Adaptively Integrated Knowledge Distillation and Prediction Uncertainty for Continual Learning
arXiv (Cornell University)(2023)
摘要
Current deep learning models often suffer from catastrophic forgetting of old knowledge when continually learning new knowledge. Existing strategies to alleviate this issue often fix the trade-off between keeping old knowledge (stability) and learning new knowledge (plasticity). However, the stability-plasticity trade-off during continual learning may need to be dynamically changed for better model performance. In this paper, we propose two novel ways to adaptively balance model stability and plasticity. The first one is to adaptively integrate multiple levels of old knowledge and transfer it to each block level in the new model. The second one uses prediction uncertainty of old knowledge to naturally tune the importance of learning new knowledge during model training. To our best knowledge, this is the first time to connect model prediction uncertainty and knowledge distillation for continual learning. In addition, this paper applies a modified CutMix particularly to augment the data for old knowledge, further alleviating the catastrophic forgetting issue. Extensive evaluations on the CIFAR100 and the ImageNet datasets confirmed the effectiveness of the proposed method for continual learning.
更多查看译文
关键词
Meta-Learning,Representation Learning,Transfer Learning
AI 理解论文
溯源树
样例
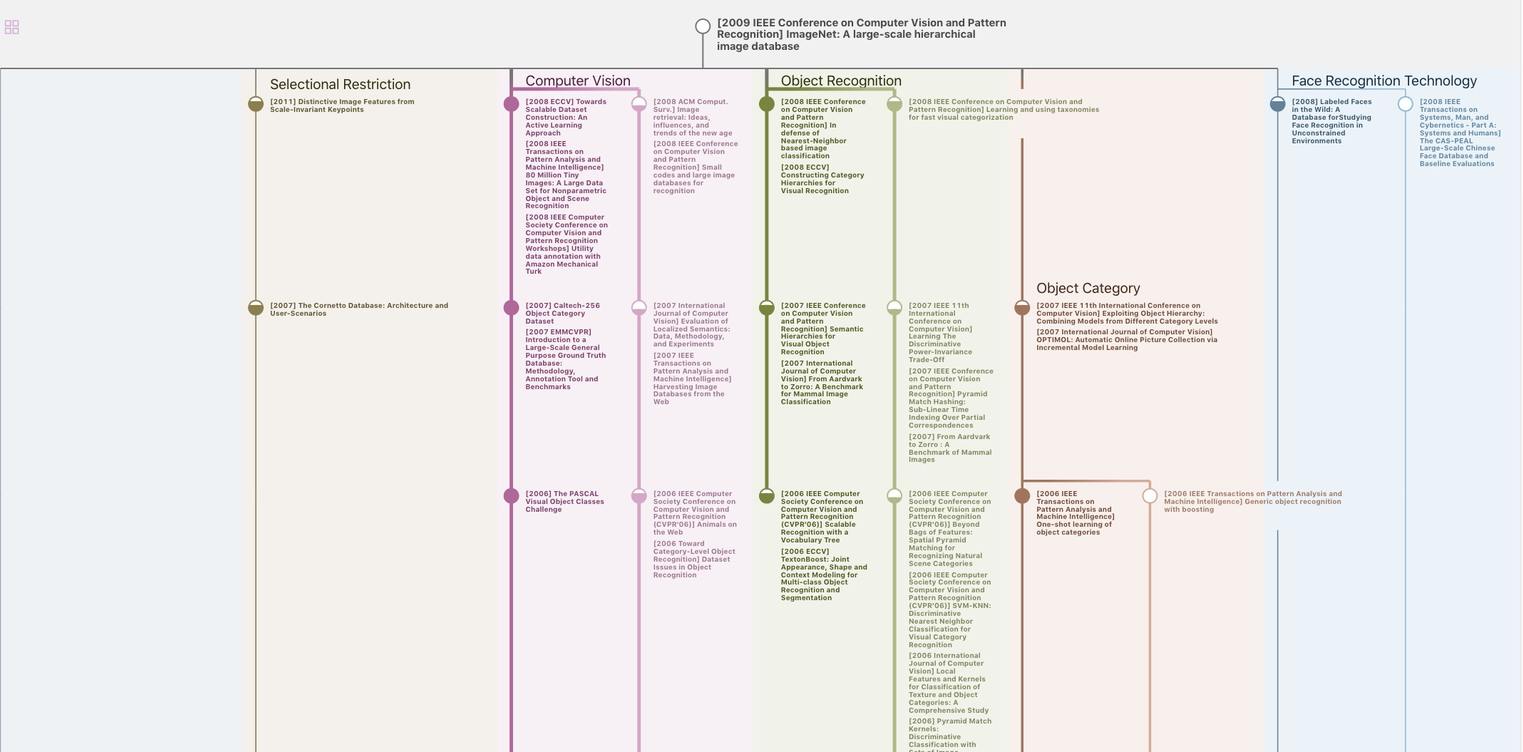
生成溯源树,研究论文发展脉络
Chat Paper
正在生成论文摘要