A Novel and Efficient Sequential Learning-Based Malware Classification Model
2022 19th International Computer Conference on Wavelet Active Media Technology and Information Processing (ICCWAMTIP)(2022)
摘要
The proliferation and increasing sophistication of malicious programs and other security risks have been identified as the most significant problem of the modern cybersecurity age. Malicious software, named malware, carries out harmful operations, causing abnormal functioning, data leakage, and crippling financial effects. Therefore, the door is widely open for one of the most crucial realms, so-called deep learning-based malware classification techniques, to thwart malware attacks. Malware's structure evolves significantly over time, making detecting them challenging. Malware invokes API call sequences while executing, so call sequences from APIs make excellent candidates for features in malware classification. Different malware samples can contain API call sequences with lengths ranging from one to millions, which can raise computation costs and complicate processing times; selecting an efficient set of features is yet another challenge. Recurrent neural networks (RNNs) are one of the most adaptable techniques for handling time-series data and are used to classify malware based on API calls. To efficiently categorize malware, in this study, a novel and efficient Long Short Term Memory model has been designed and divided into eight categories: Adware, Backdoor, Downloader, Dropper, spyware, Trojan, Virus, and Worm. The achieved results in terms of recall, precision, and F1 values are notable across several classes, whereas the adware class has the most significant result with a recall value of 80%. The proposed LSTM-based method outperformed conventional methods with a weighted F1 score of 48%.
更多查看译文
关键词
Malware classification,API call sequence,Recurrent neural network,Long Short-Term Memory
AI 理解论文
溯源树
样例
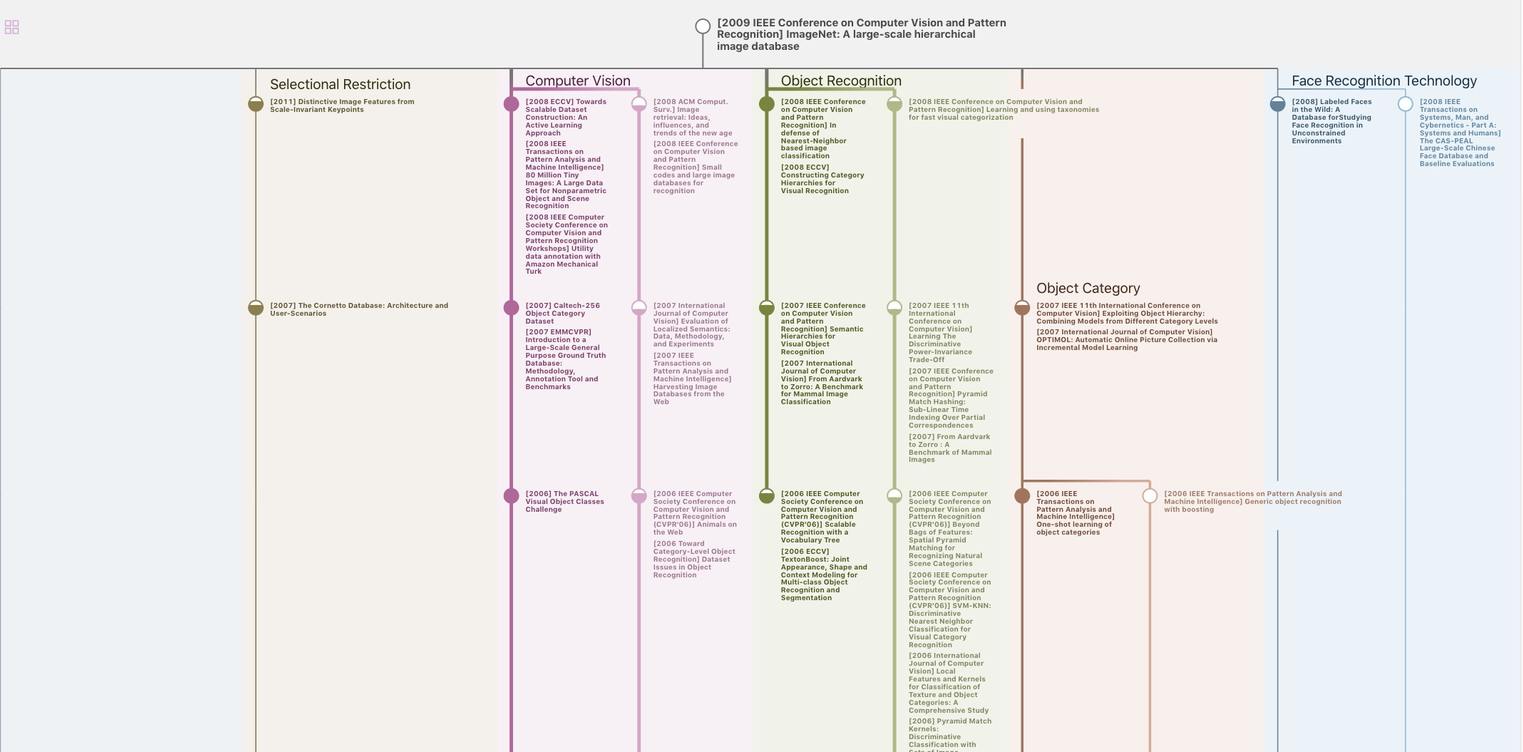
生成溯源树,研究论文发展脉络
Chat Paper
正在生成论文摘要