Semi-supervised Detection, Identification and Segmentation for Abdominal Organs
Fast and Low-Resource Semi-supervised Abdominal Organ Segmentation Lecture Notes in Computer Science(2022)
摘要
Abdominal organ segmentation is an important prerequisite in many medical image analysis applications. Methods based on U-Net have demonstrated their scalability and achieved great success in different organ segmentation tasks. However, the limited number of data and labels hinders the training process of these methods. Moreover, traditional U-Net models based on convolutional neural networks suffer from limited receptive fields. Lacking the ability to model longterm dependencies from a global perspective, these methods are prone to produce false positive predictions. In this paper, we propose a new semi-supervised learning algorithm based on the vision transformer to overcome these challenges. The overall architecture of our method consists of three stages. In the first stage, we tackle the abdomen region location problem via a lightweight segmentation network. In the second stage, we adopt a vision transformer model equipped with a semisupervised learning strategy to detect different abdominal organs. In the final stage, we attach multiple organ-specific segmentation networks to automatically segment organs from their bounding boxes. We evaluate our method on MICCAI FLARE 2022 challenge dataset. Experimental results demonstrate the effectiveness of our method. Our segmentation results currently achieve 0.897 mean DSC on the leaderboard of FLARE 2022 validation set.
更多查看译文
关键词
Semi-supervised, Organ detection, Organ segmentation
AI 理解论文
溯源树
样例
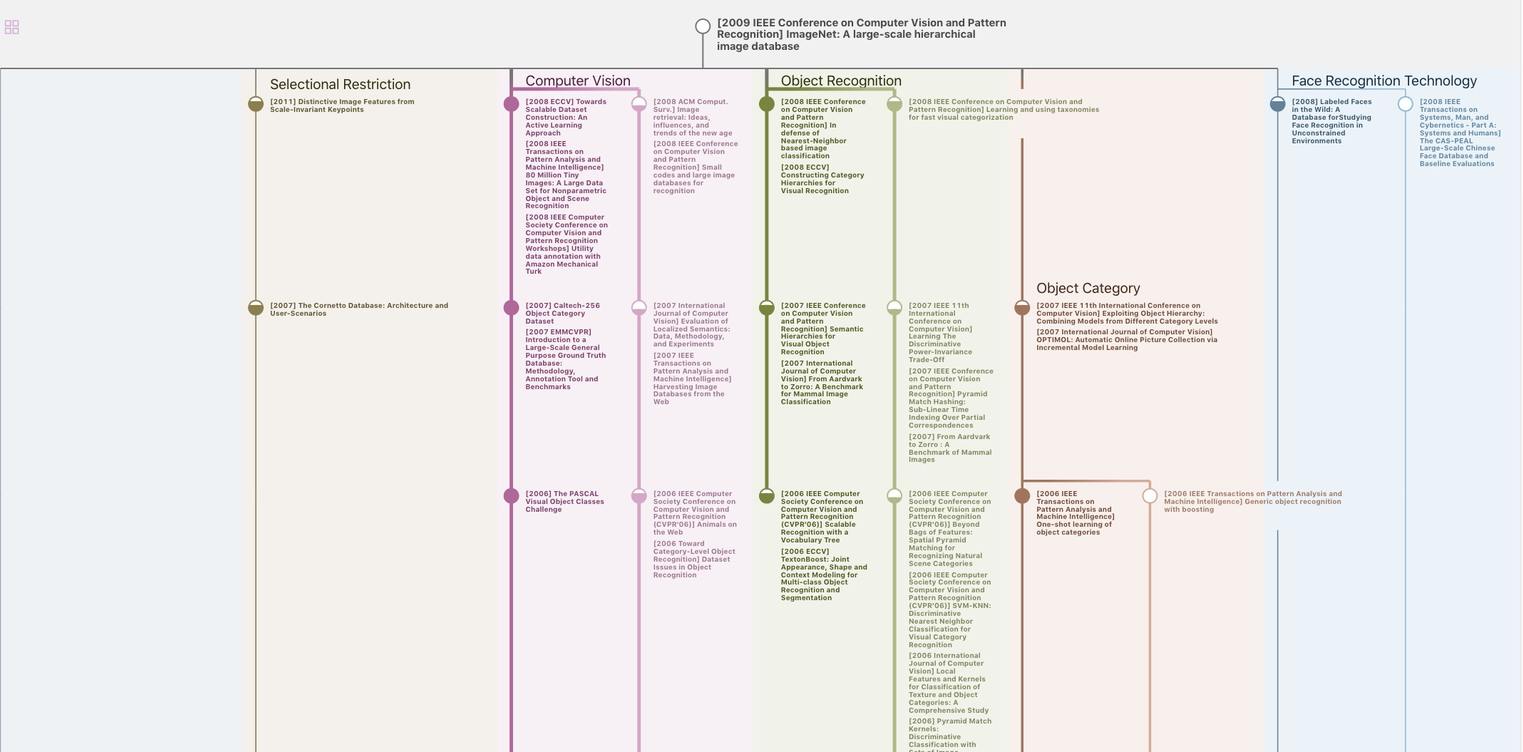
生成溯源树,研究论文发展脉络
Chat Paper
正在生成论文摘要