Rapidly predicting the effect of tool geometry on the wrinkling of biaxial NCFs during composites manufacturing using a deep learning surrogate model
Composites Part B: Engineering(2023)
摘要
A deep learning surrogate model is developed to rapidly predict the wrinkling patterns of a biaxial non-crimp fabric (NCF) layup for any given tool geometry during forming. The underlying dataset of finite element simulations is used to investigate the effect of tool geometry on wrinkling severity. The trained surrogate model is able to make reliable predictions of wrinkling patterns at a very low computational cost, suitable for tool design optimisation. Results indicate that certain geometrical features have a greater impact on wrinkling than others. In particular, forming NCFs over geometries with greater draft angles tends to result in smaller wrinkles.
更多查看译文
关键词
Fabrics/textiles [A],Defects [B],Computational modelling [C],Forming [E]
AI 理解论文
溯源树
样例
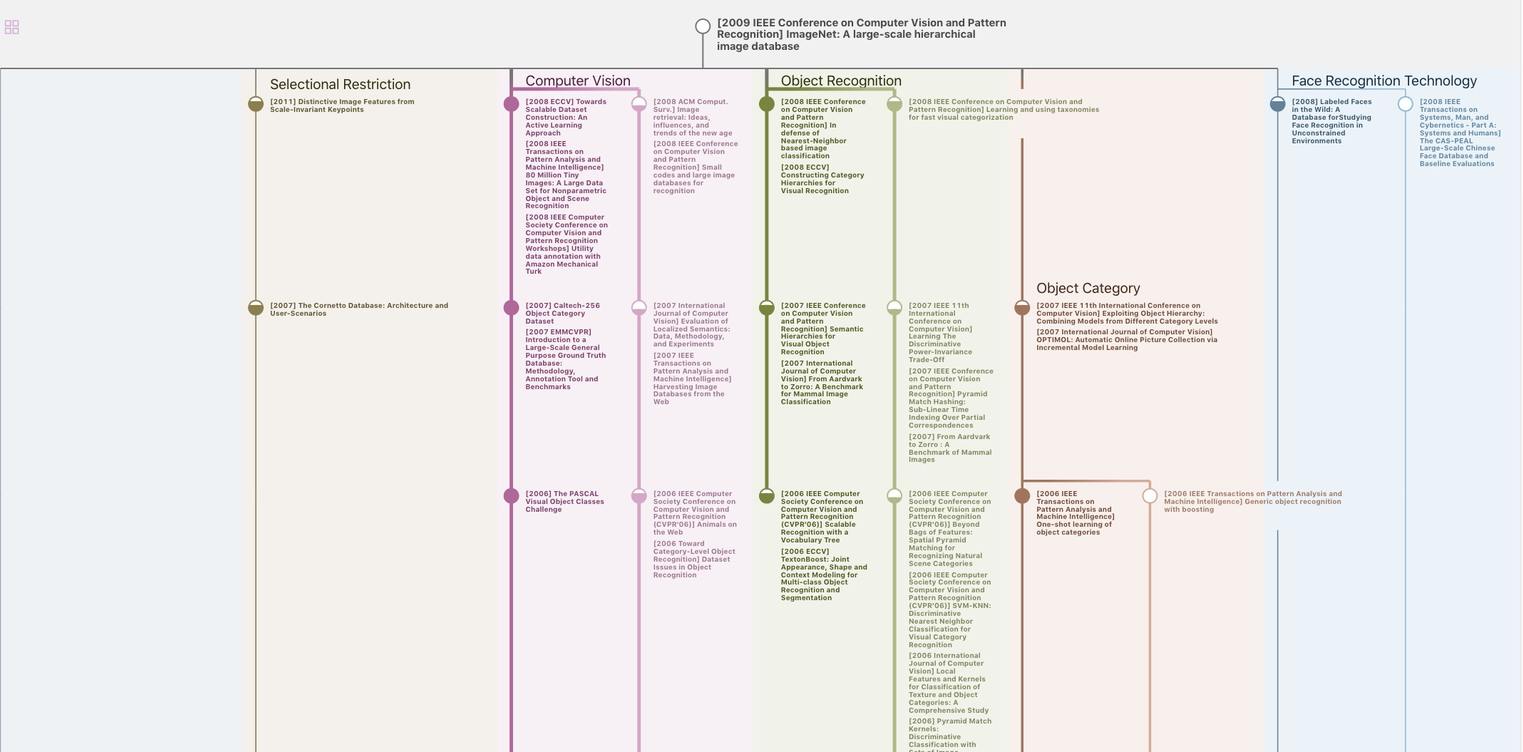
生成溯源树,研究论文发展脉络
Chat Paper
正在生成论文摘要