SS8: Source Data-free Domain Adaptation via Deep Clustering with Weighted Self-labelling
2022 IEEE International Conference on Networking, Sensing and Control (ICNSC)(2022)
摘要
As a new topic of transfer learning, source data-free domain adaptation (SFDA) is currently receiving a lot of attention due to the increasing demands on the safety and privacy. Due to that the dependence on the labelled source data is cut off by mining auxiliary information to regulate the self-training, the self-supervised learning becomes a promising SFDA solution. Among these proposed self-supervisions, the pseudo-label is a widely used and fundamental means to provide the supervision signal of category. However, the existing methods do not have a special strategy to mitigate the noise in the pseudo-labels well. This paper propose a deep clustering with weighted self-labelling (DC-WSL) approach to address the SFDA problem. Specifically, we first develop a low-entropy k-means method to generate more robust and credible clustering centers. And then, the pseudo-labels are assigned to all target data based on the distance from these centers, along with adaptive confidence scores as the weighted parameters. After that, based on these pseudo-labels with credible evaluation, we perform a self-training on the target domain under the regulation of deep clustering. The experimental results on two domain adaptation benchmarks confirm the effectiveness of the proposed method.
更多查看译文
关键词
Domain adaptation,Source data-free,Information entropy,Deep clustering
AI 理解论文
溯源树
样例
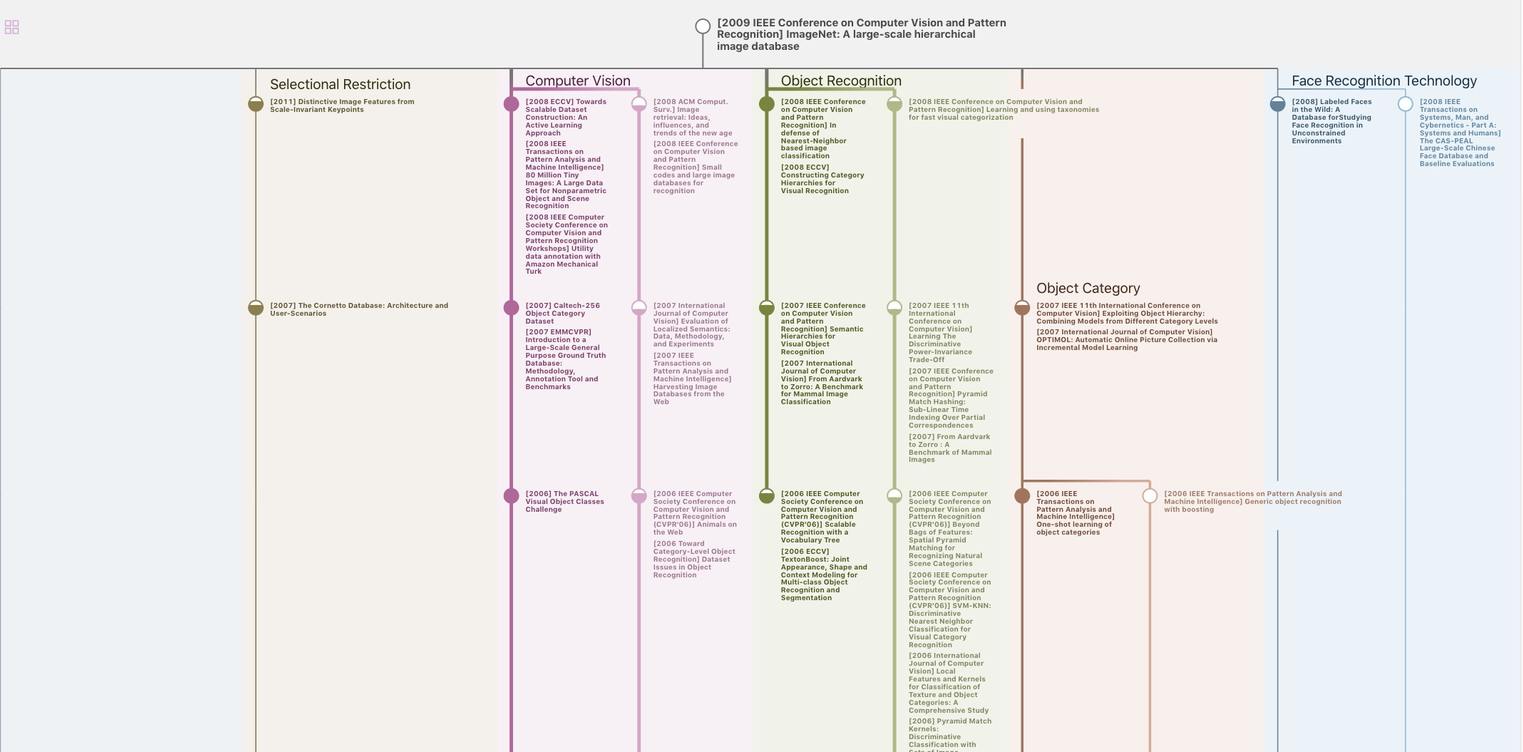
生成溯源树,研究论文发展脉络
Chat Paper
正在生成论文摘要