Scalable Batch Acquisition for Deep Bayesian Active Learning
SDM(2023)
摘要
In deep active learning, it is especially important to choose multiple examples to markup at each step to work efficiently, especially on large datasets. At the same time, existing solutions to this problem in the Bayesian setup, such as BatchBALD, have significant limitations in selecting a large number of examples, associated with the exponential complexity of computing mutual information for joint random variables. We, therefore, present the Large BatchBALD algorithm, which gives a well-grounded approximation to the BatchBALD method that aims to achieve comparable quality while being more computationally efficient. We provide a complexity analysis of the algorithm, showing a reduction in computation time, especially for large batches. Furthermore, we present an extensive set of experimental results on image and text data, both on toy datasets and larger ones such as CIFAR-100.
更多查看译文
关键词
scalable batch acquisition,deep,learning
AI 理解论文
溯源树
样例
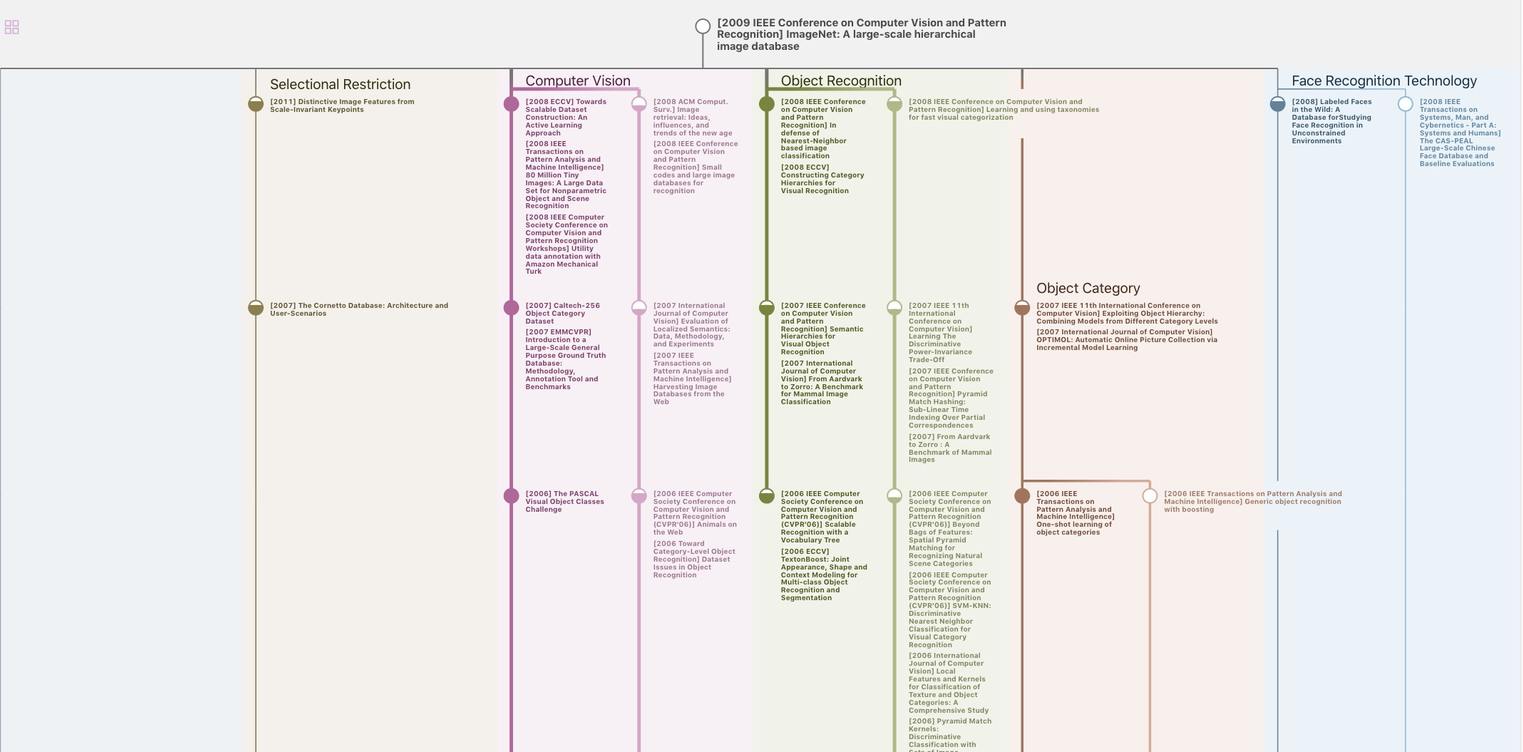
生成溯源树,研究论文发展脉络
Chat Paper
正在生成论文摘要