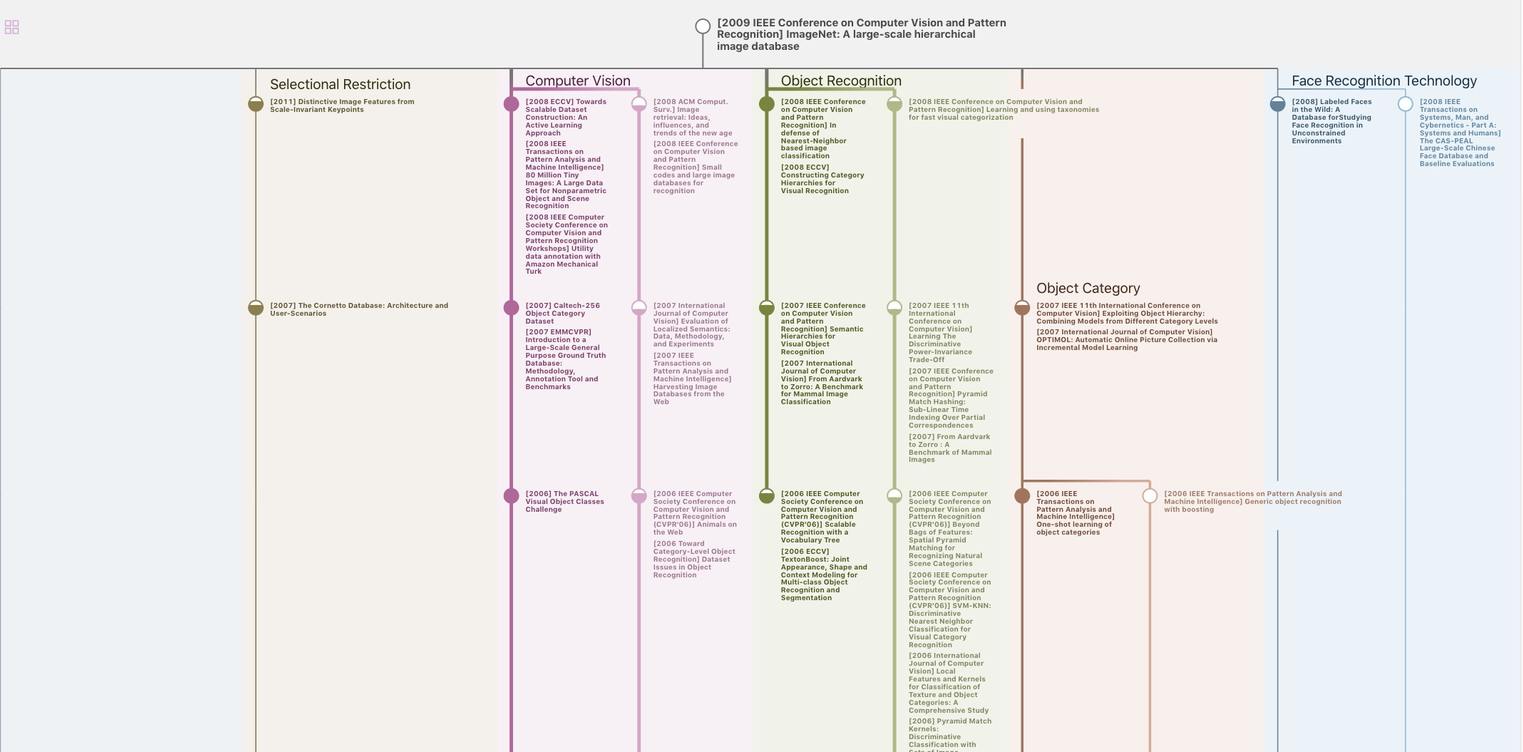
生成溯源树,研究论文发展脉络
Chat Paper
正在生成论文摘要