Human pan-body age- and sex-specific molecular phenomena inferred from public transcriptome data using machine learning
biorxiv(2023)
摘要
Age and sex are historically understudied factors in biomedical studies even though many complex traits and diseases vary by these factors in their incidence and presentation. As a result, there are massive gaps in our understanding of genes and molecular mechanisms that underlie sex- and age-associated physiology and disease. Hundreds of thousands of publicly-available human transcriptomes capturing gene expression profiles of tissues across the body and subject to various biomedical and clinical factors present an invaluable, yet untapped, opportunity for bridging these gaps. Here, we present a computational framework that leverages these data to infer genome-wide molecular signatures specific to sex and age groups. As the vast majority of these profiles lack age and sex labels, the core idea of our framework is to use the measured expression data to predict missing age/sex metadata and derive the signatures from the predictive models. We first curated ∼30,000 primary samples associated with age and sex information and profiled using microarray and RNA-seq. Then, we used this dataset to infer sex-biased genes within eleven age groups along the human lifespan and then trained machine learning (ML) models to predict these age groups from gene expression values separately within females and males. Specifically, we trained one-vs-rest logistic regression classifiers with elastic-net regularization to classify transcriptomes into age groups. Dataset-level cross validation shows that these ML classifiers are able to discriminate between age groups in a biologically meaningful way in each sex across technologies. Further, these predictive models capture sex-stratified age-group ‘gene signatures’, i.e., the strength and the direction of importance of genes across the genome for each age group in each sex. Enrichment analysis of these gene signatures with prior gene annotations helped in identifying age- and sex-associated multi-tissue and pan-body molecular phenomena (e.g., general immune response, inflammation, metabolism, hormone response). We developed a web-app ( ) to visualize our expression dataset, signatures, and enrichment results to make these easily accessible for interested researchers. Overall, we have presented a path for effectively leveraging massive public omics data collections to investigate the molecular basis of age- and sex-differences in physiology and disease.
Summary Hundreds of thousands of publicly-available human transcriptomes capturing gene expression profiles of tissues across the body and subject to various biomedical and clinical factors present an invaluable, yet untapped, opportunity for studying age and sex. We first curated ∼30,000 primary microarray and RNA-seq samples. Then, we used this dataset to infer sex-biased genes within eleven age groups along the human lifespan and trained machine learning models to predict these age groups from gene expression values separately within females and males. These predictive models capture sex-stratified age-group ‘gene signatures’, i.e., the strength and the direction of importance of every gene in each age group in each sex. Enrichment analysis of these gene signatures with prior gene annotations helped identify age- and sex-associated multi-tissue molecular phenomena. A web-app makes our dataset and results easily visualizable. Overall, we have presented a path for effectively leveraging massive public omics data collections to investigate the molecular basis of age- and sex-differences in physiology and disease.
### Competing Interest Statement
The authors have declared no competing interest.
更多查看译文
AI 理解论文
溯源树
样例
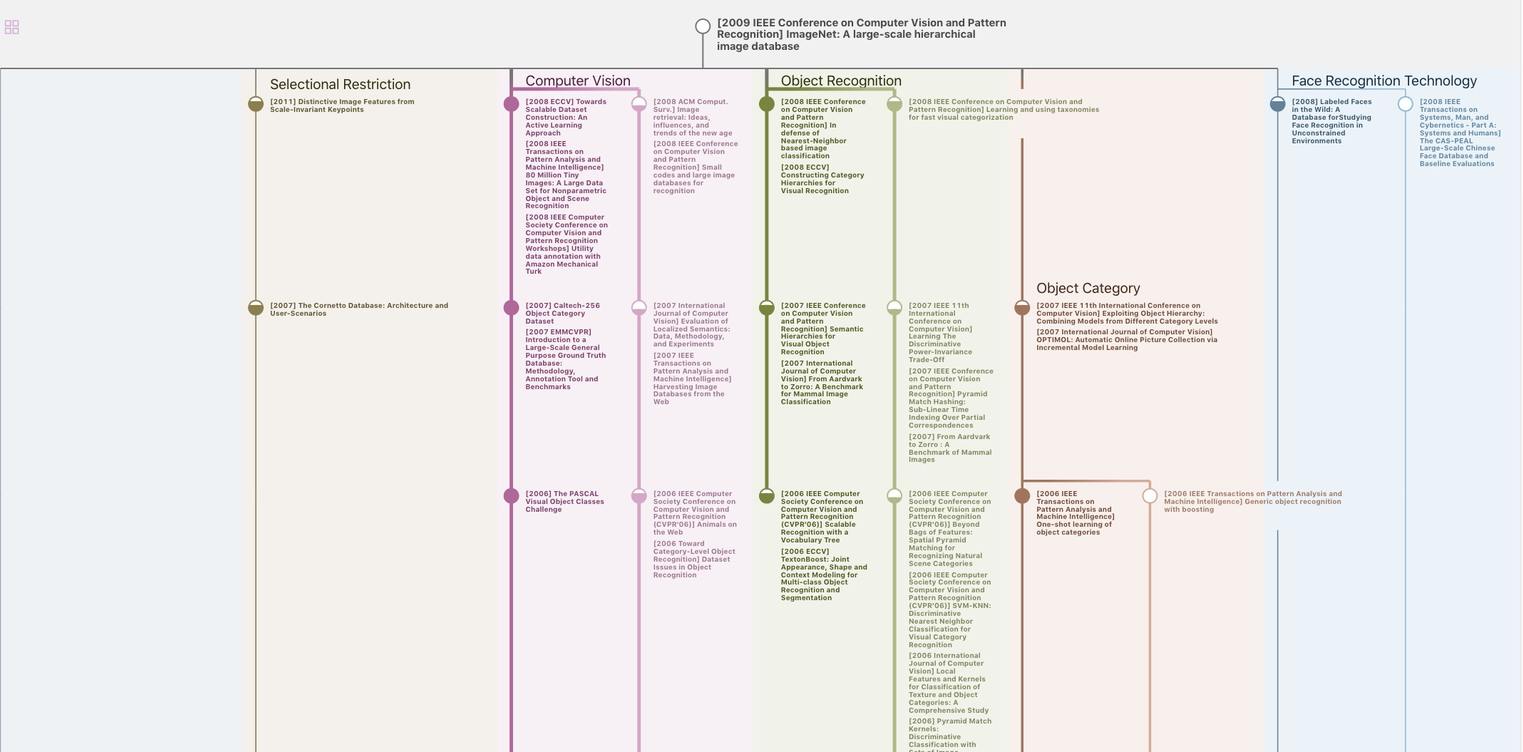
生成溯源树,研究论文发展脉络
Chat Paper
正在生成论文摘要