BULB: Lightweight and Automated Load Balancing for Fast Datacenter Networks
Proceedings of the 51st International Conference on Parallel Processing(2022)
摘要
Load balancing is essential for datacenter networks. However, prior solutions have significant limitations: they either are oblivious to congestion or involve a daunting and time-consuming parameter-tunning task over their heuristics for achieving good performance. Thus, we ask: is it possible to learn to balance datacenter traffic? While deep reinforcement learning (DRL) sounds like a good answer, we observe that it is too heavyweight due to the long decision-making latency. Therefore, we introduce BULB, a lightweight and automated datacenter load balancer. BULB learns link weights to guide the end-hosts to spread traffic, so as to free the central agent from quick flow-level decision-making. BULB offline trains a DRL agent for optimizing link weights but employs an imitation learning based approach to faithfully translate this agent's DNN to a decision tree for online deployment. We implement a BULB prototype with a popular machine learning framework and evaluate it extensively in ns-3. The results show that BULB achieves up to 36.6%/56.4%, 19.9%/42.5%, 35.9%/54.8%, and 45.1%/67.7% better average/tail flow completion time than ECMP, CONGA, LetFlow, and Hermes, respectively. Moreover, BULB reduces the decision latency by 175 times while incurring only 2% performance loss after converting the DNN into a decision tree.
更多查看译文
关键词
Data center networks, Load balancing, DRL, Imitation learning
AI 理解论文
溯源树
样例
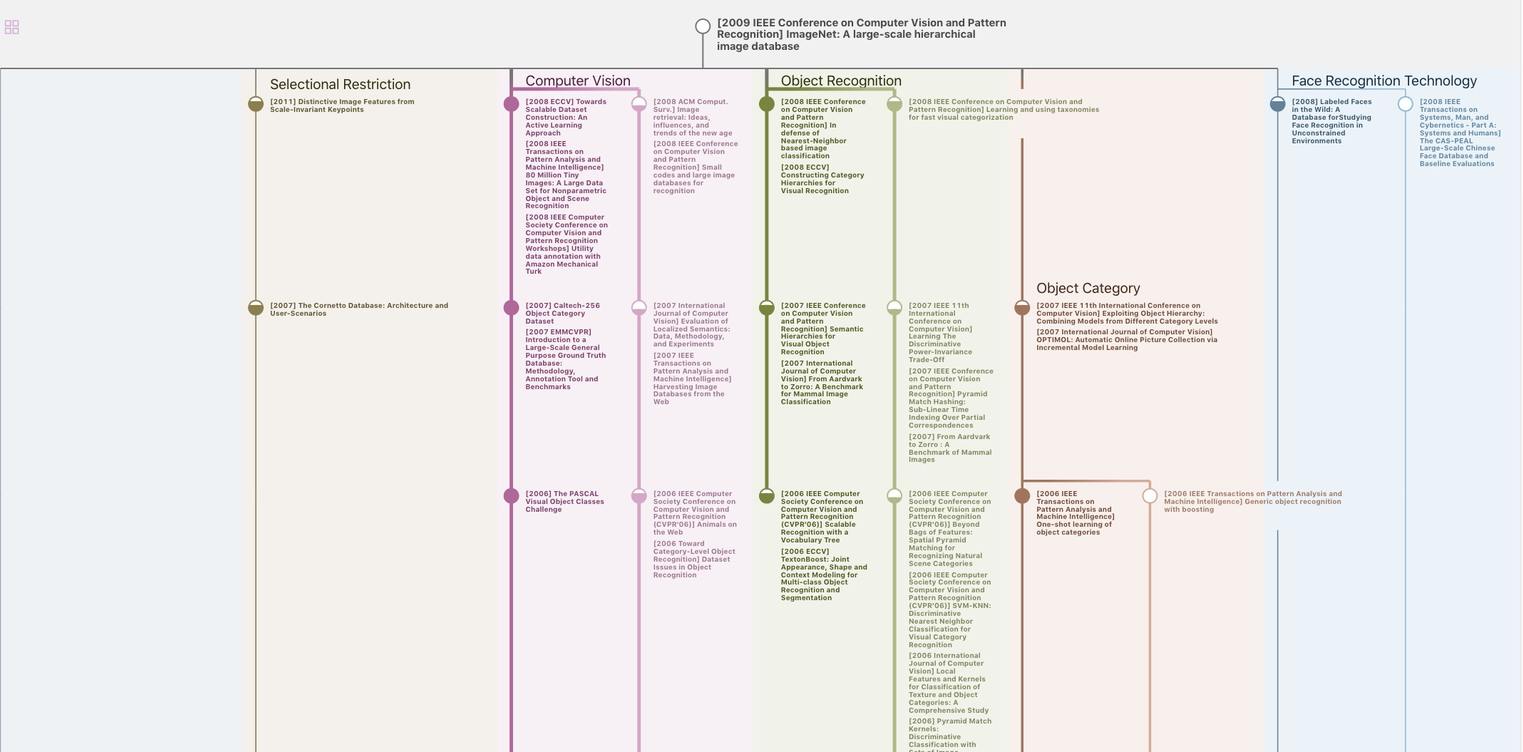
生成溯源树,研究论文发展脉络
Chat Paper
正在生成论文摘要