A Practice-Oriented Benchmark Strategy to Predict the UV-Vis Spectra of Organic Photocatalysts
Chemistry–Methods(2023)
摘要
With this work, we wish to facilitate further developments in photocatalysis by proposing reliable methods for the computational pre-screening of potential photocatalysts. To this end, we have developed a new benchmark strategy, and we have applied it to evaluate the predictions given by two wavefunction and several density functional theory (DFT) methods for the UV-vis absorption spectra of recently developed organic photocatalyst molecules. The novelty in our benchmark framework is that it focuses on evaluating the real-world applicability of computational methods and does not penalize errors that do not contribute to spectral shapes. We employ a spectral fitting process where the calculated excitations are convoluted with Gaussians using two parameters for broadening and wavelength scaling. This way, most methods can sufficiently reproduce the experimental spectra, but they differ in how much adjustment they require from the parameters. Overall, the double hybrids (with the notable exception of DSD-BLYP) are the best functionals that offer the highest predictive power as they require practically no scaling. They are exceptionally good in estimating the excitation energies with almost 90% of the fitted spectra falling into the +/- 10% scaling window. This is the same level of accuracy as provided by the STEOM-DLPNO-CCSD correlated wavefunction method. In terms of cost efficiency, M06 emerges as the best functional. It compensates a slightly less consistent performance with lower computational demand and availability in nearly all computational codes. Therefore, we recommend the use of double-hybrid and M06 functionals for UV-vis spectrum prediction of novel organic photocatalysts, and we also highlight that M06 can be used as a black-box method even by those who are non-experts in computational chemistry. The developed protocol and a user-friendly notebook to assist the analysis are available on GitHub.
更多查看译文
关键词
uv‐vis
AI 理解论文
溯源树
样例
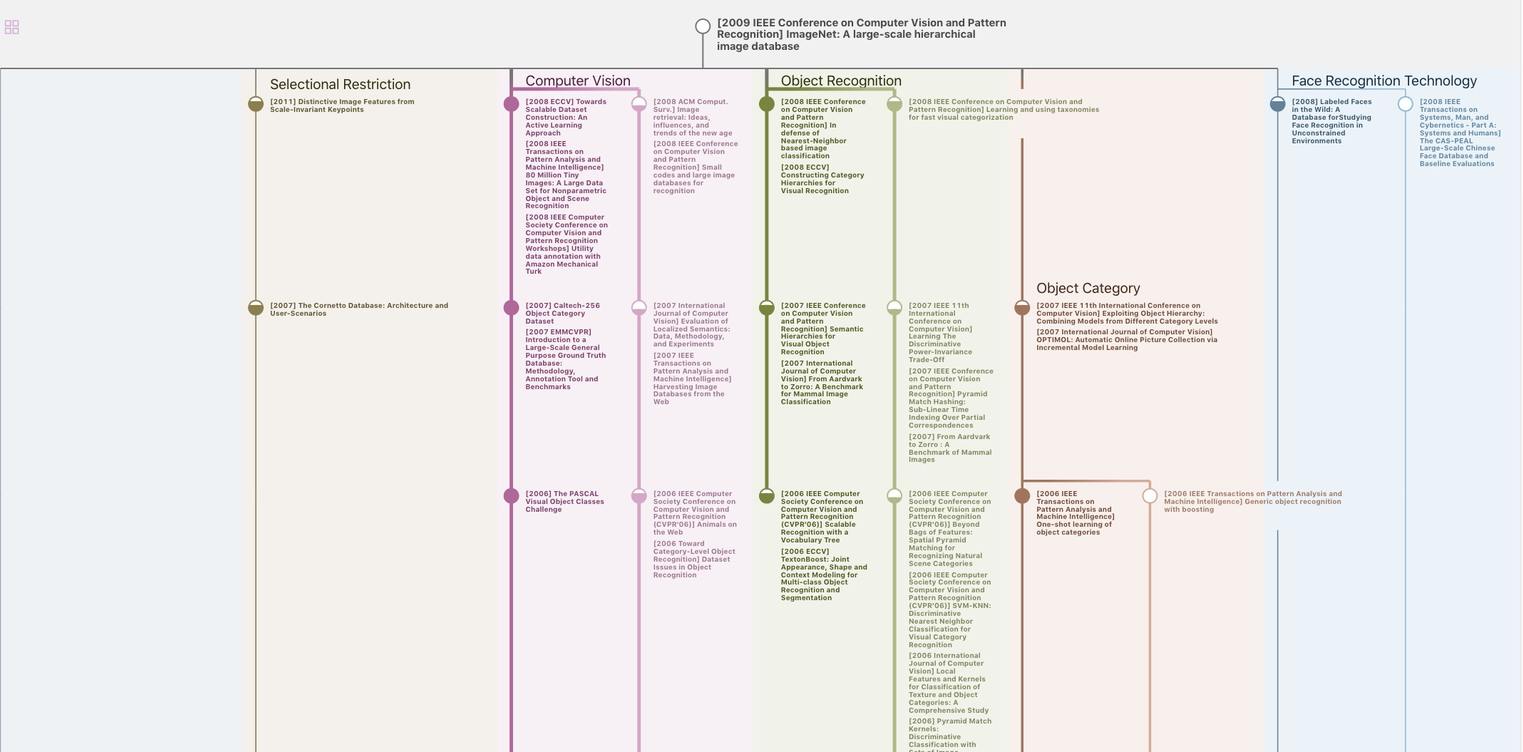
生成溯源树,研究论文发展脉络
Chat Paper
正在生成论文摘要