Co-manipulation of soft-materials estimating deformation from depth images
ROBOTICS AND COMPUTER-INTEGRATED MANUFACTURING(2024)
摘要
Human-robot manipulation of soft materials, such as fabrics, composites, and sheets of paper/cardboard, is a challenging operation that presents several relevant industrial applications. Estimating the deformation state of the manipulated material is one of the main challenges. Viable methods provide the indirect measure by calculating the human-robot relative distance. In this paper, we develop a data-driven model to estimate the deformation state of the material from a depth image through a Convolutional Neural Network (CNN). First, we define the deformation state of the material as the relative roto-translation from the current robot pose and a human grasping position. The model estimates the current deformation state through a Convolutional Neural Network, specifically, DenseNet-121 pretrained on ImageNet. The delta between the current and the desired deformation state is fed to the robot controller that outputs twist commands. The paper describes the developed approach to acquire, preprocess the dataset and train the model. The model is compared with the current state-of-the-art method based on a camera skeletal tracker. Results show that the approach achieves better performances and avoids the drawbacks of a skeletal tracker. The model was also validated over three different materials showing its generalization ability. Finally, we also studied the model performance according to different architectures and dataset dimensions to minimize the time required for dataset acquisition.
更多查看译文
关键词
Human-robot collaborative transportation,Soft materials co-manipulation,Vision-based robot manual guidance
AI 理解论文
溯源树
样例
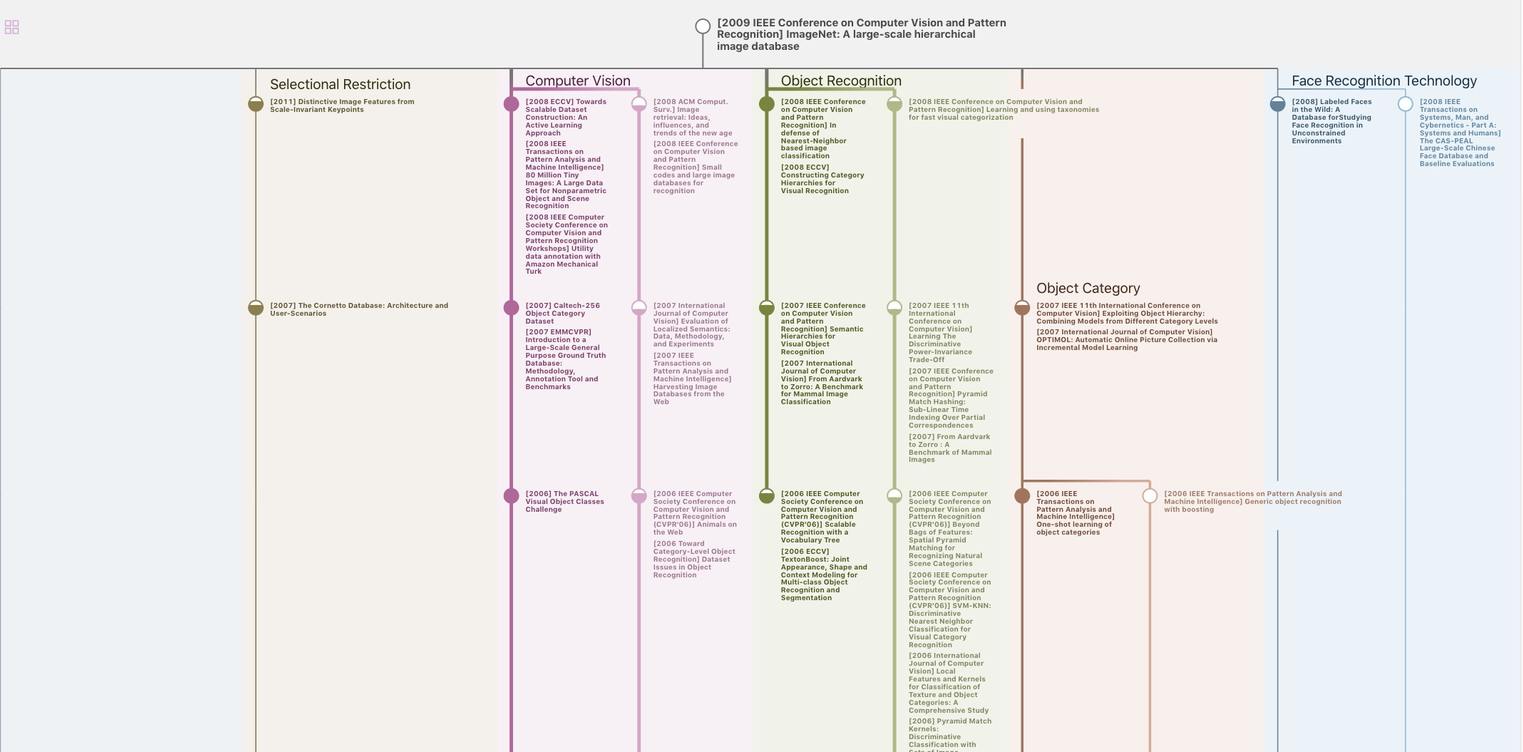
生成溯源树,研究论文发展脉络
Chat Paper
正在生成论文摘要