Subjective Probability Correction for Uncertainty Representations.
Proceedings of the 2023 CHI Conference on Human Factors in Computing Systems(2023)
摘要
We propose a new approach to uncertainty communication: we keep the uncertainty representation fixed, but adjust the distribution displayed to compensate for biases in people’s subjective probability in decision-making. To do so, we adopt a linear-in-probit model of subjective probability and derive two corrections to a Normal distribution based on the model’s intercept and slope: one correcting all right-tailed probabilities, and the other preserving the mode and one focal probability. We then conduct two experiments on U.S. demographically-representative samples. We show participants hypothetical U.S. Senate election forecasts as text or a histogram and elicit their subjective probabilities using a betting task. The first experiment estimates the linear-in-probit intercepts and slopes, and confirms the biases in participants’ subjective probabilities. The second, preregistered follow-up shows participants the bias-corrected forecast distributions. We find the corrections substantially improve participants’ decision quality by reducing the integrated absolute error of their subjective probabilities compared to the true probabilities. These corrections can be generalized to any univariate probability or confidence distribution, giving them broad applicability. Our preprint, code, data, and preregistration are available at https://doi.org/10.17605/osf.io/kcwxm
更多查看译文
关键词
uncertainty visualization,subjective probability,perception,election forecasts
AI 理解论文
溯源树
样例
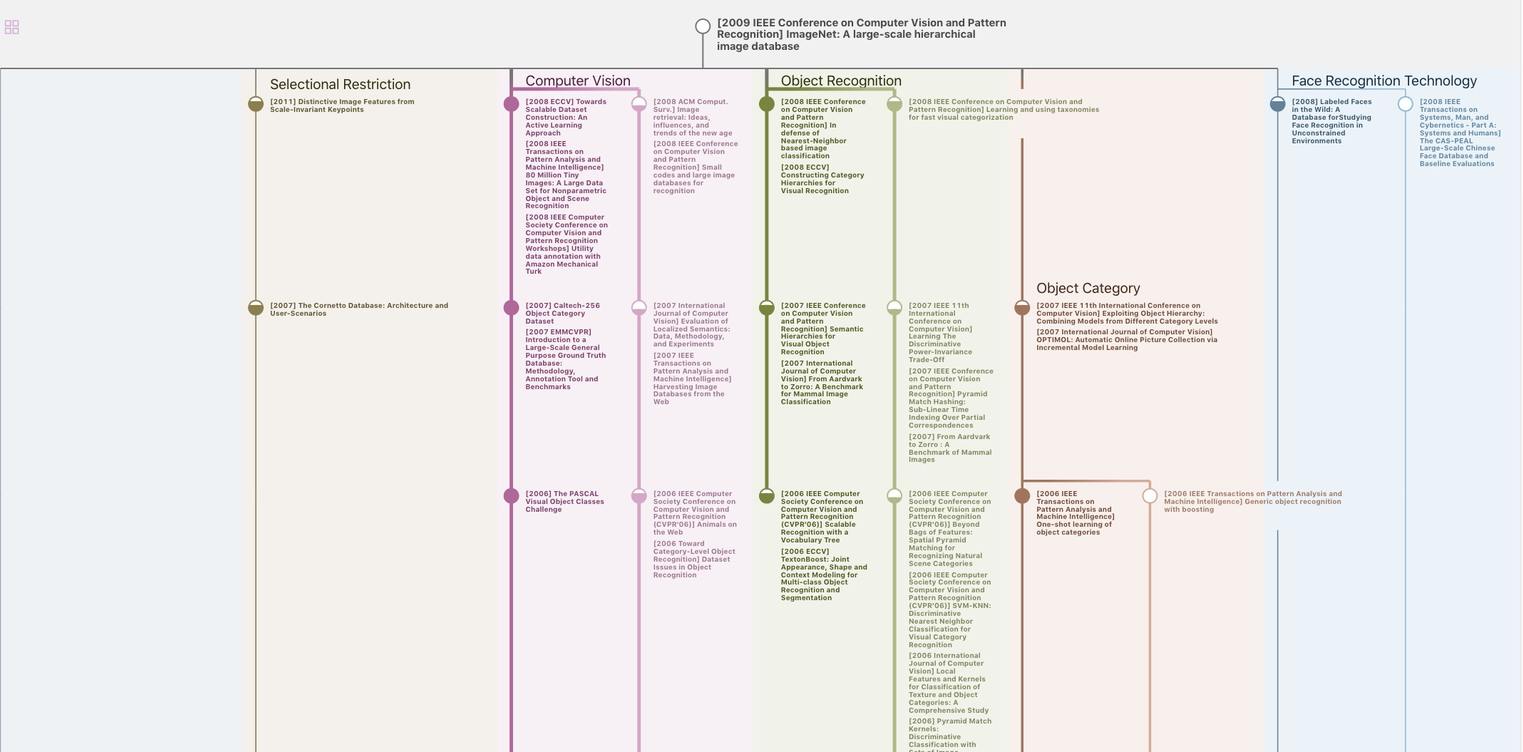
生成溯源树,研究论文发展脉络
Chat Paper
正在生成论文摘要