Multi-Task Weakly Supervised Learning for OriginCDestination Travel Time Estimation
arxiv(2023)
摘要
Travel time estimation from GPS trips is of great importance to order duration, ridesharing, taxi dispatching, etc. However, the dense trajectory is not always available due to the limitation of data privacy and acquisition, while the origin-destination (OD) type of data, such as NYC taxi data, NYC bike data, and Capital Bikeshare data, is more accessible. To address this issue, this paper starts to estimate the OD trips travel time combined with the road network. Subsequently, a Multi-task Weakly Supervised Learning Framework for Travel Time Estimation (MWSL-TTE) has been proposed to infer transition probability between roads segments, and the travel time on road segments and intersection simultaneously. Technically, given an OD pair, the transition probability intends to recover the most possible route. And then, the output of travel time is equal to the summation of all segments' and intersections' travel time in this route. A novel route recovery function has been proposed to iteratively maximize the current routes' co-occurrence probability, and minimize the discrepancy between routes' probability distribution and the inverse distribution of routes' estimation loss. Moreover, the expected log-likelihood function based on a weakly-supervised framework has been deployed in optimizing the travel time from road segments and intersections concurrently. We conduct experiments on a wide range of real-world taxi datasets in Xi'an and Chengdu and demonstrate our method's effectiveness on route recovery and travel time estimation.
更多查看译文
关键词
Travel time estimation,urban computing,weakly supervised learning
AI 理解论文
溯源树
样例
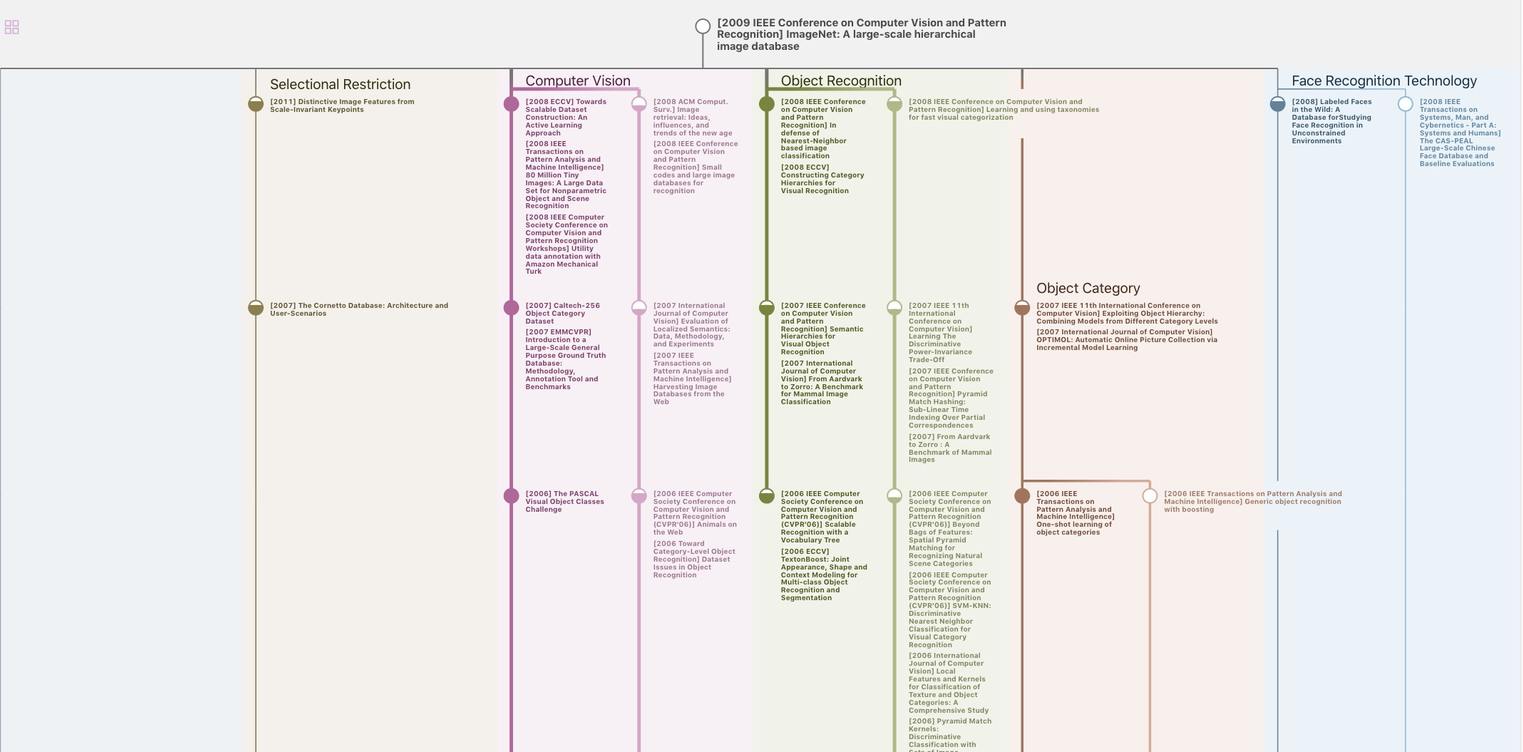
生成溯源树,研究论文发展脉络
Chat Paper
正在生成论文摘要