Summative Student Course Review Tool Based on Machine Learning Sentiment Analysis to Enhance Life Science Feedback Efficacy
arxiv(2023)
摘要
Machine learning enables the development of new, supplemental, and empowering tools that can either expand existing technologies or invent new ones. In education, space exists for a tool that supports generic student course review formats to organize and recapitulate students' views on the pedagogical practices to which they are exposed. Often, student opinions are gathered with a general comment section that solicits their feelings towards their courses without polling specifics about course contents. Herein, we show a novel approach to summarizing and organizing students' opinions via analyzing their sentiment towards a course as a function of the language/vocabulary used to convey their opinions about a class and its contents. This analysis is derived from their responses to a general comment section encountered at the end of post-course review surveys. This analysis, accomplished with Python, LaTeX, and Google's Natural Language API, allows for the conversion of unstructured text data into both general and topic-specific sub-reports that convey students' views in a unique, novel way.
更多查看译文
关键词
life science feedback efficacy,machine learning sentiment
AI 理解论文
溯源树
样例
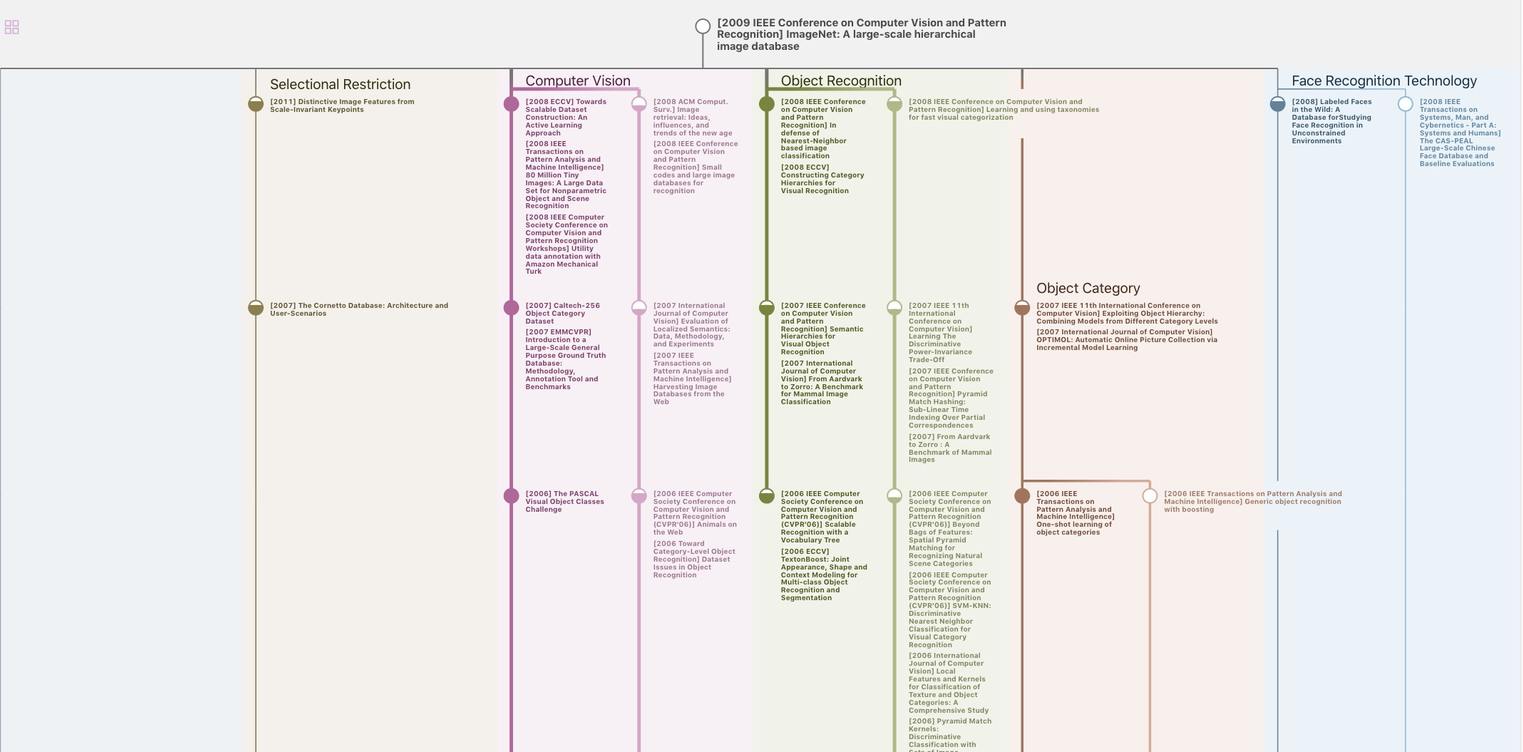
生成溯源树,研究论文发展脉络
Chat Paper
正在生成论文摘要