Fundamental Frequency Contour Classification: A Comparison between Hand-crafted and CNN-based Features
ICASSP 2019 - 2019 IEEE International Conference on Acoustics, Speech and Signal Processing (ICASSP)(2019)
摘要
In this paper, we evaluate hand-crafted features as well as features learned from data using a convolutional neural network (CNN) for different fundamental frequency classification tasks. We compare classification based on full (variable-length) contours and classification based on fixed-sized subcontours in combination with a fusion strategy. Our results show that hand-crafted and learned features lead to comparable results for both classification scenarios. Aggregating contour-level to file-level classification results generally improves the results. In comparison to the hand-crafted features, our examination indicates that the CNN-based features show a higher degree of redundancy across feature dimensions, where multiple filters (convolution kernels) specialize on similar contour shapes.
更多查看译文
关键词
Feature extraction,Task analysis,Music,Instruments,Frequency modulation,Shape
AI 理解论文
溯源树
样例
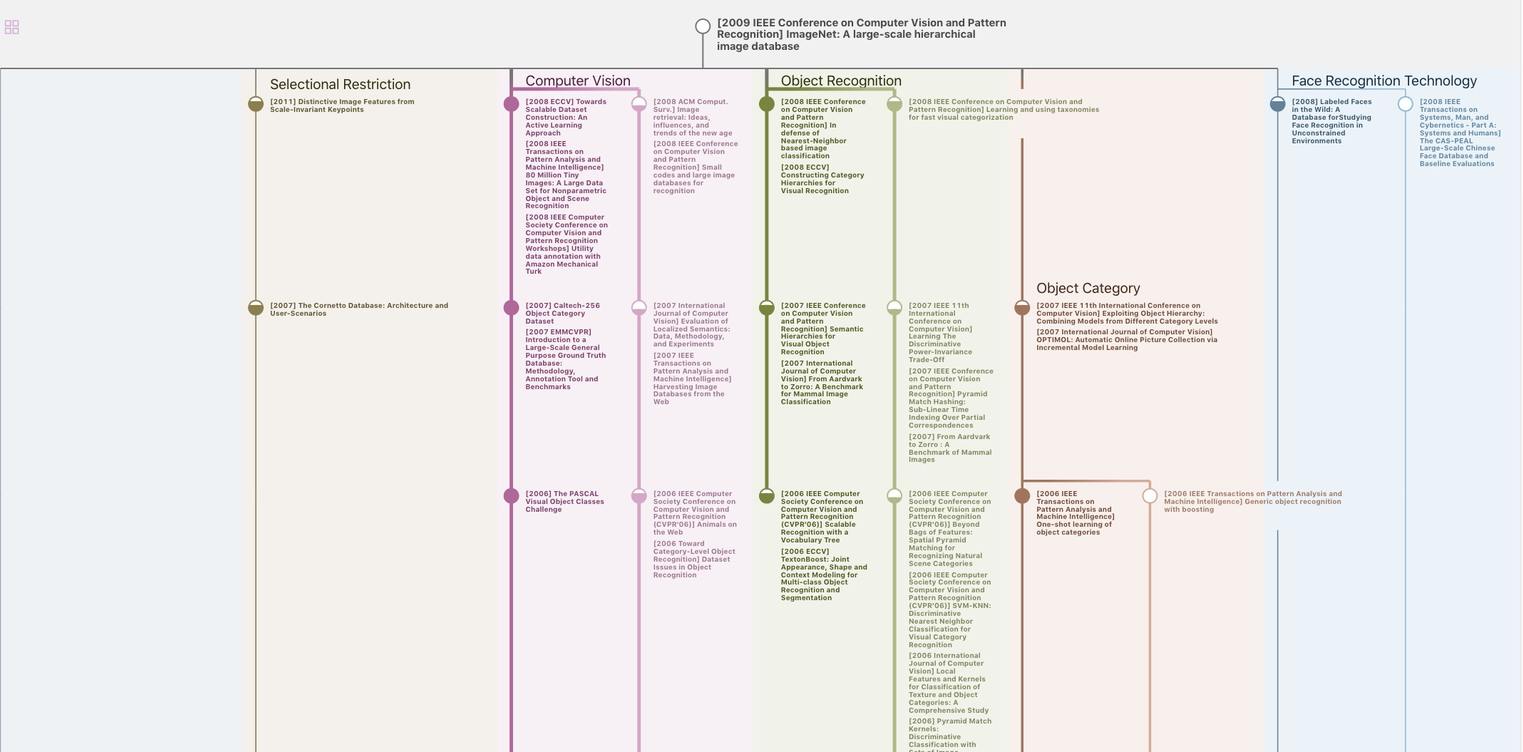
生成溯源树,研究论文发展脉络
Chat Paper
正在生成论文摘要