Curvilinear object segmentation in medical images based on ODoS filter and deep learning network
CoRR(2023)
摘要
Automatic segmentation of curvilinear objects in medical images plays an important role in the diagnosis and evaluation of human diseases, yet it is a challenging uncertainty in the complex segmentation tasks due to different issues such as various image appearances, low contrast between curvilinear objects and their surrounding backgrounds, thin and uneven curvilinear structures, and improper background illumination conditions. To overcome these challenges, we present a unique curvilinear structure segmentation framework based on an oriented derivative of stick (ODoS) filter and a deep learning network for curvilinear object segmentation in medical images. Currently, a large number of deep learning models emphasize developing deep architectures and ignore capturing the structural features of curvilinear objects, which may lead to unsatisfactory results.In consequence, a new approach that incorporates an ODoS filter as part of a deep learning network is presented to improve the spatial attention of curvilinear objects.Specifically, the input image is transferred into four-channel image constructed by the ODoS filter. In which, the original image is considered the principal part to describe various image appearance and complex background illumination conditions, a multi-step strategy is used to enhance the contrast between curvilinear objects and their surrounding backgrounds, and a vector field is applied to discriminate thin and uneven curvilinear structures. Subsequently, a deep learning framework is employed to extract various structural features for curvilinear object segmentation in medical images.Which can effectively capture the spatial attention of curvature objects. The performance of the computational model is validated in experiments conducted on the publicly available DRIVE, STARE and CHASEDB1 datasets. Compared with ground truths, the presented model acquired high F1 values of 0.826, 0.819 and 0.808, and high accuracy of 0.969, 0.973, and 0.973 on three different datasets, respectively. The experimental results indicate that the presented model yields surprising results compared with those of some state-of-the-art methods.
更多查看译文
关键词
Curvilinear object segmentation,Medical images,Deep learning network,An oriented derivative of stick filter
AI 理解论文
溯源树
样例
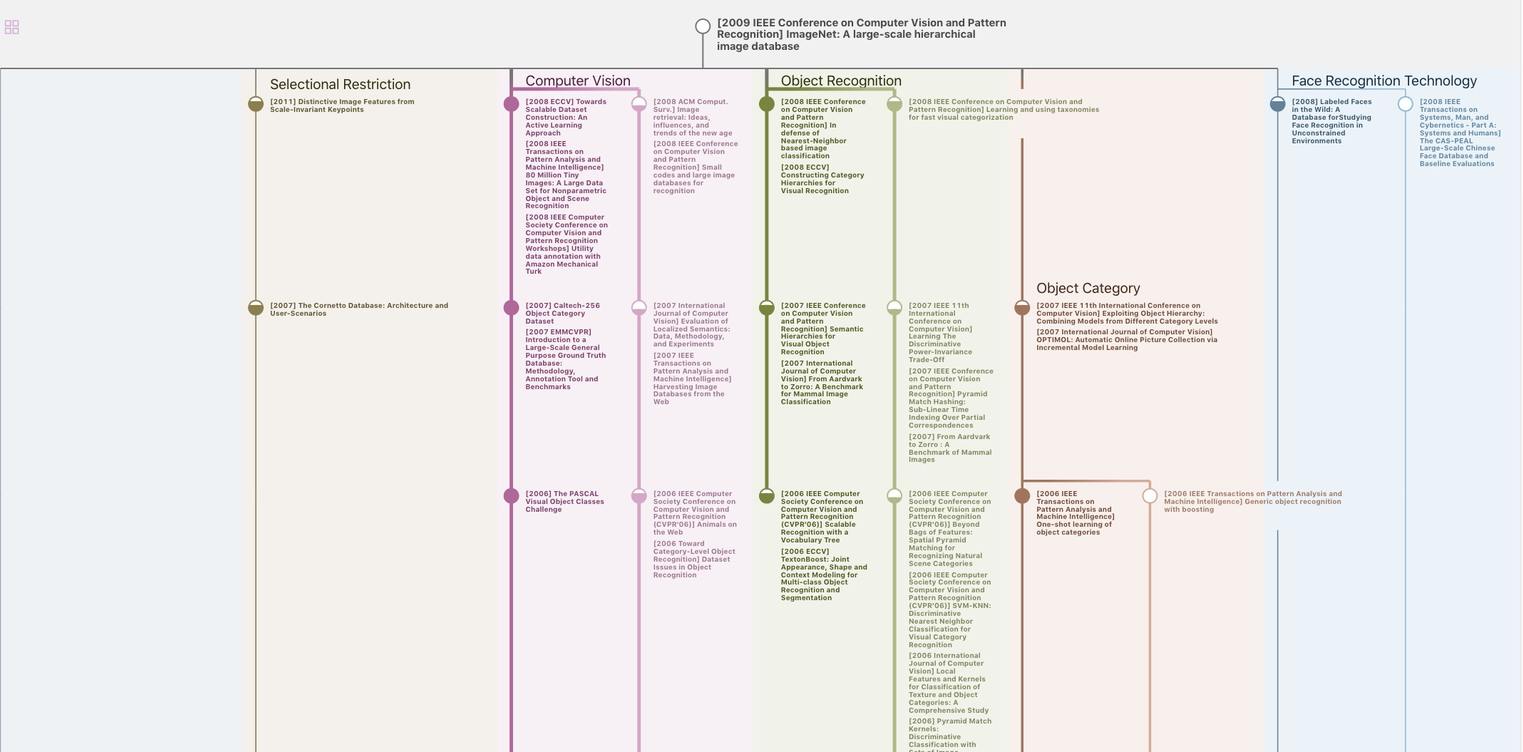
生成溯源树,研究论文发展脉络
Chat Paper
正在生成论文摘要