The impact of correlated exposures and missing data on multiple informant models used to identify critical exposure windows.
Statistics in medicine(2023)
摘要
There has been heightened interest in identifying critical windows of exposure for adverse health outcomes; that is, time points during which exposures have the greatest impact on a person's health. Multiple informant models implemented using generalized estimating equations (MIM GEEs) have been applied to address this research question because they enable statistical comparisons of differences in associations across exposure windows. As interest rises in using MIMs, the feasibility and appropriateness of their application under settings of correlated exposures and partially missing exposure measurements requires further examination. We evaluated the impact of correlation between exposure measurements and missing exposure data on the power and differences in association estimated by the MIM GEE and an inverse probability weighted extension to account for informatively missing exposures. We assessed these operating characteristics under a variety of correlation structures, sample sizes, and missing data mechanisms considering various exposure-outcome scenarios. We showed that applying MIM GEEs maintains higher power when there is a single critical window of exposure and exposure measures are not highly correlated, but may result in low power and bias under other settings. We applied these methods to a study of pregnant women living with HIV to explore differences in association between trimester-specific viral load and infant neurodevelopment.
更多查看译文
关键词
critical windows,exposure timing,generalized estimating equations,inverse probability weights,missing data,multiple informants
AI 理解论文
溯源树
样例
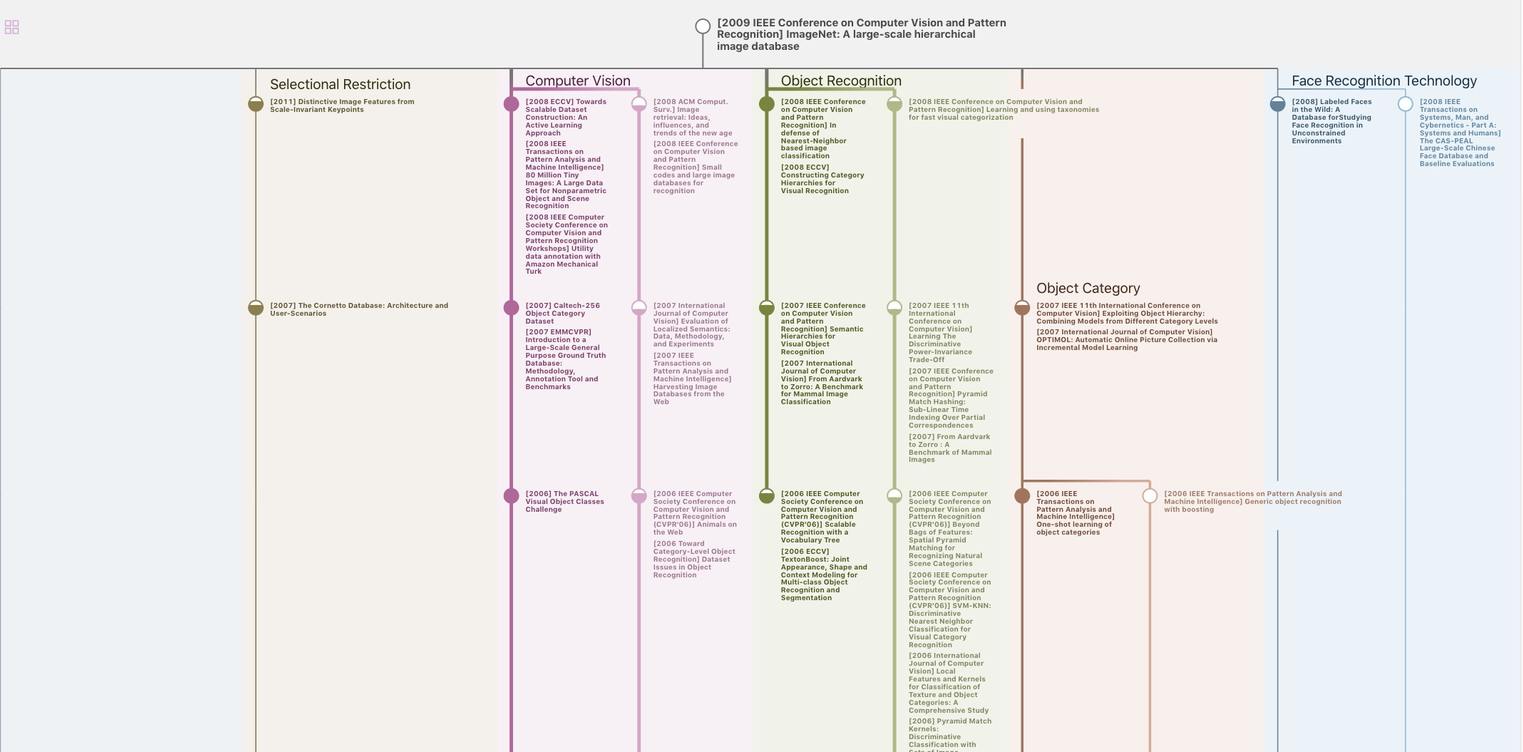
生成溯源树,研究论文发展脉络
Chat Paper
正在生成论文摘要