Cooperative Edge Caching via Federated Deep Deterministic Policy Gradient Learning in Fog-RANs
2022 IEEE Globecom Workshops (GC Wkshps)(2022)
摘要
In this paper, the cooperative edge caching problem in fog radio access networks (F-RANs) is investigated. On account of the non-deterministic polynomial hard (NP-hard) property of this problem, a federated deep deterministic policy gradient learning (FDDPG) based caching policy is proposed. By considering the dynamic content popularity and time varying requested content information, deep deterministic policy gradient learning (DDPG) is adopted to make optimal caching decisions. As the action selection is directly generated by policy network, DDPG can effectively solve the high dimensional action space caching problem. To address the over-consumption of computational resources, slow network convergence and leakage of user sensitive data, we apply reward weighted horizontal federated learning (RWHFL) in the training of DDPG network. Simulation results show the superiority of our proposed policy compared with the baselines in reducing the average content request delay.
更多查看译文
关键词
Fog radio access networks,cooperative edge caching,federated deep deterministic policy gradient learning,reward weighted horizontal federated learning
AI 理解论文
溯源树
样例
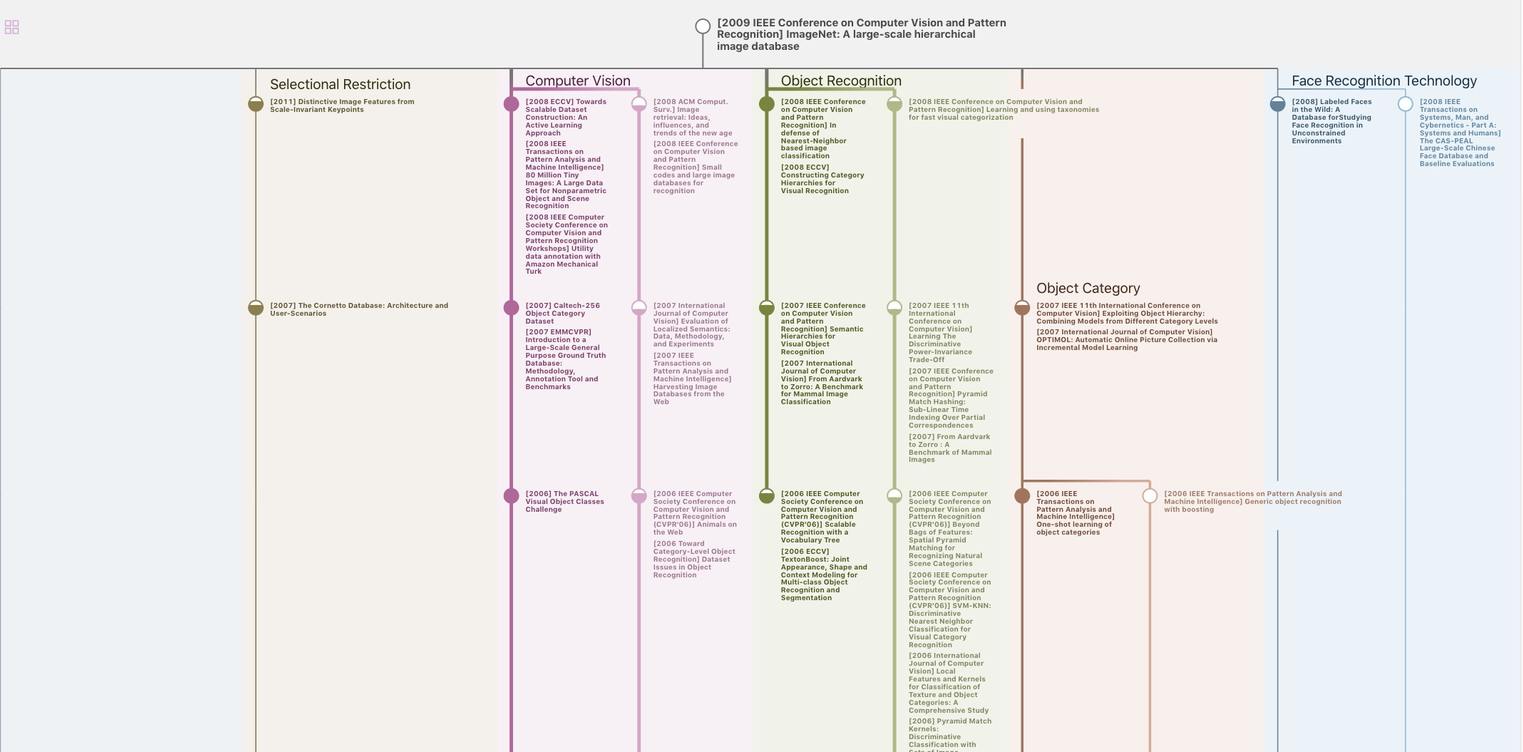
生成溯源树,研究论文发展脉络
Chat Paper
正在生成论文摘要