Machine-learning assisted scheduling optimization and its application in quantum chemical calculations.
Journal of computational chemistry(2023)
摘要
Easy and effective usage of computational resources is crucial for scientific calculations, both from the perspectives of timeliness and economic efficiency. This work proposes a bi-level optimization framework to optimize the computational sequences. Machine-learning (ML) assisted static load-balancing, and different dynamic load-balancing algorithms can be integrated. Consequently, the computational and scheduling engine of the ParaEngine is developed to invoke optimized quantum chemical (QC) calculations. Illustrated benchmark calculations include high-throughput drug suit, solvent model, P38 protein, and SARS-CoV-2 systems. The results show that the usage rate of given computational resources for high throughput and large-scale fragmentation QC calculations can primarily profit, and faster accomplishing computational tasks can be expected when employing high-performance computing (HPC) clusters.
更多查看译文
关键词
distributed computing,fragmentation approach,high throughput computing,interaction energy calculations,load-balancing
AI 理解论文
溯源树
样例
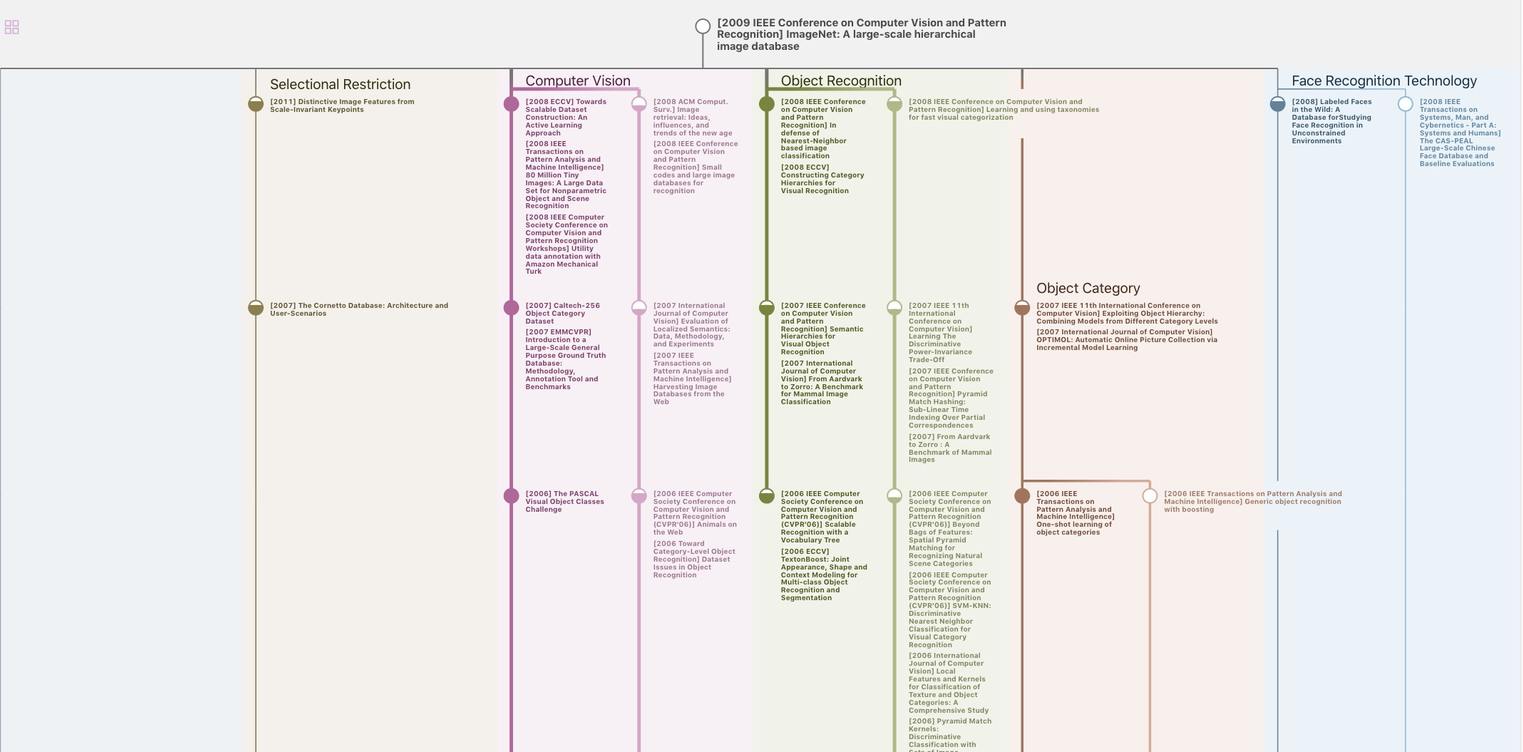
生成溯源树,研究论文发展脉络
Chat Paper
正在生成论文摘要