Detection Transformer: Ultrasonic Echo Signal Inclusion Detection with Transformer
2022 IEEE International Conference on Networking, Sensing and Control (ICNSC)(2022)
摘要
The micro non-metallic inclusions in metallic materials will seriously affect the quality and service life of products. The methods based on ultrasonic signal analysis are commonly used to detect inclusions in materials by detecting the abnormal part of the signal. However, few researchers attempt deep learning based methods for the field of ultrasonic inclusion detection. In this paper, we present Detection Transformer, a novel deep learning approach for non-metallic micro inclusion detection. Which regards ultrasonic echo inclusion detection as the problem of time series anomaly detection. We found that when ultrasonic wave propagates inside the material, the background noise or scattering noise generated by coarse grains increase the detection error rate of micro inclusions. Based on the traditional transformer architecture, the proposed Detection Transformer sets up the denoise self-attention mechanism module, allocate different weights by studying the importance of noise frequency characteristics, so as to remove the interference noise attached on original ultrasonic data. In addition, we remove the forward propagation layer in the transformer decoder, and use a full connection layer for detection at the end to detect all abnormal data at one time. We comprehensively evaluate the performance of Detection Transformer on the collected ultrasonic dataset, showing that Detection Transformer is an effective method in terms of accuracy and speed, outperforming state-of-the-art deep learning and signal processing methods, and able to provide meaningful solutions for the detection of inclusions in industrial materials.
更多查看译文
关键词
inclusion defects,anomaly detection,transformer,ultrasonic testing,noise suppression
AI 理解论文
溯源树
样例
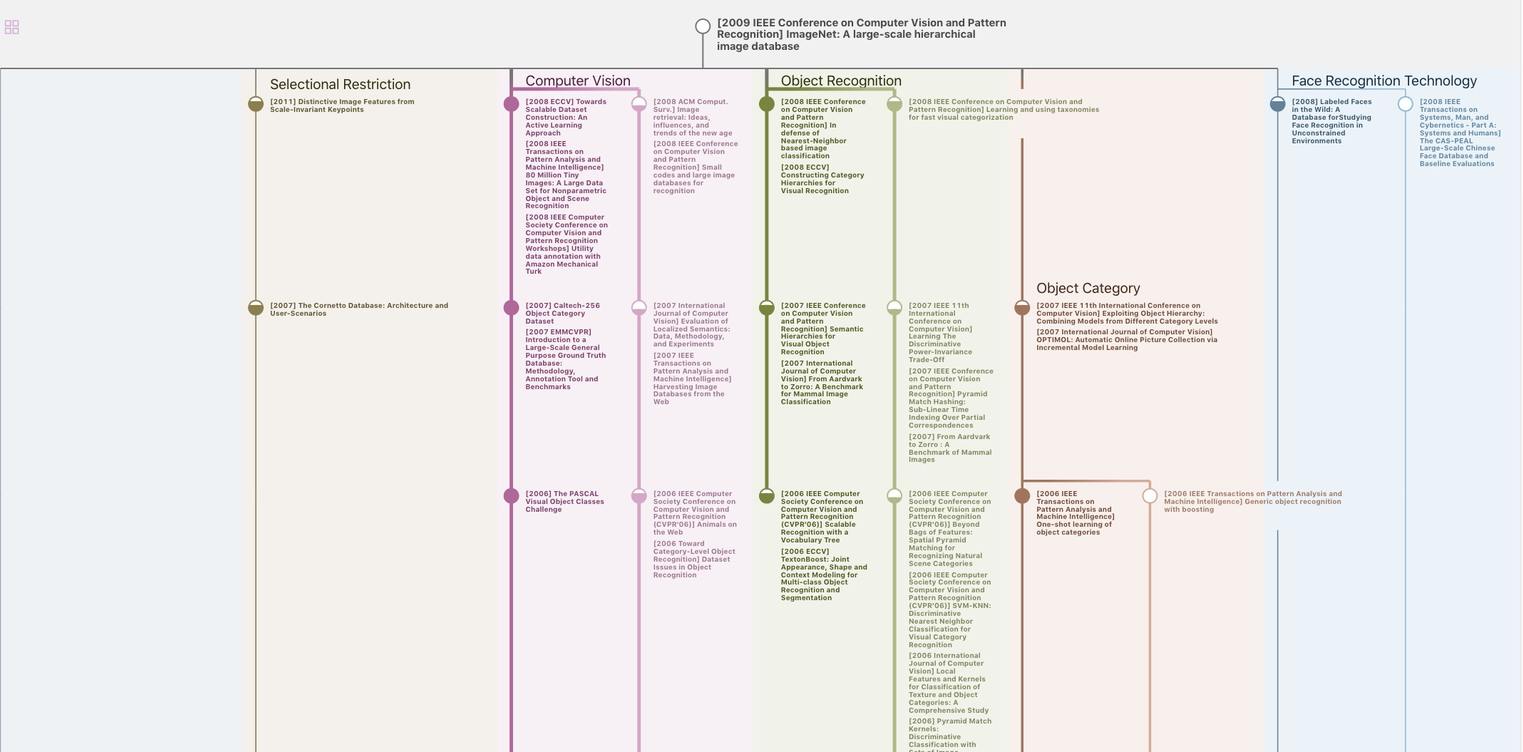
生成溯源树,研究论文发展脉络
Chat Paper
正在生成论文摘要