Risk Sensitive Dead-end Identification in Safety-Critical Offline Reinforcement Learning
arxiv(2023)
摘要
In safety-critical decision-making scenarios being able to identify worst-case outcomes, or dead-ends is crucial in order to develop safe and reliable policies in practice. These situations are typically rife with uncertainty due to unknown or stochastic characteristics of the environment as well as limited offline training data. As a result, the value of a decision at any time point should be based on the distribution of its anticipated effects. We propose a framework to identify worst-case decision points, by explicitly estimating distributions of the expected return of a decision. These estimates enable earlier indication of dead-ends in a manner that is tunable based on the risk tolerance of the designed task. We demonstrate the utility of Distributional Dead-end Discovery (DistDeD) in a toy domain as well as when assessing the risk of severely ill patients in the intensive care unit reaching a point where death is unavoidable. We find that DistDeD significantly improves over prior discovery approaches, providing indications of the risk 10 hours earlier on average as well as increasing detection by 20%.
更多查看译文
关键词
risk,offline
AI 理解论文
溯源树
样例
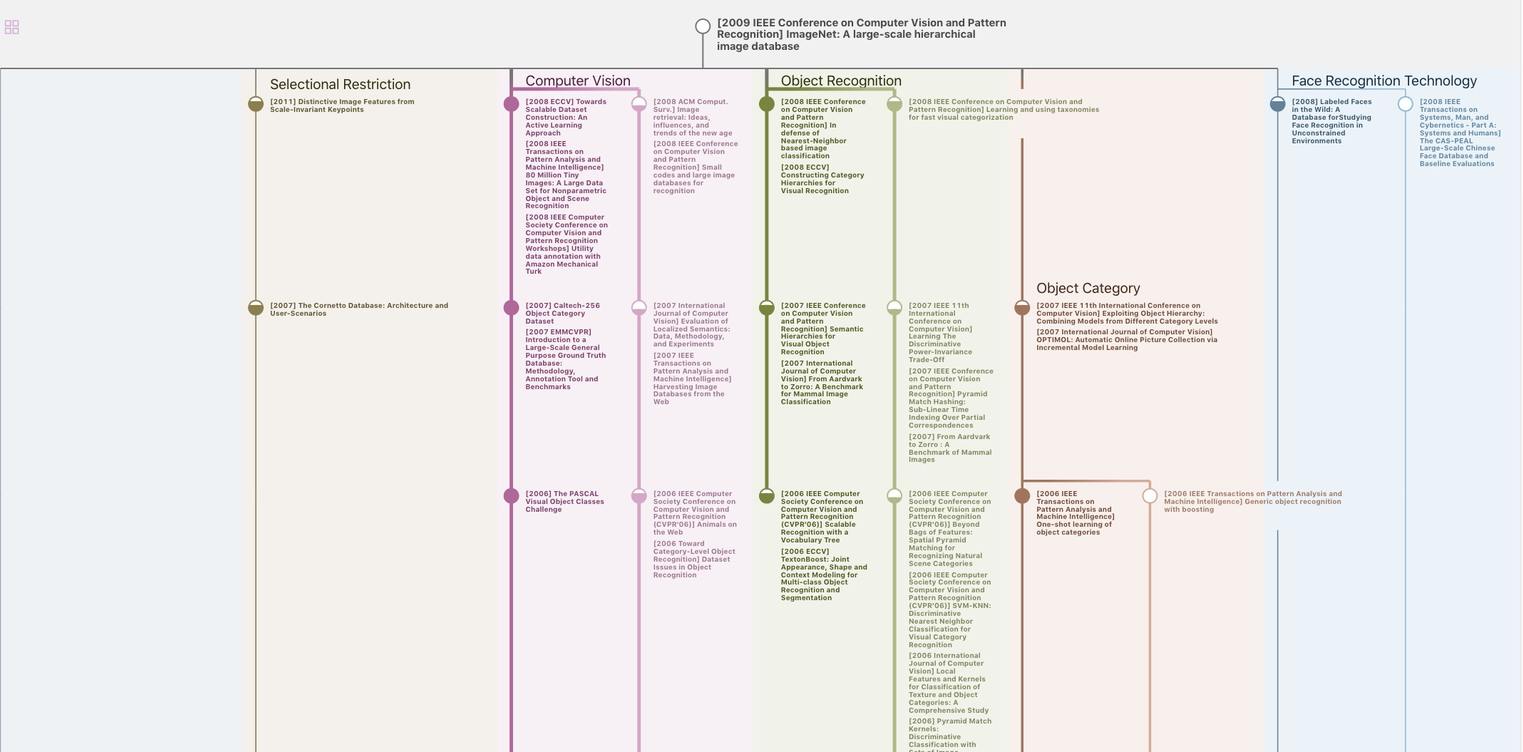
生成溯源树,研究论文发展脉络
Chat Paper
正在生成论文摘要