KOMPUTE: Imputing summary statistics of missing phenotypes in high-throughput model organism data
biorxiv(2023)
摘要
Motivation The International Mouse Phenotyping Consortium (IMPC) is striving to build a comprehensive functional catalog of mammalian protein-coding genes by systematically producing and phenotyping gene-knockout mice for almost every protein-coding gene in the mouse genome and by testing associations between gene loss-of-function and phenotype. To date, the IMPC has identified over 90,000 gene-phenotype associations, but many phenotypes have not yet been measured for each gene, resulting in largely incomplete data; about 75.6% of association summary statistics are still missing in the latest IMPC summary statistics dataset (IMPC release version 16).
Results To overcome these challenges, we propose KOMPUTE, a novel method for imputing missing summary statistics in the IMPC dataset. Using conditional distribution properties of multivariate normal, KOMPUTE estimates association Z-scores of unmeasured phenotypes for a particular gene as a conditional expectation given the Z-scores of measured phenotypes. We evaluate the efficacy of the proposed method for recovering missing Z-scores using simulated and real-world data sets and compare it to a singular value decomposition (SVD) matrix completion method. Our results show that KOMPUTE outperforms the comparison method across different scenarios.
Availability and implementation An R package for KOMPUTE is publicly available at , along with usage examples and results for different phenotype domains at .
Contact leed13{at}miamioh.edu
Supplementary information Supplementary data are available at Bioinformatics online.
### Competing Interest Statement
The authors have declared no competing interest.
更多查看译文
关键词
phenotypes,summary statistics,high-throughput
AI 理解论文
溯源树
样例
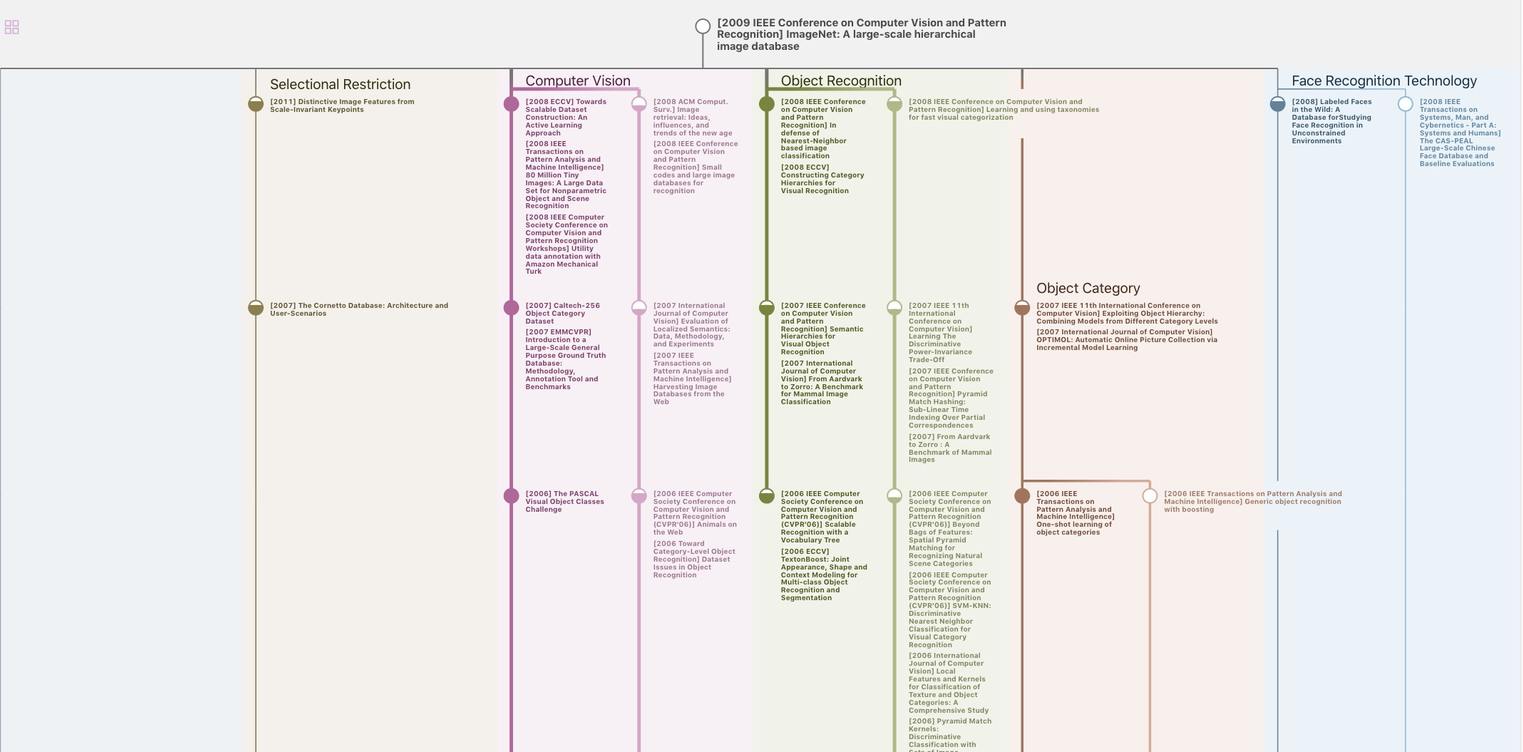
生成溯源树,研究论文发展脉络
Chat Paper
正在生成论文摘要