Comparing risk prediction models aimed at predicting hospitalizations for adverse drug events in community dwelling older adults: a protocol paper.
Research Square (Research Square)(2023)
摘要
Background:The objective of this paper is to describe the creation, validation, and comparison of two risk prediction modeling approaches for community-dwelling older adults to identify individuals at highest risk for adverse drug event-related hospitalizations. One approach will use traditional statistical methods, the second will use a machine learning approach.
Methods:We will construct medication, clinical, health care utilization, and other variables known to be associated with adverse drug event-related hospitalizations. To create the cohort, we will include older adults (≥ 65 years of age) empaneled to a primary care physician within the Cedars-Sinai Health System primary care clinics with polypharmacy (≥ 5 medications) or at least 1 medication commonly implicated in ADEs (certain oral hypoglycemics, anti-coagulants, anti-platelets, and insulins). We will use a Fine-Gray Cox proportional hazards model for one risk modeling approach and DataRobot, a data science and analytics platform, to run and compare several widely used supervised machine learning algorithms, including Random Forest, Support Vector Machine, Extreme Gradient Boosting (XGBoost), Decision Tree, Naïve Bayes, and K-Nearest Neighbors. We will use a variety of metrics to compare model performance and to assess the risk of algorithmic bias.
Discussion:In conclusion, we hope to develop a pragmatic model that can be implemented in the primary care setting to risk stratify older adults to further optimize medication management.
更多查看译文
关键词
adverse drug events,risk prediction models,hospitalizations
AI 理解论文
溯源树
样例
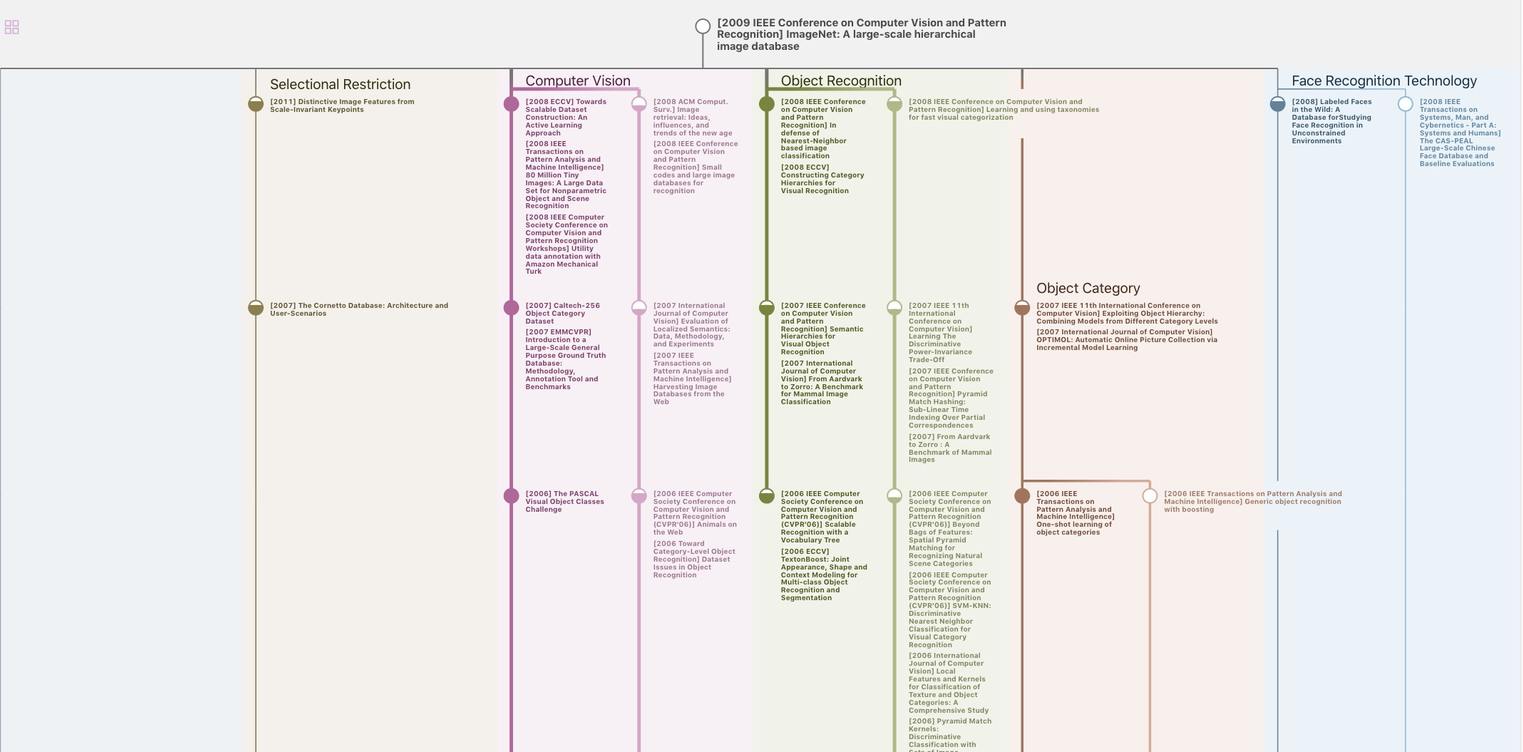
生成溯源树,研究论文发展脉络
Chat Paper
正在生成论文摘要