Finding Hidden Patterns in High Resolution Wind Flow Model Simulations
Communications in Computer and Information Science Accelerating Science and Engineering Discoveries Through Integrated Research Infrastructure for Experiment, Big Data, Modeling and Simulation(2022)
摘要
Wind flow data is critical in terms of investment decisions and policy making. High resolution data from wind flow model simulations serve as a supplement to the limited resource of original wind flow data collection. Given the large size of data, finding hidden patterns in wind flow model simulations are critical for reducing the dimensionality of the analysis. In this work, we first perform dimension reduction with two autoencoder models: the CNN-based autoencoder (CNN-AE) [1], and hierarchical autoencoder (HIER-AE) [2], and compare their performance with the Principal Component Analysis (PCA). We then investigate the super-resolution of the wind flow data. By training a Generative Adversarial Network (GAN) with 300 epochs, we obtained a trained model with 2x resolution enhancement. We compare the results of GAN with Convolutional Neural Network (CNN), and GAN results show finer structure as expected in the data field images. Also, the kinetic energy spectra comparisons show that GAN outperforms CNN in terms of reproducing the physical properties for high wavenumbers and is critical for analysis where high-wavenumber kinetics play an important role.
更多查看译文
关键词
hidden patterns,simulations,flow
AI 理解论文
溯源树
样例
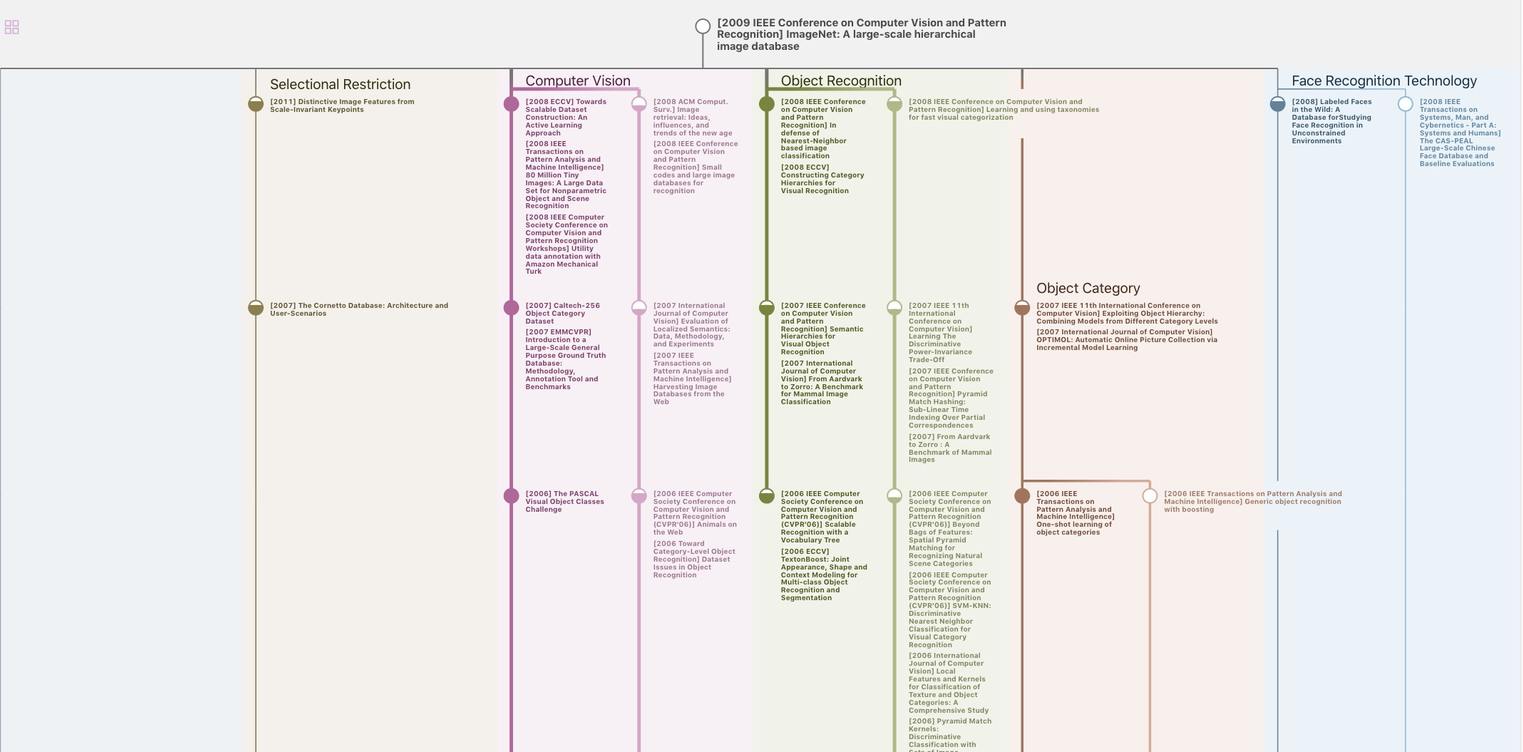
生成溯源树,研究论文发展脉络
Chat Paper
正在生成论文摘要