The Nucleotide Transformer: Building and Evaluating Robust Foundation Models for Human Genomics
biorxiv(2023)
摘要
Closing the gap between measurable genetic information and observable traits is a longstanding challenge in genomics. Yet, the prediction of molecular phenotypes from DNA sequences alone remains limited and inaccurate, often driven by the scarcity of annotated data and the inability to transfer learnings between prediction tasks. Here, we present an extensive study of foundation models pre-trained on DNA sequences, named the Nucleotide Transformer, integrating information from 3,202 diverse human genomes, as well as 850 genomes from a wide range of species, including model and non-model organisms. These transformer models yield transferable, context-specific representations of nucleotide sequences, which allow for accurate molecular phenotype prediction even in low-data settings. We show that the representations alone match or outperform specialized methods on 11 of 18 prediction tasks, and up to 15 after fine-tuning. Despite no supervision, the transformer models learnt to focus attention on key genomic elements, including those that regulate gene expression, such as enhancers. Lastly, we demonstrate that utilizing model representations alone can improve the prioritization of functional genetic variants. The training and application of foundational models in genomics explored in this study provide a widely applicable stepping stone to bridge the gap of accurate molecular phenotype prediction from DNA sequence alone.
### Competing Interest Statement
The authors have declared no competing interest.
更多查看译文
关键词
nucleotide transformer,human genomics
AI 理解论文
溯源树
样例
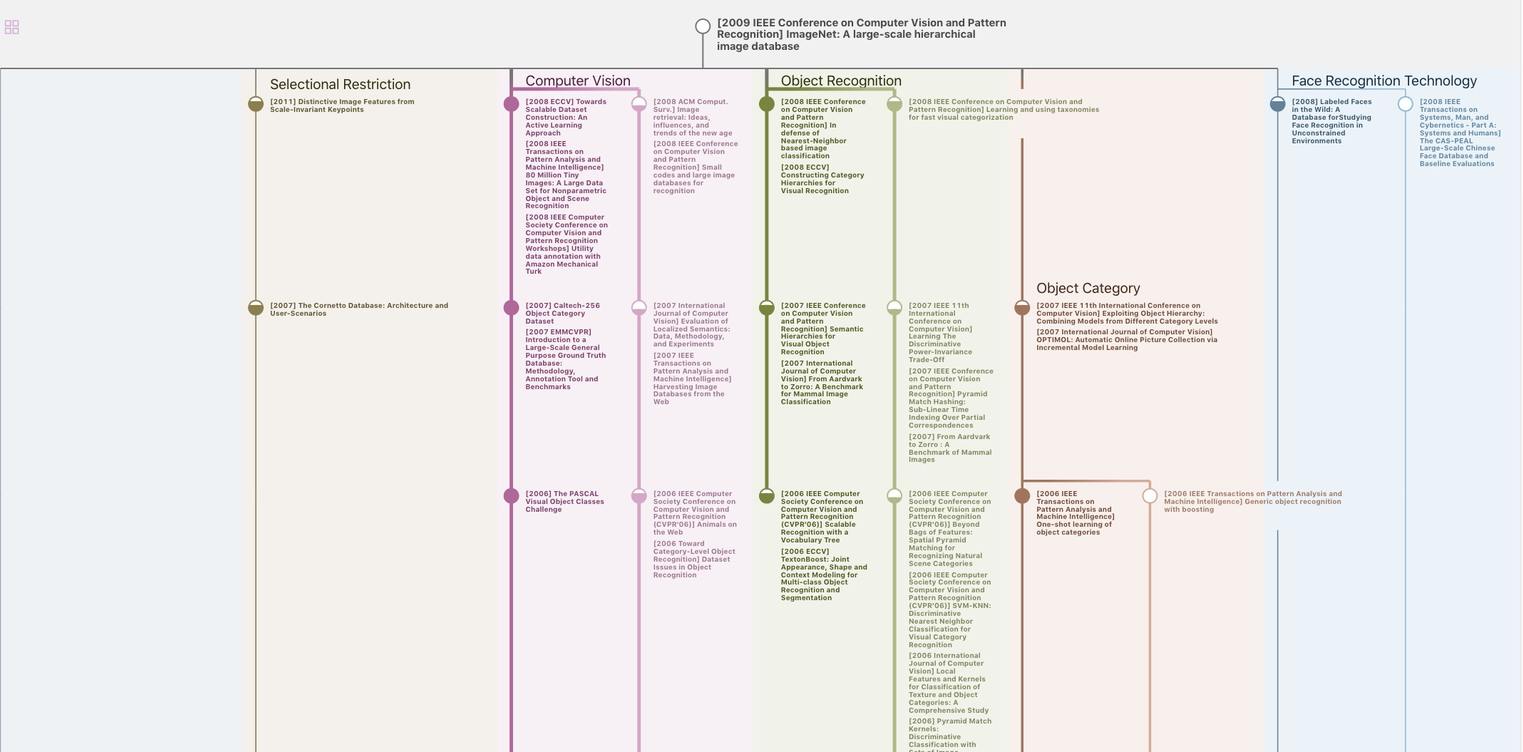
生成溯源树,研究论文发展脉络
Chat Paper
正在生成论文摘要