Discrete Latent Structure in Neural Networks
CoRR(2023)
Abstract
Many types of data from fields including natural language processing, computer vision, and bioinformatics, are well represented by discrete, compositional structures such as trees, sequences, or matchings. Latent structure models are a powerful tool for learning to extract such representations, offering a way to incorporate structural bias, discover insight about the data, and interpret decisions. However, effective training is challenging, as neural networks are typically designed for continuous computation. This text explores three broad strategies for learning with discrete latent structure: continuous relaxation, surrogate gradients, and probabilistic estimation. Our presentation relies on consistent notations for a wide range of models. As such, we reveal many new connections between latent structure learning strategies, showing how most consist of the same small set of fundamental building blocks, but use them differently, leading to substantially different applicability and properties.
MoreTranslated text
Key words
neural networks,structure
AI Read Science
Must-Reading Tree
Example
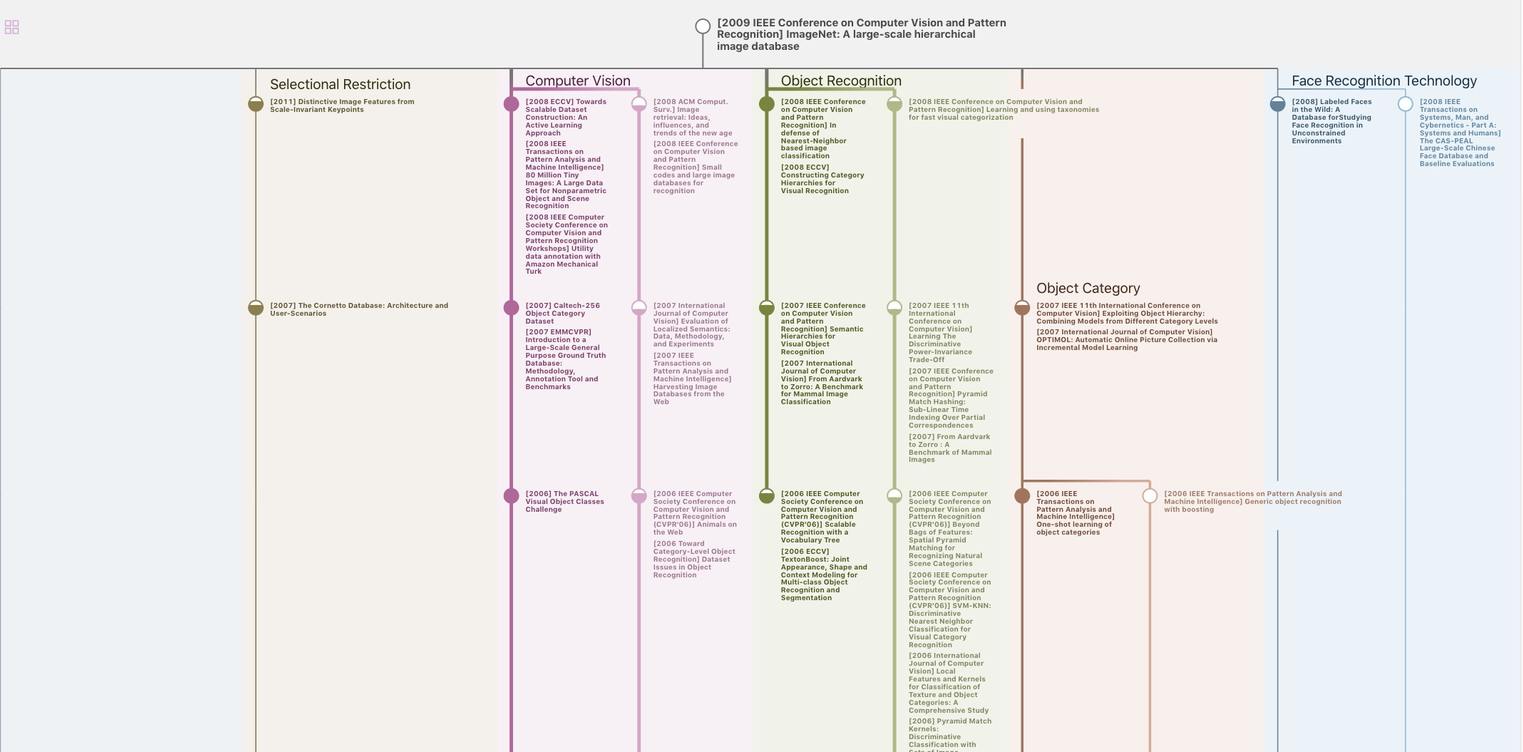
Generate MRT to find the research sequence of this paper
Chat Paper
Summary is being generated by the instructions you defined