Performances of a Seq2Seq-LSTM methodology to predict crop rotations in Quebec
Smart Agricultural Technology(2023)
摘要
To meet global food requirements while responding to the environmental challenges of the 21st century, an agrienvironmental transition towards sustainable agricultural practices is necessary. Crop rotation is an ancestral practice and is a pillar of sustainable agriculture. However, this practice requires more organization on the part of producers for the management of crop inputs. That is why the development of a methodology for forecasting crop rotations in the medium term and at the field level is necessary. However, to date, only a methodology based on the Seq2Seq-LSTM has been theorized without being tested on a concrete case of application. The objective of this article is therefore to evaluate the performance of a Seq2Seq-LSTM methodology to predict crop rotations on a real case. The methodology was applied to a problem of crop rotation prediction for field crop farms in Que ' bec, Canada. Using the Recall(N) metric and a historical sequence of length 6, the next 3 crops grown in a field can be predicted with over 81% success when considering 10 selected options. In addition, the methodology was augmented with contextual information such as economic and meteorological data to refine the forecasts. This augmentation systematically improves the performance of the model. This observation provides a relevant line of research for identifying other factors that influence producers' decision-making on crop rotation.
更多查看译文
关键词
Agriculture 4.0,Crop rotation,Deep learning,Seq2Seq,LSTM
AI 理解论文
溯源树
样例
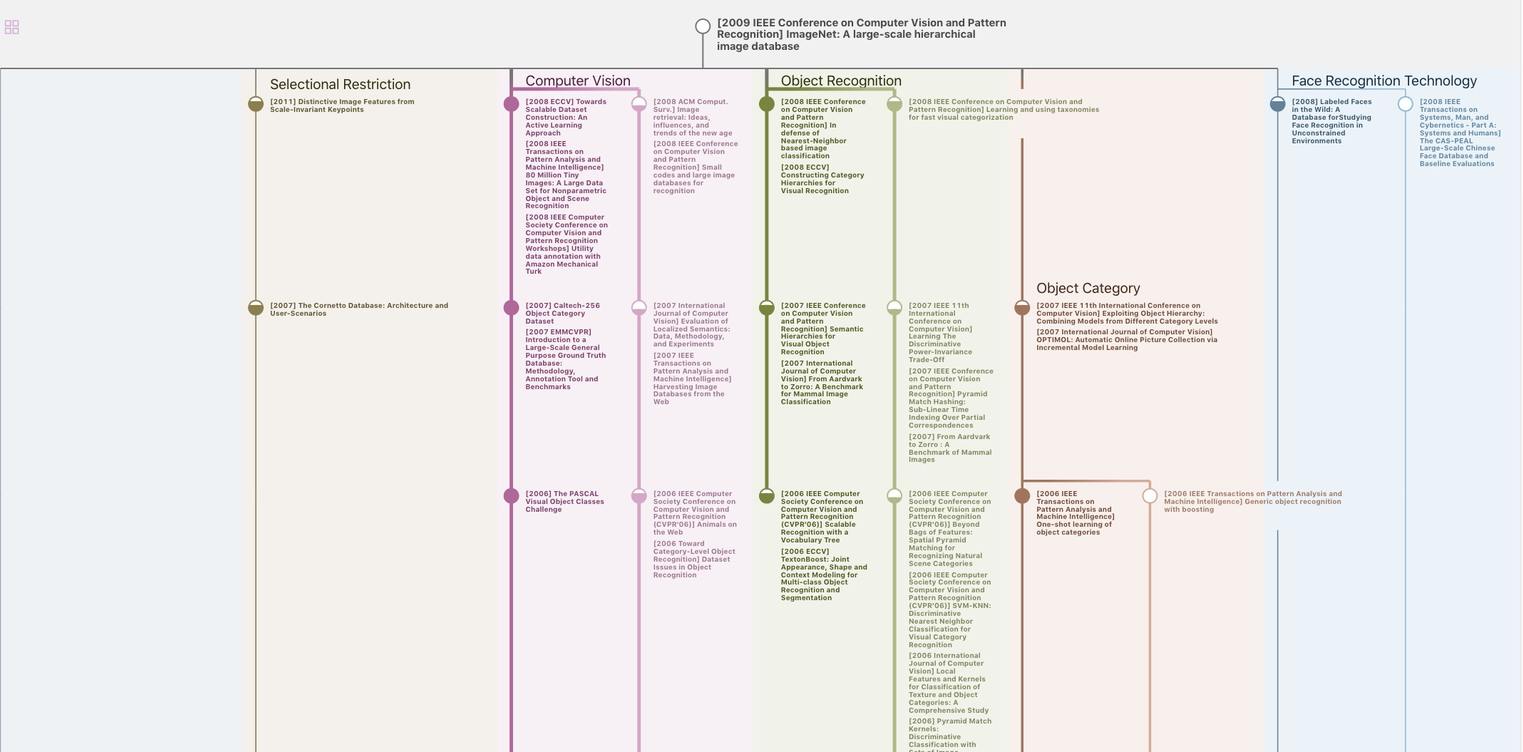
生成溯源树,研究论文发展脉络
Chat Paper
正在生成论文摘要