Building a Fuel Moisture Model for the Coupled Fire-Atmosphere Model WRF-SFIRE from Data: From Kalman Filters to Recurrent Neural Networks
arxiv(2023)
摘要
The current fuel moisture content (FMC) subsystems in WRF-SFIRE and its workflow system WRFx use a time-lag differential equation model with assimilation of data from FMC sensors on Remote Automated Weather Stations (RAWS) by the extended augmented Kalman filter. But the quality of the result is constrained by the limitations of the model and of the Kalman filter. We observe that the data flow in a system consisting of a model and the Kalman filter can be interpreted to be the same as the data flow in a recurrent neural network (RNN). Thus, instead of building more sophisticated models and data assimilation methods, we want to train a RNN to approximate the dynamics of the response of the FMC sensor to a time series of environmental data. Because standard AI approaches did not converge to reasonable solutions, we pre-train the RNN with special initial weights devised to turn it into a numerical solver of the differential equation. We then allow the AI training machinery to optimize the RNN weights to fit the data better. We illustrate the method on an example of a time series of 10h-FMC from RAWS and weather data from the Real-Time Mesoscale Analysis (RTMA).
更多查看译文
关键词
fuel moisture model,recurrent neural networks,kalman filters,fire-atmosphere,wrf-sfire
AI 理解论文
溯源树
样例
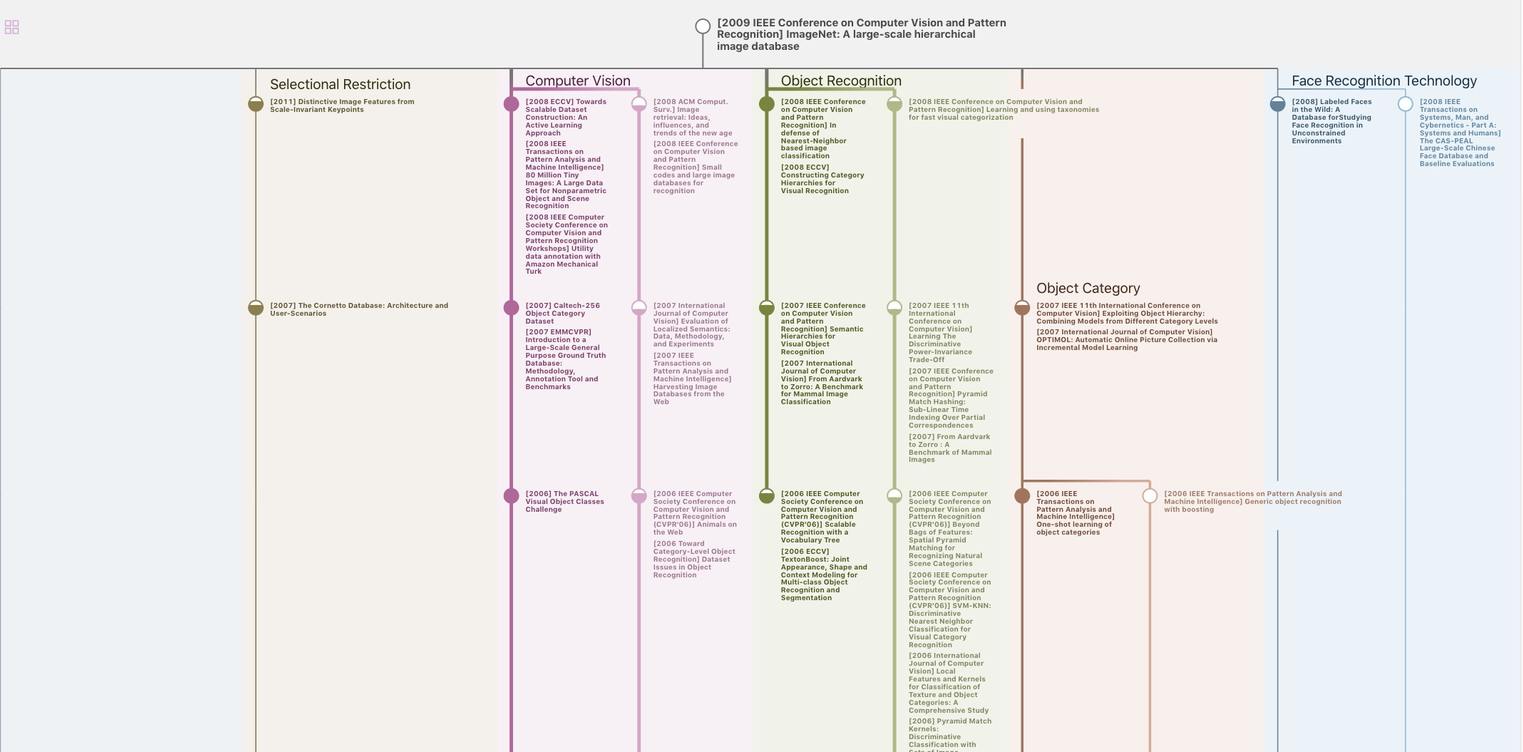
生成溯源树,研究论文发展脉络
Chat Paper
正在生成论文摘要