HRL-Edge-Cloud: Multi-Resource Allocation in Edge-Cloud based Smart-StreetScape System using Heuristic Reinforcement Learning
Information Systems Frontiers(2023)
摘要
The Edge Cloud (EC) architecture aims at providing the compute power at the edge of the network to minimize the latency necessary for the Internet of Things (IoT). However, an EC endures a limited compute capacity in contrast with the back-end cloud (BC). Intelligent resource management techniques become imperative in such resource constrained environment. In this study, to achieve the efficient resource allocation objective, we propose HRL-Edge-Cloud, a novel heuristic reinforcement learning-based multi-resource allocation (MRA) framework which significantly overcomes the bottlenecks of wireless bandwidth and compute capacity jointly at the EC and BC. We solve the MRA problem by accelerating the conventional Q-Learning algorithm with a heuristic method and applying a novel linear-annealing technique. Additionally, our proposed pruning principle achieves remarkably high resource utilization efficiency while maintaining a low rejection rate. The effectiveness of our proposed method is validated by running extensive simulations in three different scales of environments. When compared with the baseline algorithm, the proposed HRL-Edge-Cloud achieves 240X, 95X and 2.4X reduction in runtime, convergence time and rejection rate, respectively, and achieves 2.34X operational cost efficiency improvement on average while satisfying the latency requirement.
更多查看译文
关键词
Edge cloud,Heuristic reinforcement learning,Task offloading,Resource allocation,Admission control,Smart city,IoT
AI 理解论文
溯源树
样例
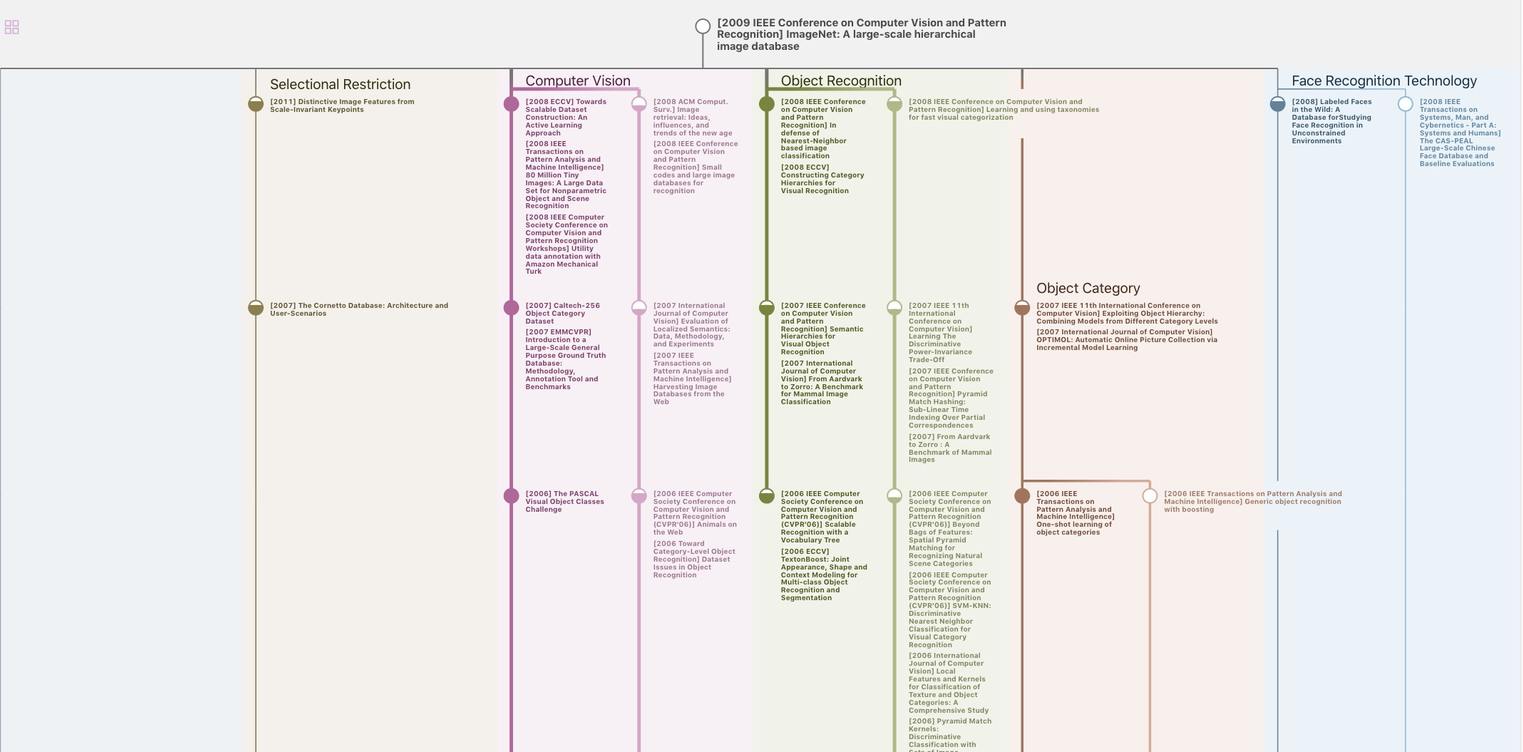
生成溯源树,研究论文发展脉络
Chat Paper
正在生成论文摘要