Near-Optimal Estimation of Linear Functionals with Log-Concave Observation Errors
arxiv(2023)
摘要
This note addresses the question of optimally estimating a linear functional of an object acquired through linear observations corrupted by random noise, where optimality pertains to a worst-case setting tied to a symmetric, convex, and closed model set containing the object. It complements the article "Statistical Estimation and Optimal Recovery" published in the Annals of Statistics in 1994. There, Donoho showed (among other things) that, for Gaussian noise, linear maps provide near-optimal estimation schemes relatively to a performance measure relevant in Statistical Estimation. Here, we advocate for a different performance measure arguably more relevant in Optimal Recovery. We show that, relatively to this new measure, linear maps still provide near-optimal estimation schemes even if the noise is merely log-concave. Our arguments, which make a connection to the deterministic noise situation and bypass properties specific to the Gaussian case, offer an alternative to parts of Donoho's proof.
更多查看译文
关键词
linear functionals,estimation,near-optimal,log-concave
AI 理解论文
溯源树
样例
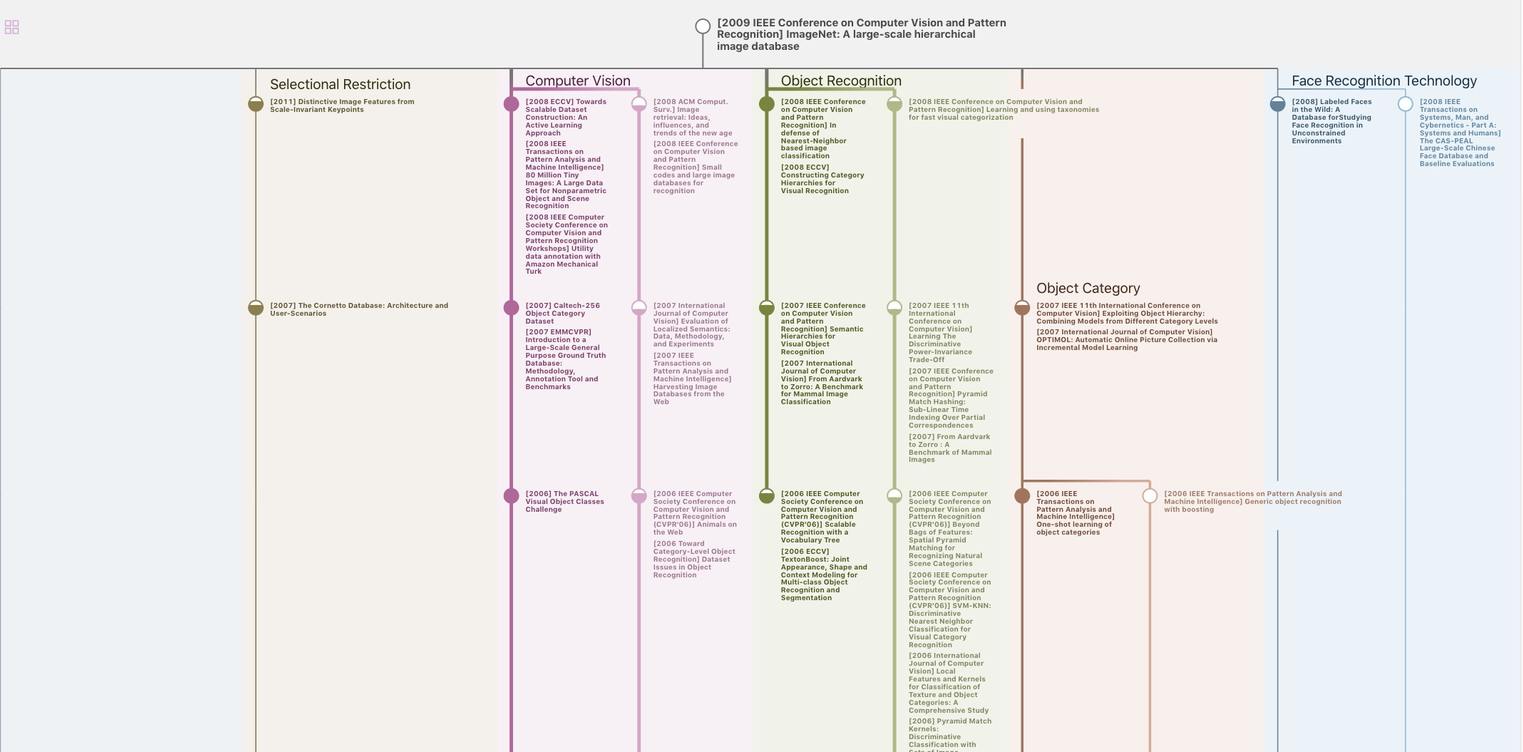
生成溯源树,研究论文发展脉络
Chat Paper
正在生成论文摘要