Robust graph embedding via self-supervised graph denoising
2022 19th International Computer Conference on Wavelet Active Media Technology and Information Processing (ICCWAMTIP)(2022)
摘要
Graph embedding has attracted increasing attention in the past few years. Graph neural network is the most popular type of algorithm to provide high-quality graph embedding. It brings stunning success but causes an important and easy-to-be-ignored problem, i.e., vulnerability, which makes the embedding quality dramatically degrade and affects the performance of downstream tasks like node classification. In this paper, we propose an algorithm for robust graph embedding via self-supervised graph denoising (SSGD). The key idea is to learn normal patterns about how a graph is organized and apply the patterns to reorganize the structure and remove noisy edges in the graph. Since the vulnerability is mainly caused by noisy edges, graph neural networks are supposed to work well on denoised graphs. In experiments, we introduce 6 state-of-the-art algorithms and 3 real-world datasets to demonstrate the superiority of our algorithm.
更多查看译文
关键词
Graph embedding,Robustness,Self-supervised learning
AI 理解论文
溯源树
样例
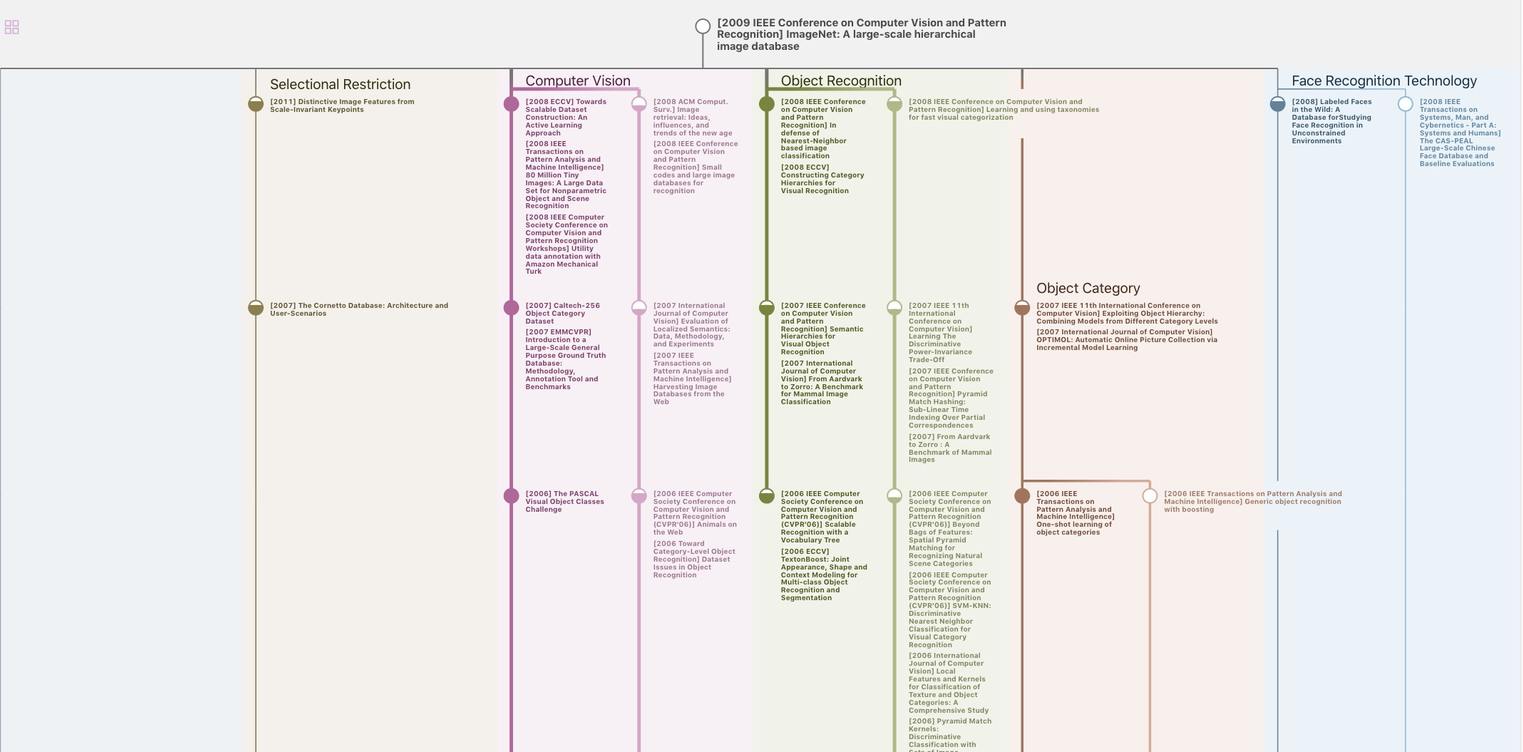
生成溯源树,研究论文发展脉络
Chat Paper
正在生成论文摘要