Learning Disease Specific Knowledge Graph from Unstructured Radiology Reports and Electronic Health Records (EHRs)
2022 17th International Conference on Emerging Technologies (ICET)(2022)
摘要
With an increasing growth rate, cancer is now the second biggest cause of mortality in the globe, killing a growing number of individuals every year. Electronic health records (EHRs) and radiology reports contain an enormous amount of clinical information. Case studies are crucial because they provide a wealth of medical knowledge regarding disease, medications, therapies, and other aspects. However, it is difficult to work with this information because it is often available in unstructured notes. Moreover, the production rate is quick, the volume of data is enormous, and the format is unique. The optimal solution to this problem is a knowledge graph (KG), a potent tool for information interaction and knowledge organisation. The goal of this study is to create a disease specific KG to present medical information from EHRs and unstructured radiology reports with focused semantics and granularity. In this paper, an entire KG construction framework built on the foundation of EHRs is proposed. The research proposed a KG schema with 7 classes and 15 different types of semantic relationships, and developed the proposed KG in Neo4j Desktop by using the extracted knowledge through Named-Entity Recognition (NER) from radiology reports and EHRs. The proposed study would be a learning step towards using KG intelligently to analyse the progression of diseases course, learnt from EHRs data.
更多查看译文
关键词
Electronic Health Records,EHRs,Knowledge Graph,KG,Neo4j
AI 理解论文
溯源树
样例
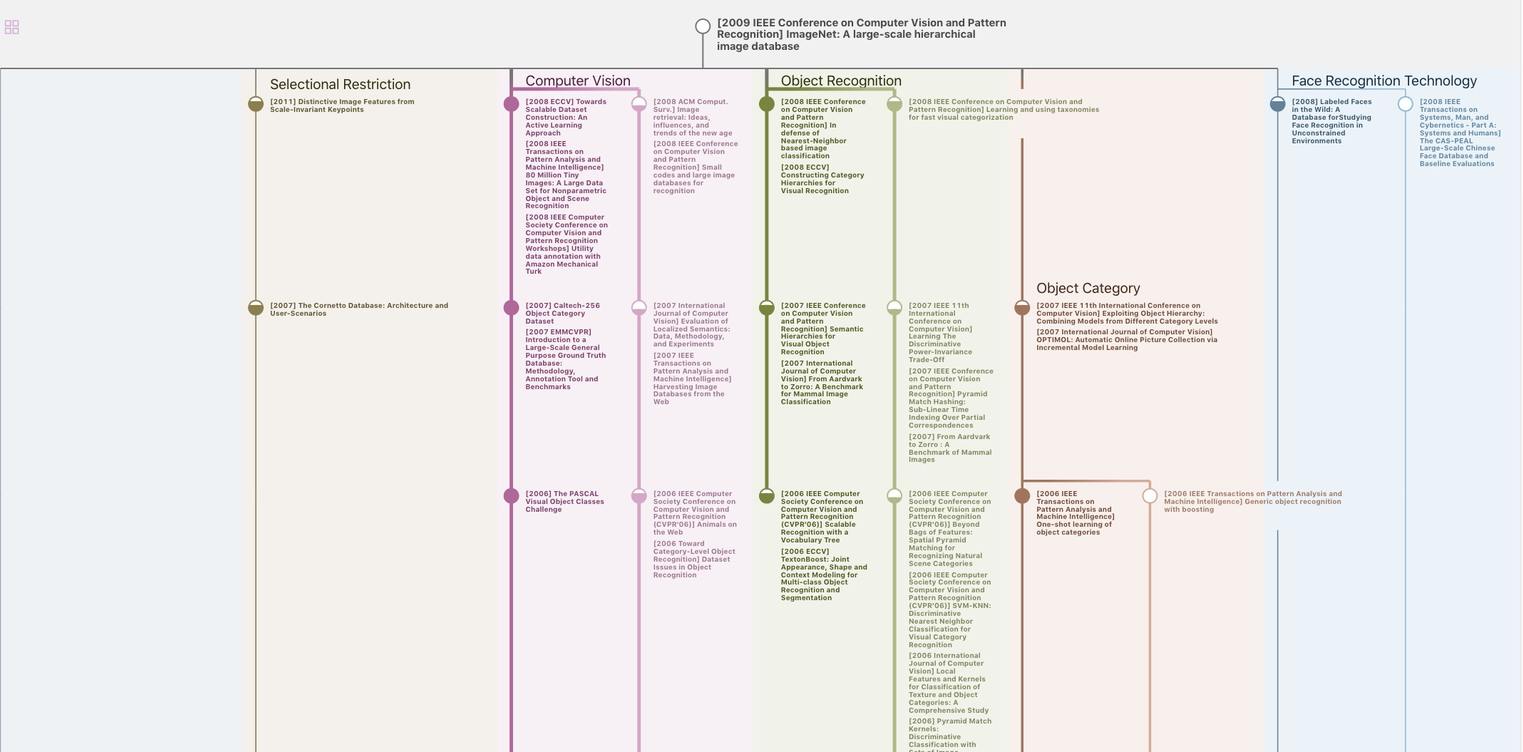
生成溯源树,研究论文发展脉络
Chat Paper
正在生成论文摘要