Intelligent dissipative particle dynamics: Bridging mesoscopic models from microscopic simulations via deep neural networks
Journal of Computational Physics(2023)
摘要
We develop a bottom-up coarse graining framework to construct mesoscopic force fields directly from microscopic dynamics by a machine learning technique. This scheme, called the intelligent dissipative particle dynamics (IDPD), holds various consistencies, including configuration, phase, static and dynamic consistencies that are incorporated into a deep neural network. The network is trained with full atomic data in a special way for preserving the natural symmetries of the system, and generates both the conservative and non-conservative force fields in the mesoscopic system. Subsequently, the force fields are used in the dissipative particle dynamics (DPD) that is considered as the effective mesoscopic dynamics resulting from the Mori–Zwanzig projection of the underlying atomic dynamics. To show the validity and applicability of IDPD, we coarse grain star polymer and methane fluids respectively, from full molecular dynamics (MD) simulations. Quantitative comparisons between the MD and IDPD indicate that the IDPD is able to reproduce both the static and dynamic properties of underlying MD system, with the aids of a pressure correction and a diffusion rescaling, even when coarse graining multiple molecules into a particle. It follows that the IDPD is competent for preserving the dynamic properties and coarse graining non-bonded molecules, which are common challenges for most of coarse graining methods.
更多查看译文
关键词
Dissipative particle dynamics,Molecular dynamics,Deep neural network,Coarse graining
AI 理解论文
溯源树
样例
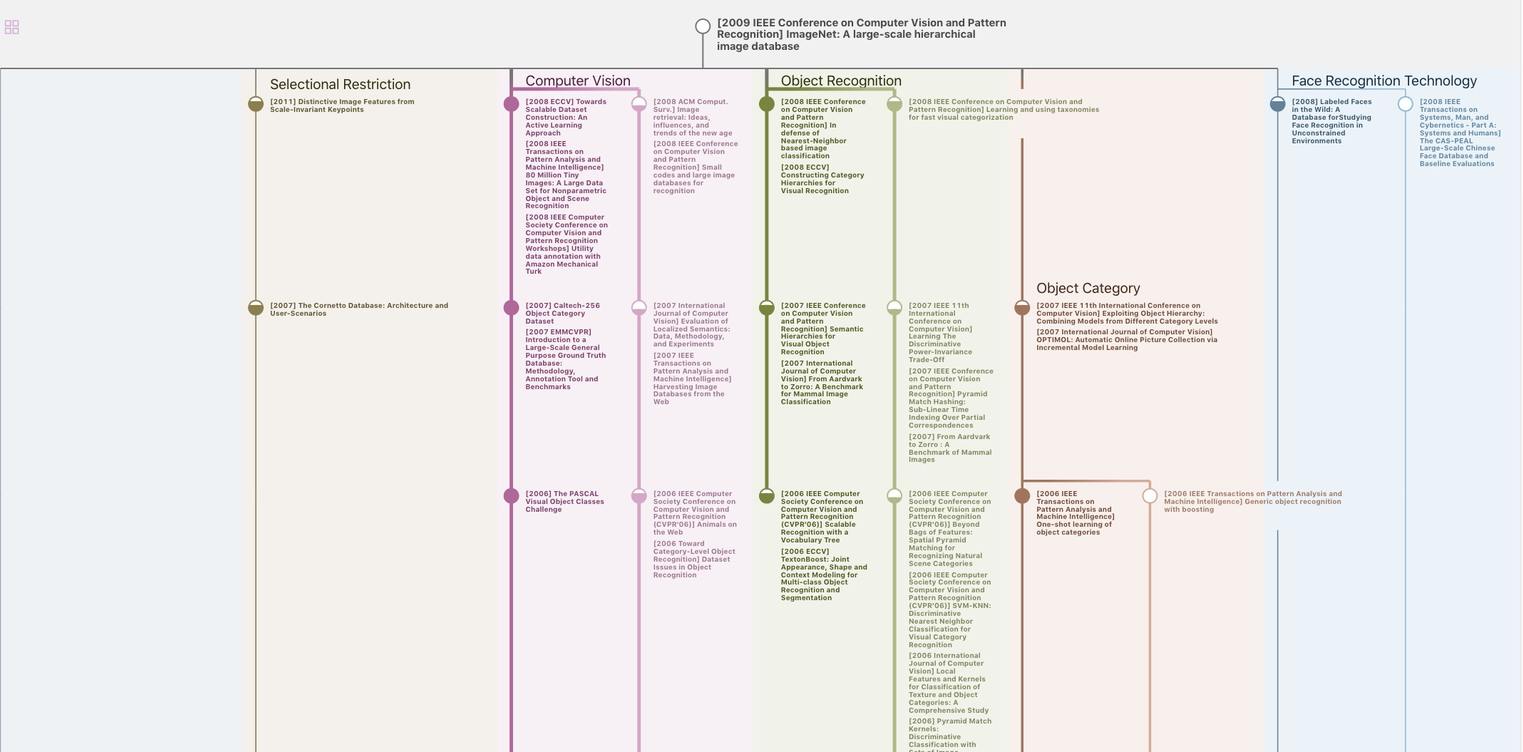
生成溯源树,研究论文发展脉络
Chat Paper
正在生成论文摘要