Assessment of Carbon-Aware Flexibility Measures From Data Centres Using Machine Learning
IEEE Transactions on Industry Applications(2023)
摘要
Potential solutions for unlocking operational flexibility from data centres are investigated. Two types of data centres, in terms of their cooling facilities, are modelled in order to provide an in-depth assessment of potential variations in data centre performance based on their energy consumption and server temperatures. Using synthetic datasets generated from year-long building dynamic simulations under distinct system conditions, four machine learning (ML) models are designed and trained to predict data centre energy consumption and server temperatures. Three different ML algorithms are considered, including random forest, XGBOOST, and multiple linear regression, which all achieve high accuracy ranging from 93.8% to 98.1% for the mean absolute percentage error. The resulting ML models are then utilised to represent a system-wide fleet of data centres, which are integrated within a power system unit scheduling framework for potential demand shifting based on (system) carbon intensity. Five alternative flexibility scenarios are introduced and compared, with the objective of determining the achievable flexibility from a system-wide portfolio of data centres, focusing on concerns relating to server temperatures. On average, a 6.5% load reduction is seen to be achievable for a winter day during periods of high CO
2
intensity.
更多查看译文
关键词
Artificial intelligence,climate change,CO ${}_2$ intensity,data analysis,data centre,demand response,machine learning,renewable energy
AI 理解论文
溯源树
样例
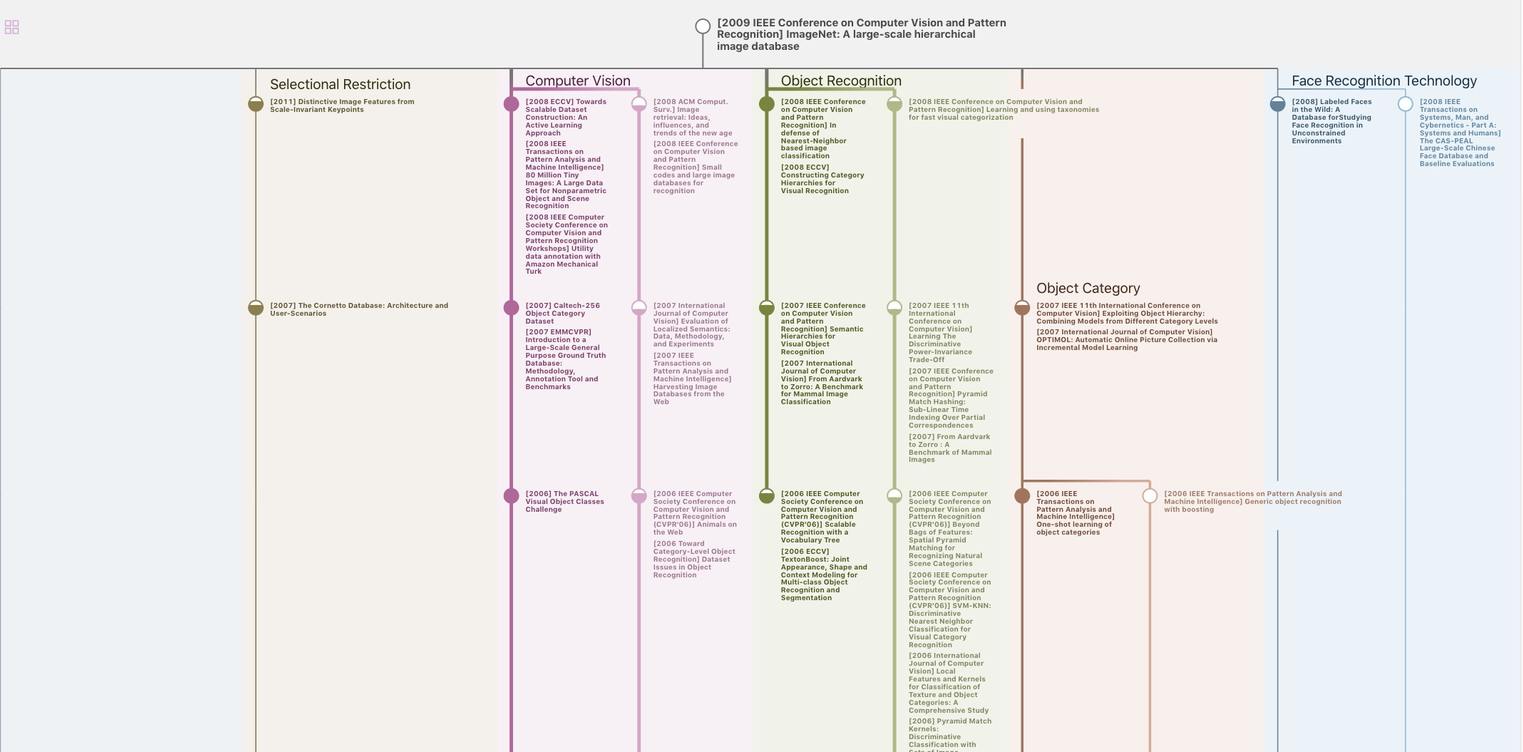
生成溯源树,研究论文发展脉络
Chat Paper
正在生成论文摘要