Hierarchical Reinforcement Learning Based Traffic Steering in Multi-RAT 5G Deployments
arxiv(2023)
摘要
In 5G non-standalone mode, an intelligent traffic steering mechanism can vastly aid in ensuring smooth user experience by selecting the best radio access technology (RAT) from a multi-RAT environment for a specific traffic flow. In this paper, we propose a novel load-aware traffic steering algorithm based on hierarchical reinforcement learning (HRL) while satisfying diverse QoS requirements of different traffic types. HRL can significantly increase system performance using a bi-level architecture having a meta-controller and a controller. In our proposed method, the meta-controller provides an appropriate threshold for load balancing, while the controller performs traffic admission to an appropriate RAT in the lower level. Simulation results show that HRL outperforms a Deep Q-Learning (DQN) and a threshold-based heuristic baseline with 8.49%, 12.52% higher average system throughput and 27.74%, 39.13% lower network delay, respectively.
更多查看译文
关键词
Multi-RAT,traffic steering,hierarchical reinforcement learning
AI 理解论文
溯源树
样例
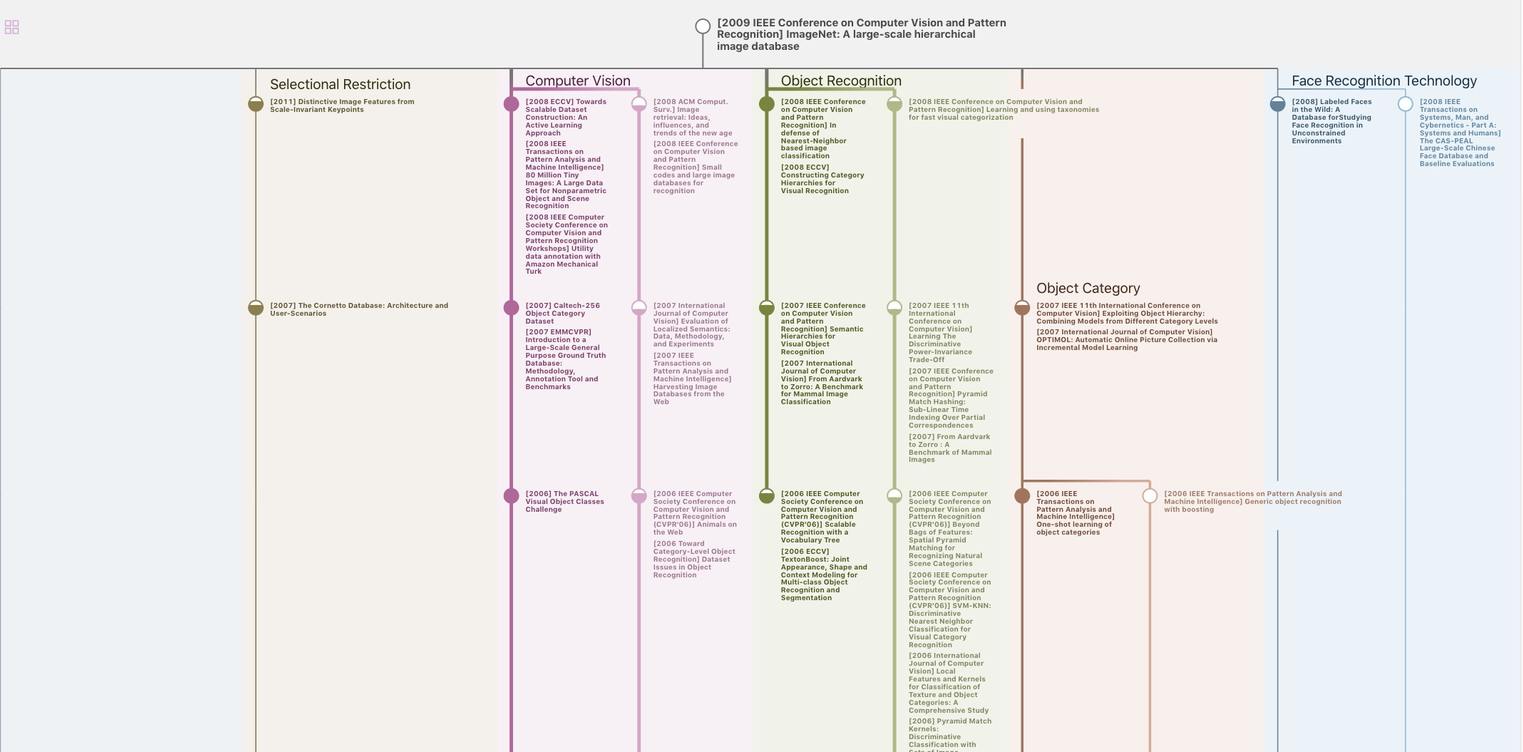
生成溯源树,研究论文发展脉络
Chat Paper
正在生成论文摘要