Physics Informed Neural Network-based High-frequency Modeling of Induction Motors
Chinese Journal of Electrical Engineering
摘要
The high-frequency (HF) modeling of induction motors plays a key role in predicting the motor terminal overvoltage and conducted emissions in a motor drive system. In this study, a physics informed neural network-based HF modeling method, which has the merits of high accuracy, good versatility, and simple parameterization, is proposed. The proposed model of the induction motor consists of a three-phase equivalent circuit with eighteen circuit elements per phase to ensure model accuracy. The per phase circuit structure is symmetric concerning its phase-start and phase-end points. This symmetry enables the proposed model to be applicable for both star- and delta-connected induction motors without having to recalculate the circuit element values when changing the motor connection from star to delta and vice versa. Motor physics knowledge, namely per-phase impedances, are used in the artificial neural network to obtain the values of the circuit elements. The parameterization can be easily implemented within a few minutes using a common personal computer (PC). Case studies verify the effectiveness of the proposed HF modeling method.
更多查看译文
关键词
Equivalent circuit,high-frequency (HF) modeling,induction motor,parameterization,physics informed neural network
AI 理解论文
溯源树
样例
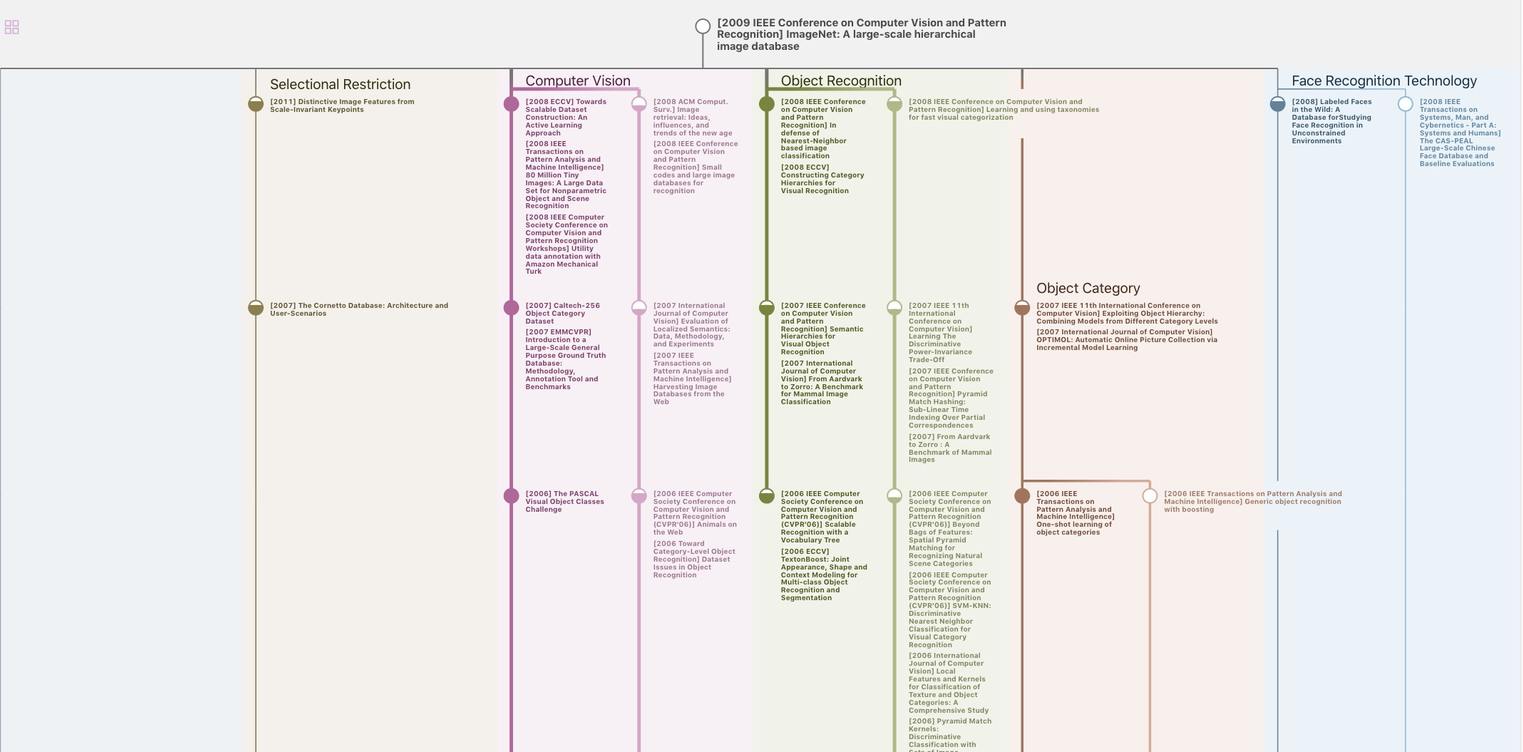
生成溯源树,研究论文发展脉络
Chat Paper
正在生成论文摘要