A data integration framework for spatial interpolation of temperature observations using climate model data.
PeerJ(2023)
摘要
Meteorological station measurements are an important source of information for understanding the weather and its association with risk, and are vital in quantifying climate change. However, such data tend to lack spatial coverage and are often plagued with flaws such as erroneous outliers and missing values. Alternative meteorological data exist in the form of climate model output that have better spatial coverage, at the expense of bias. We propose a probabilistic framework to integrate temperature measurements with climate model (reanalysis) data, in a way that allows for biases and erroneous outliers, while enabling prediction at any spatial resolution. The approach is Bayesian which facilitates uncertainty quantification and simulation based inference, as illustrated by application to two countries from the Middle East and North Africa region, an important climate change hotspot. We demonstrate the use of the model in: identifying outliers, imputing missing values, non-linear bias correction, downscaling and aggregation to any given spatial configuration.
更多查看译文
关键词
Bayesian models,Bias correction,Data blending,Outliers,Penalised splines,Spatial extrapolation,Statistical downscaling
AI 理解论文
溯源树
样例
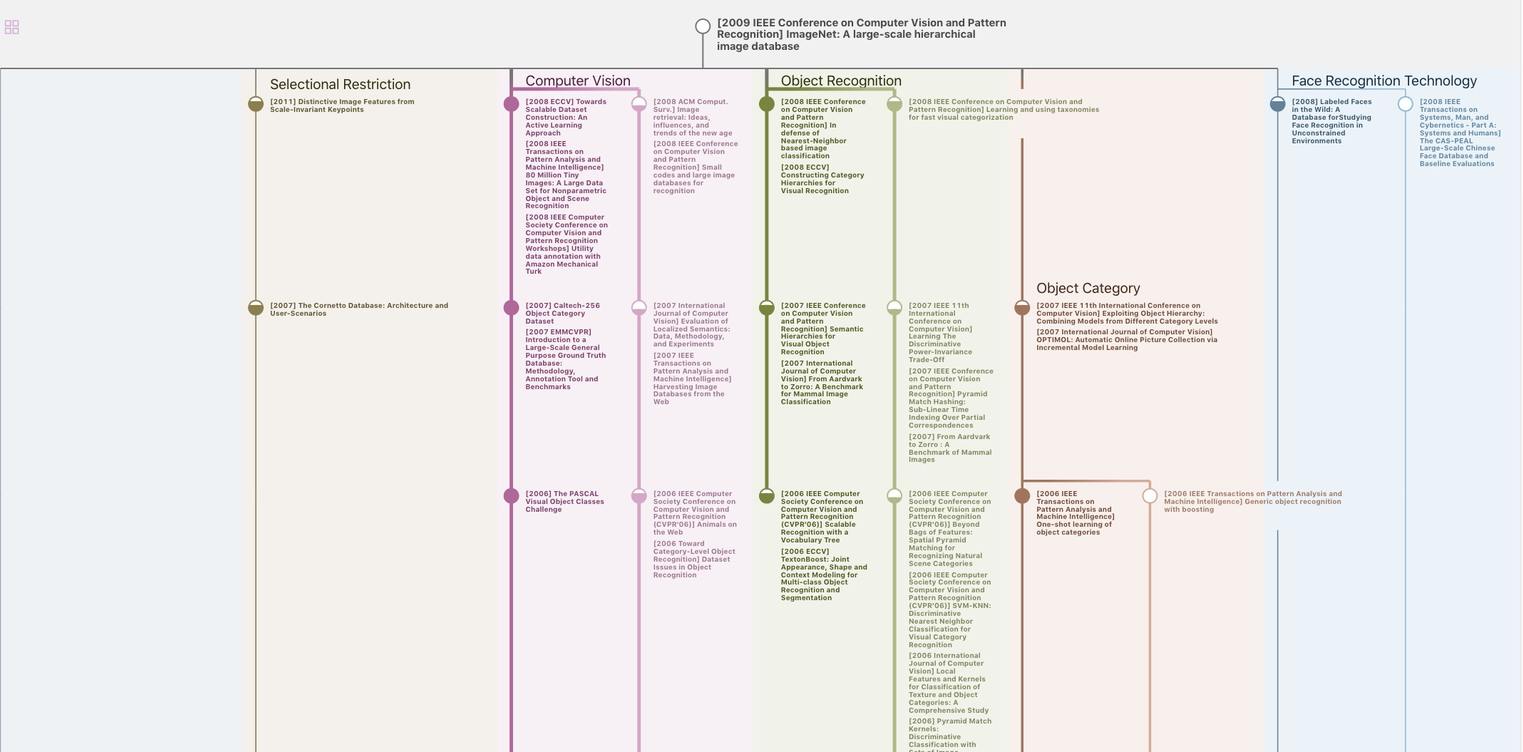
生成溯源树,研究论文发展脉络
Chat Paper
正在生成论文摘要