Data-driven Model Reduction Via Operator Inference for Coupled Aeroelastic Flutter
AIAA SCITECH 2023 Forum(2023)
摘要
This paper presents a data-driven, physics-informed model reduction technique applied to two large-scale applications: parametric nonlinear aerodynamics and coupled aeroelastic flutter. We first develop a reduced-order model (ROM) parameterized by Mach number for the compressible Navier-Stokes equations solved via a computational fluid dynamics code. We use the non-intrusive operator inference scientific machine learning method as our model reduction technique. We then extend this technique to the coupled aeroelastic case by incorporating a modal decomposition model obtained from a finite element analysis code. We use NASA's FUN3D software to run the high-fidelity simulations. We demonstrate the parametric ROM for nonlinear subsonic fluid flow over the VAT aircraft and the coupled ROM for flutter of the AGARD wing. We show that compared to the high-fidelity FUN3D simulations, parametric operator inference speeds up computation time by approximately three orders of magnitude, and coupled operator inference speeds up flutter computation time by approximately two orders of magnitude.
更多查看译文
AI 理解论文
溯源树
样例
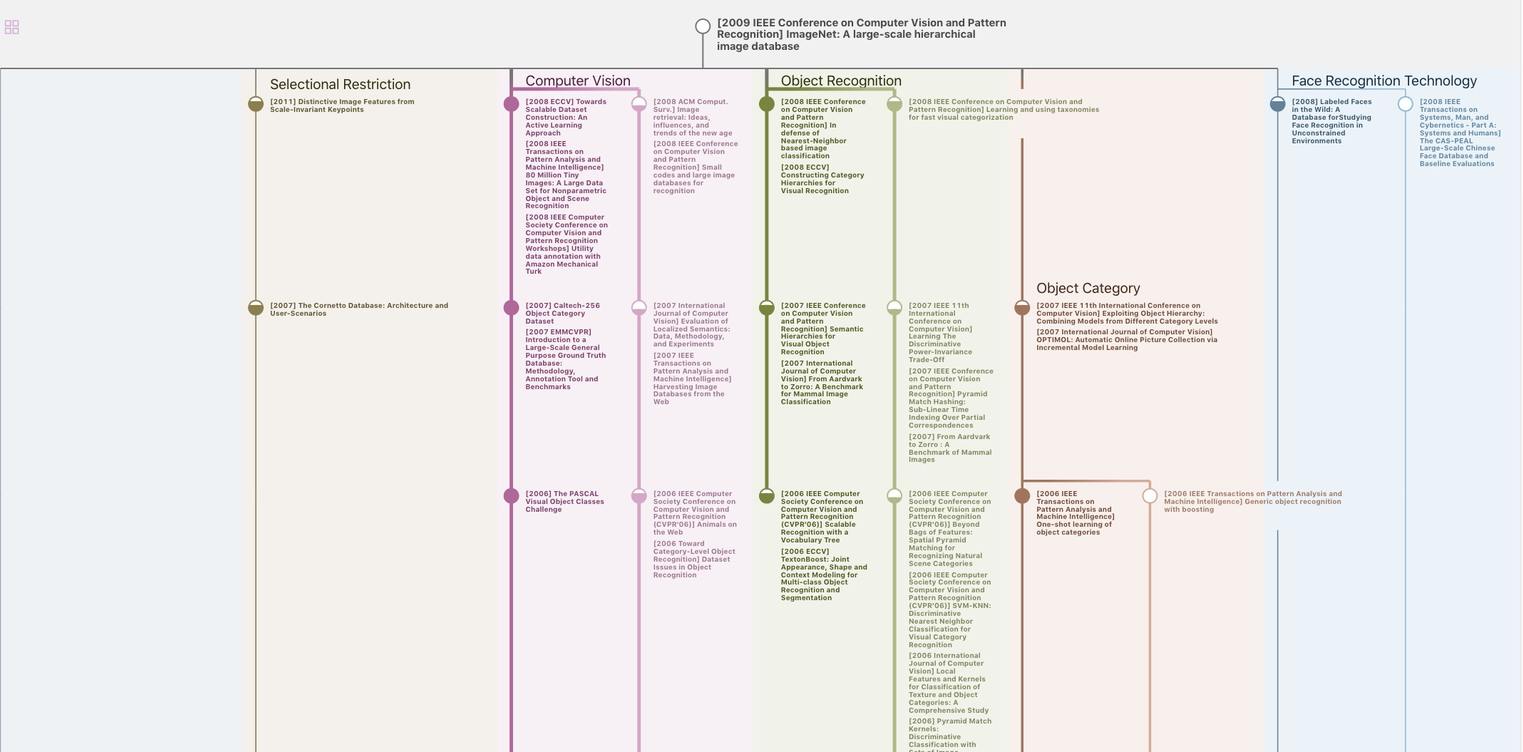
生成溯源树,研究论文发展脉络
Chat Paper
正在生成论文摘要